MOGNET: A Mux-residual quantized Network leveraging Online-Generated weights
2022 IEEE 4th International Conference on Artificial Intelligence Circuits and Systems (AICAS)(2022)
摘要
This paper presents a compact model architecture called MOGNET, compatible with a resource-limited hardware. MOGNET uses a streamlined Convolutional factorization block based on a combination of 2 point-wise (1×1) convolutions with a group-wise convolution in-between. To further limit the overall model size and reduce the on-chip required memory, the second point-wise convolution's parameters are on-line generated by a Cellular Automaton structure. In addition, MOGNET enables the use of low-precision weights and activations, by taking advantage of a Multiplexer mechanism with a proper Bitshift rescaling for integrating residual paths without increasing the hardware- related complexity. To efficiently train this model we also introduce a novel weight ternarization method favoring the balance between quantized levels. Experimental results show that given tiny memory budget (sub-2Mb), MOGNET can achieve higher accuracy with a clear gap up to 1% at a similar or even lower model size compared to recent state-of-the-art methods.
更多查看译文
关键词
CNN,quantized neural networks,skip connections,channel attention,logic-gated CNN,Cellular Automaton
AI 理解论文
溯源树
样例
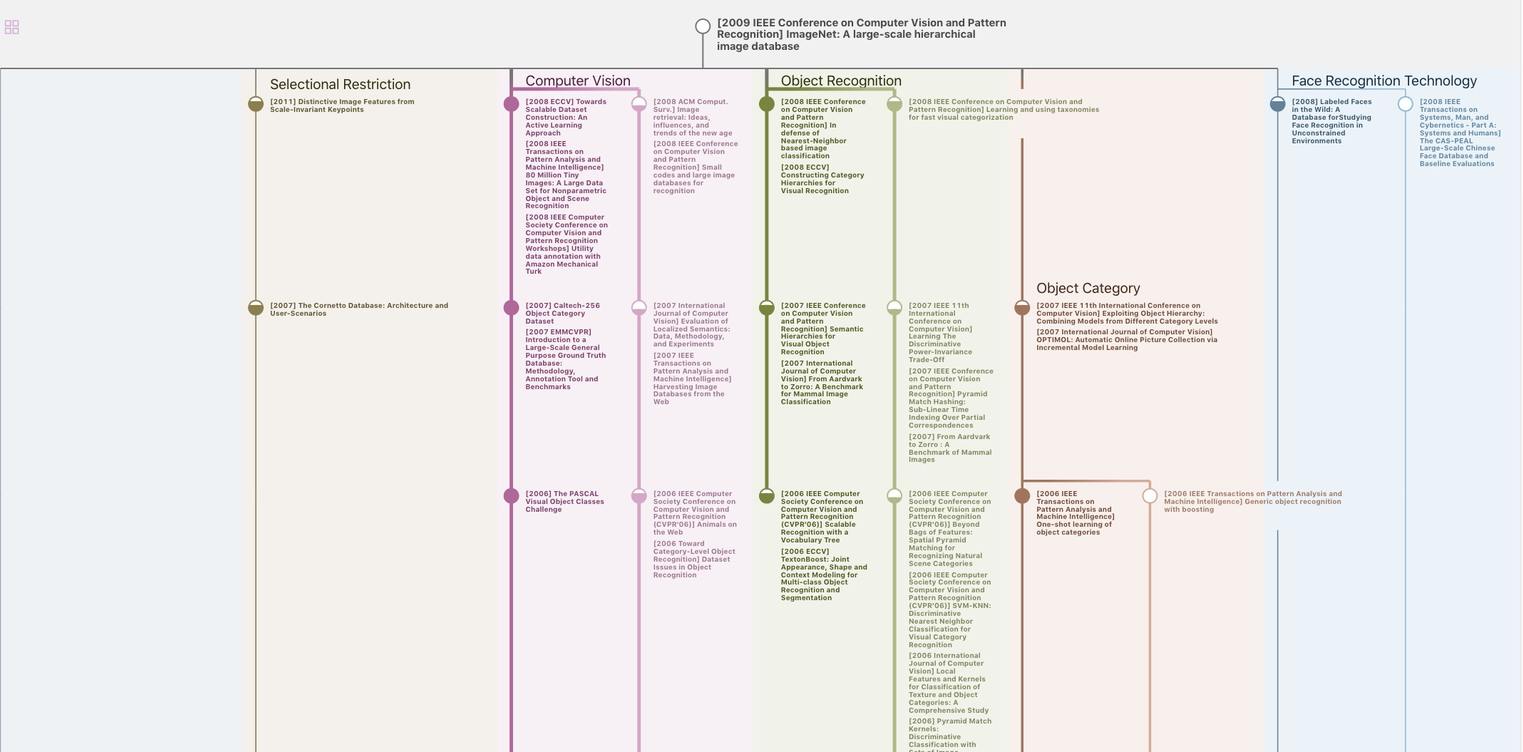
生成溯源树,研究论文发展脉络
Chat Paper
正在生成论文摘要