A Transformer-Based Iterative Reconstruction Model for Sparse-View CT Reconstruction
MEDICAL IMAGE COMPUTING AND COMPUTER ASSISTED INTERVENTION, MICCAI 2022, PT VI(2022)
摘要
Sparse-view computed tomography (CT) is one of the primary means to reduce the radiation risk. But the reconstruction of sparse-view CT will be contaminated by severe artifacts. By carefully designing the regularization terms, the iterative reconstruction (IR) algorithm can achieve promising results. With the introduction of deep learning techniques, learned regularization terms with convolution neural network (CNN) attracts much attention and can further improve the performance. In this paper, we propose a learned local-nonlocal regularization-based model called RegFormer to reconstruct CT images. Specifically, we unroll the iterative scheme into a neural network and replace handcrafted regularization terms with learnable kernels. The convolution layers are used to learn local regularization with excellent denoising performance. Simultaneously, transformer encoders and decoders incorporate the learned nonlocal prior into the model, preserving the structures and details. To improve the ability to extract deep features during iteration, we introduce an iteration transmission (IT) module, which can further promote the efficiency of each iteration. The experimental results show that our proposed RegFormer achieves competitive performance in artifact reduction and detail preservation compared to some state-of-the-art sparse-view CT reconstruction methods.
更多查看译文
关键词
Computed tomography, Image reconstruction, Deep learning, Nonlocal regularization, Transformer
AI 理解论文
溯源树
样例
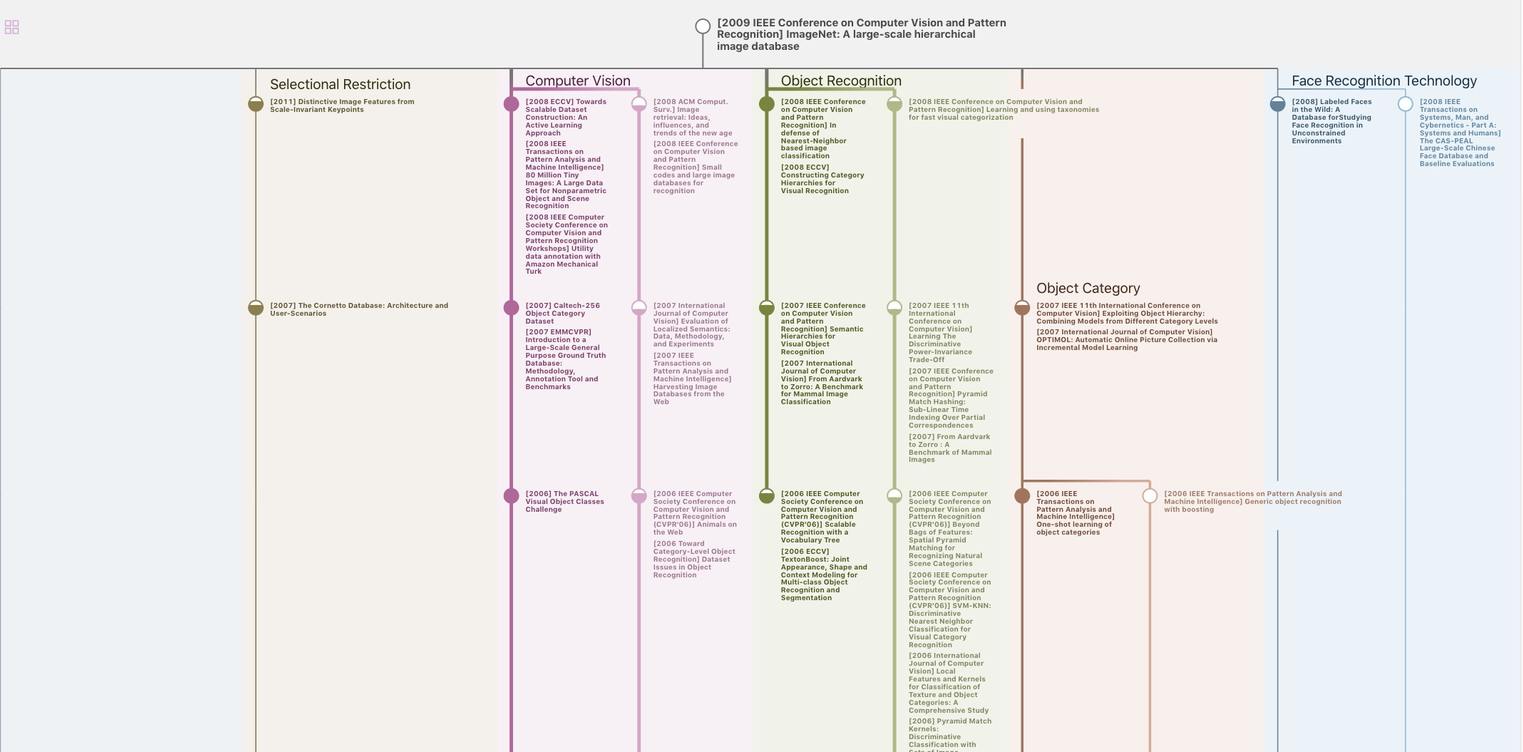
生成溯源树,研究论文发展脉络
Chat Paper
正在生成论文摘要