Kernel Evolving Participatory Fuzzy Modeling for Time Series Forecasting: New Perspectives Based on Distance Measures
2022 IEEE INTERNATIONAL CONFERENCE ON FUZZY SYSTEMS (FUZZ-IEEE)(2022)
摘要
The evolving Fuzzy Systems (eFSs) has demonstrated a powerful class of model for time series forecasting, due to their autonomy to handle with the data, and with highly complex problems in real-world applications. Unfortunately, real-world data contains sources of perturbations and variations that can be correlated to external variables, known as randomness. An alternative cause of randomness is chaos, forming the chaotic time series. This paper suggests the ePL-KRLS-FSM, a new class of evolving fuzzy modeling approach that combines participatory learning (PL), a kernel recursive least squares method (KRLS), and data transformation into fuzzy sets (FSs). This transformation allows better handling of inaccuracies with the data, proposing a model that can predict chaotic data with more exactness. The model is evaluated using nonlinear dynamic system and the main stock index of the TAIEX. Furthermore, the performance of the ePL-KRLS-FSM is compared with some related state-of-the-art rule-based eFSs and traditional forecasting models. The computational results show that the proposed model is competitive and performs more consistently than the compared models.
更多查看译文
关键词
Time series forecasting, evolving Fuzzy Systems, Chaotic time series
AI 理解论文
溯源树
样例
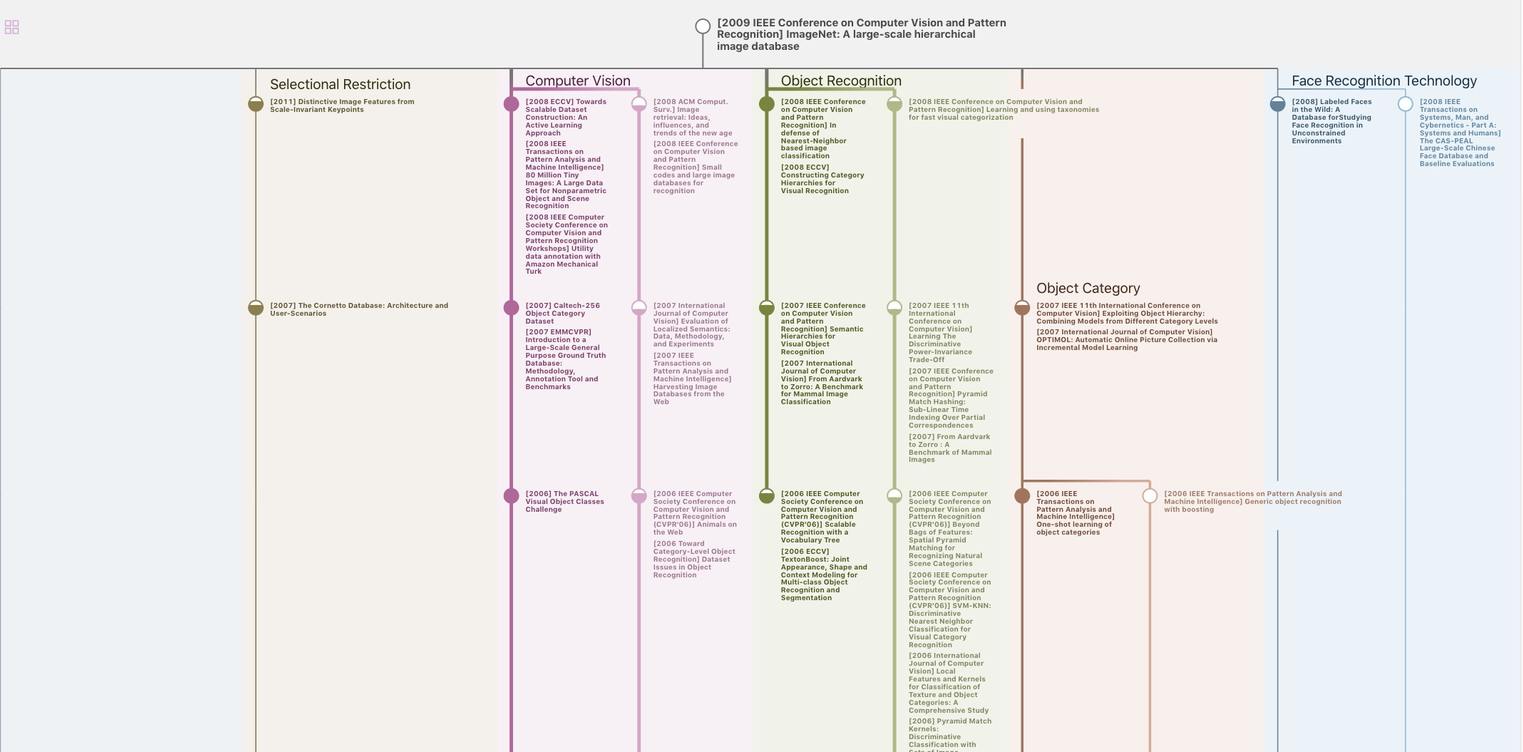
生成溯源树,研究论文发展脉络
Chat Paper
正在生成论文摘要