Optimized Deep Ensemble Technique for Malicious Behavior Classification in Cloud
CYBERNETICS AND SYSTEMS(2023)
摘要
We must find novel approaches to improve intrusion detection systems in cloud computing as network traffic increases and attacks become more pervasive and complex. This study offers Ensemble methods for Cloud-based Network Intrusion Detection and Classification. The main means through which Ensemble Learning enhances each Machine Learning result Using algorithms and a strong classifier. In starting, the redundant data is removed from the collected raw data and the data cleaning is performed as the pre-processing phase. From these pre-processed data, modified holoentropy, correntropy, information gain, and statistical features are extracted. An ensemble technique that uses models like the neural network (NN), support vector machine (SVM), random forest (RF), and optimized deep belief network (DBN) performs the final detection of the presence or absence of attacks. The process of fine-tuning is supervised, and in order to increase the training's accuracy, the weights received from the RBM pre-training are modified. A new hybrid model called Moth Logarithmic Assisted Deer Hunting Optimization performs this fine-tuning (MLADHO). The conceptual fusion of the traditional Moth Flame Optimization (MFO) and the Deer Hunting Optimization Algorithm (DHO) yields the suggested algorithm. Finally, both the algorithmic and classifier evaluation is performed by comparing the suggested over conventional methods.
更多查看译文
关键词
Cloud computing, intrusion detection, modified holoentropy, correntropy, information gain, deer updated moth flame optimization (DUMFO)
AI 理解论文
溯源树
样例
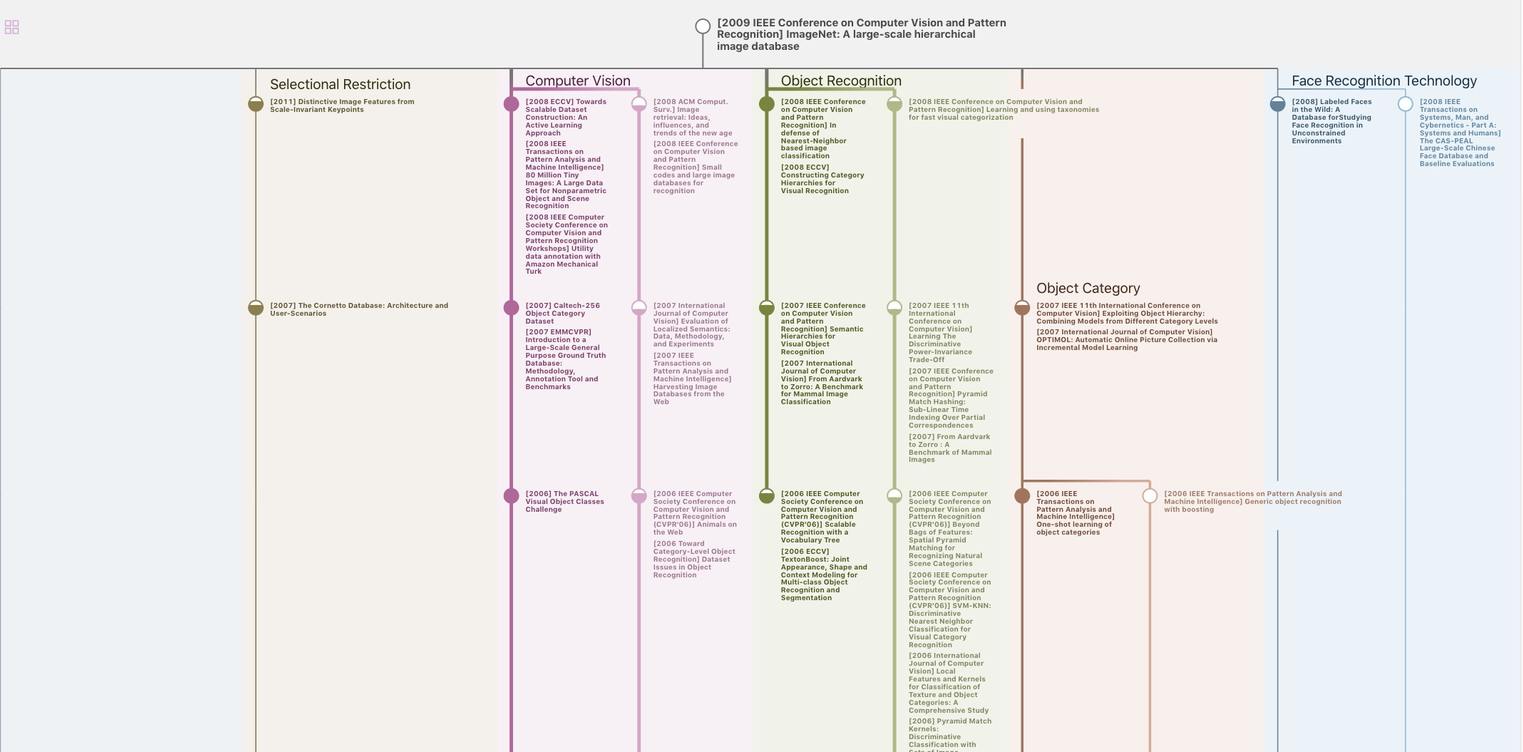
生成溯源树,研究论文发展脉络
Chat Paper
正在生成论文摘要