Optimizing Accelerator Configurability for Mobile Transformer Networks
2022 IEEE INTERNATIONAL CONFERENCE ON ARTIFICIAL INTELLIGENCE CIRCUITS AND SYSTEMS (AICAS 2022) INTELLIGENT TECHNOLOGY IN THE POST-PANDEMIC ERA(2022)
摘要
Transformers are increasingly used to process time series data. Their deployment in mobile devices is however challenging due to their large computational requirements. Hardware acceleration through custom neural network processors can reduce the resulting latency and energy footprint of the network. Yet, the large variety of different layer types in mobile transformers troubles the selection of the best accelerator architecture and hardware mapping. Specifically, the layer performance strongly depends on the spatial unrolling dimensions, i.e. the layer loop dimensions along which hardware parallelization is enabled. This paper will therefore research the best datapath organization, and required datapath flexibility to efficiently support mobile transformers. The MobileViT-S network is selected as the reference network for its wide variety in layer types. Results are explored across a wide range of accelerator area (datapath dimensions, memory size) and bandwidth constraints.
更多查看译文
关键词
CNN,transformer,cross-layer,configurability,hardware modelling and optimization
AI 理解论文
溯源树
样例
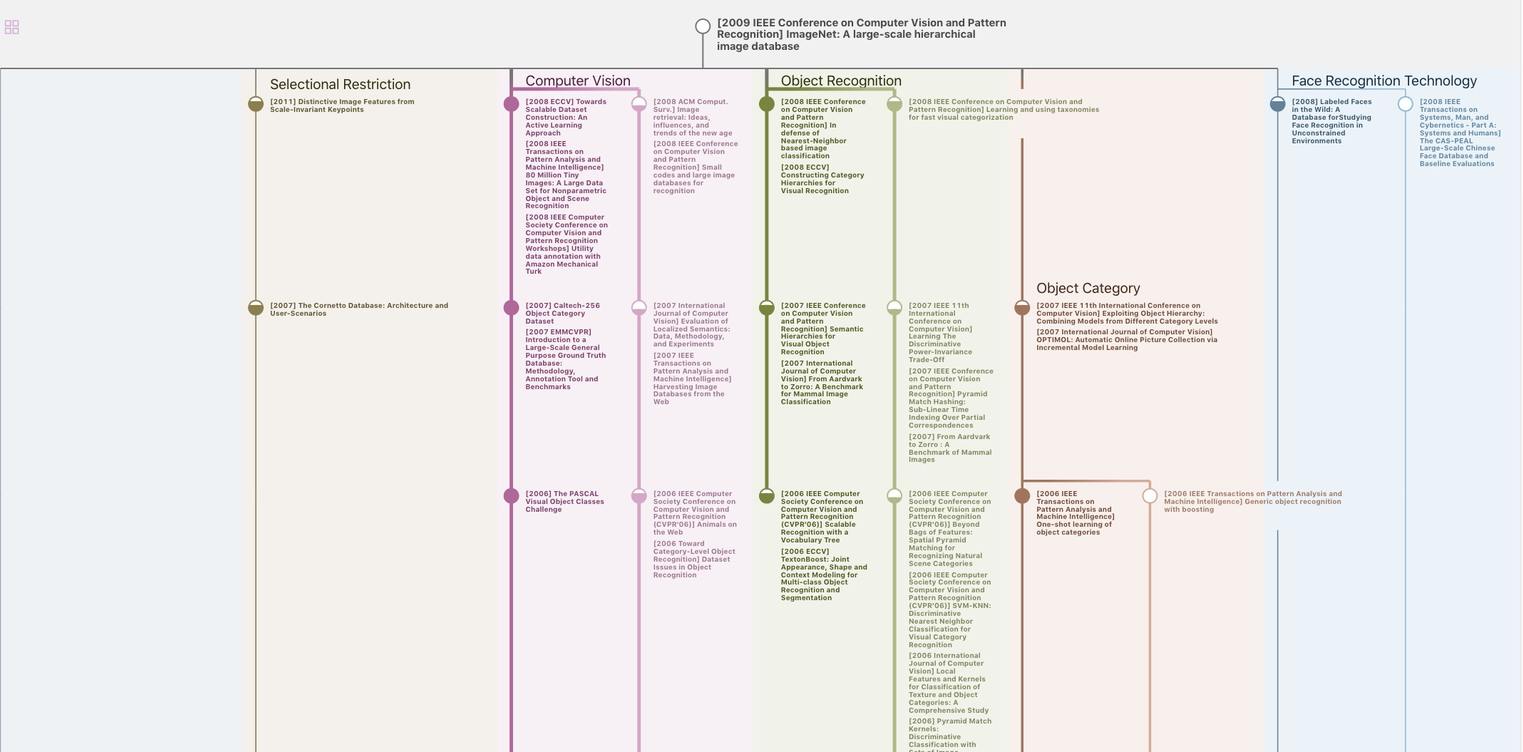
生成溯源树,研究论文发展脉络
Chat Paper
正在生成论文摘要