UASSR: Unsupervised Arbitrary Scale Super-Resolution Reconstruction of Single Anisotropic 3D Images via Disentangled Representation Learning
MEDICAL IMAGE COMPUTING AND COMPUTER ASSISTED INTERVENTION, MICCAI 2022, PT VI(2022)
摘要
Deep learning-based single image super resolution (SISR) algorithms have great potential to recover high-resolution (HR) images from low-resolution (LR) inputs. However, most studies require paired LR and HR images for a supervised training, which are difficult to organize in clinical applications. In this paper, we propose an unsupervised arbitrary scale super-resolution reconstruction (UASSR) method based on disentangled representation learning, eliminating the requirement of paired images for training. Applying our method to applications of generating HR images with high inter-plane resolution from LR images with low inter-plane resolution. At the inference stage, we design a strategy to fuse multiple reconstructed HR images from different views to achieve better super-resolution (SR) result. We conduct experiments on one publicly available dataset including 507 MR volumes of the knee joint and an in-house dataset containing 130 CT volumes of the lower spine. Results from our comprehensive experiments demonstrate superior performance of UASSR over other state-of-the-art methods. A reference implementation of our method can be found at: https://github.com/jialewang1/UASSR.
更多查看译文
关键词
Unsupervised image super-resolution, Arbitrary scale, Disentangled representation learning, Magnetic resonance imaging, Computed tomography
AI 理解论文
溯源树
样例
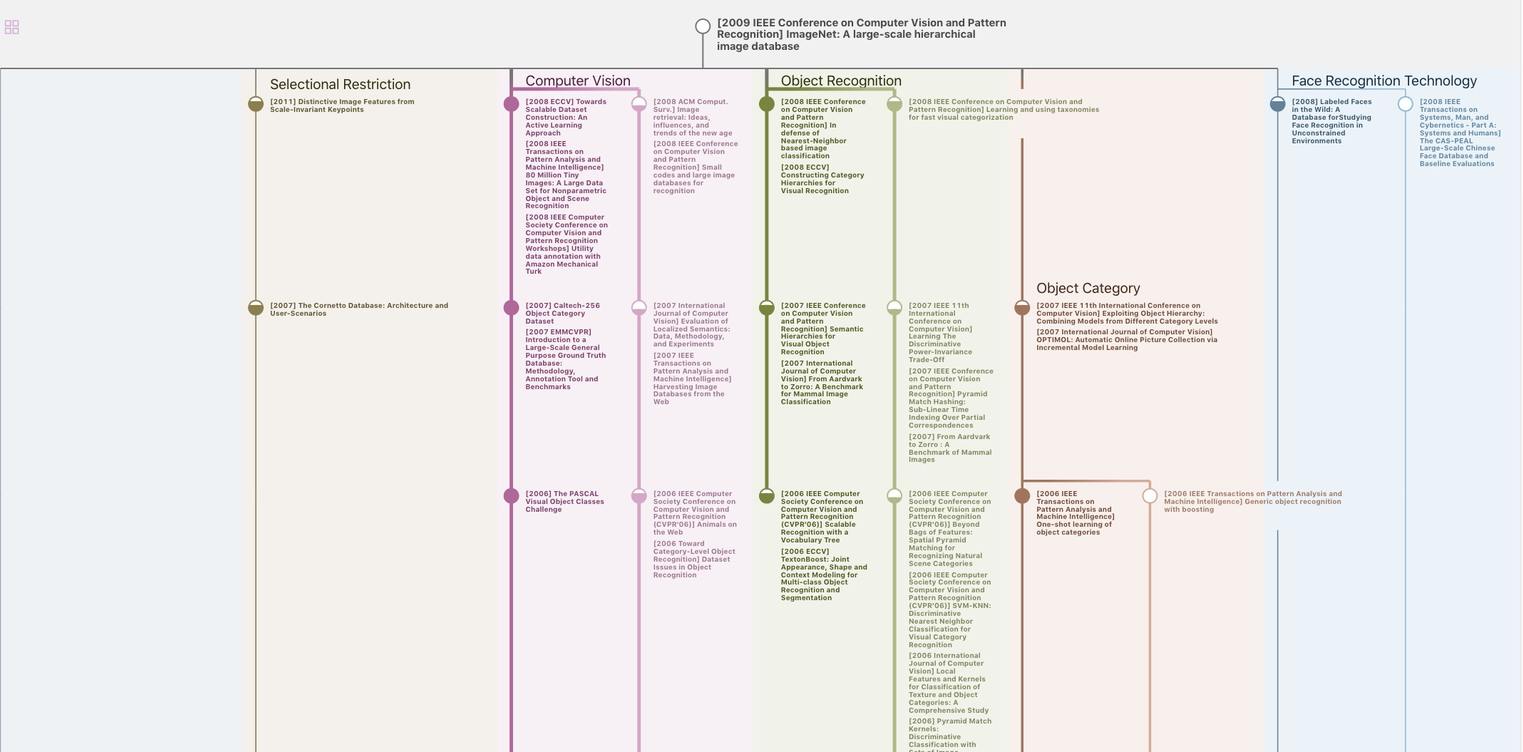
生成溯源树,研究论文发展脉络
Chat Paper
正在生成论文摘要