Abstractive Summarization of Broadcast News Stories for Estonian
BALTIC JOURNAL OF MODERN COMPUTING(2022)
摘要
We present an approach for generating abstractive summaries for Estonian spoken news stories in a low-resource setting. Given a recording of a radio news story, the goal is to create a summary that captures the essential information in a short format. The approach consists of two steps: automatically generating the transcript and applying a state-of-the-art text summarization system to generate the result. We evaluated a number of models, with the best-performing model leveraging the large English BART model pre-trained on CNN/DailyMail dataset and fine-tuned on machine-translated in-domain data, and with the test data translated to English and back. The method achieved a ROUGE-1 score of 17.22, improving on the alternatives and achieving the best result in human evaluation. The applicability of the proposed solution might be limited in languages where machine translation systems are not mature. In such cases multilingual BART should be considered, which achieved a ROUGE-1 score of 17.00 overall and a score of 16.22 without machine translation based data augmentation.
更多查看译文
关键词
Abstractive summarization, low-resource languages, pre-trained models, multilingual models, machine-translation
AI 理解论文
溯源树
样例
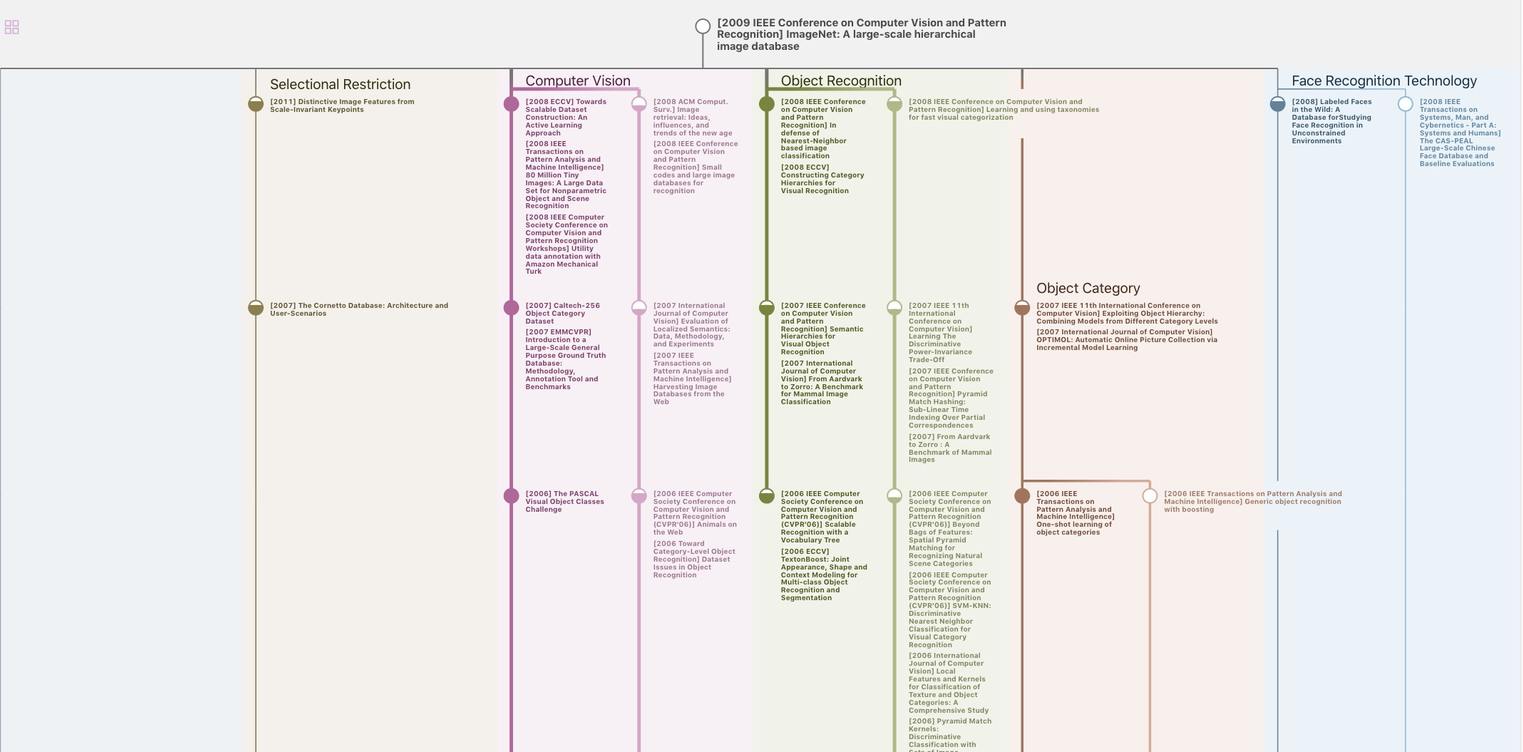
生成溯源树,研究论文发展脉络
Chat Paper
正在生成论文摘要