Unsupervised Deep Non-rigid Alignment by Low-Rank Loss and Multi-input Attention
MEDICAL IMAGE COMPUTING AND COMPUTER ASSISTED INTERVENTION, MICCAI 2022, PT VI(2022)
摘要
We propose a deep low-rank alignment network that can simultaneously perform non-rigid alignment and noise decomposition for multiple images despite severe noise and sparse corruptions. To address this challenging task, we introduce a low-rank loss in deep learning under the assumption that a set of well-aligned, well-denoised images should be linearly correlated, and thus, that a matrix consisting of the images should be low-rank. This allows us to remove the noise and corruption from input images in a self-supervised learning manner (i.e., without requiring supervised data). In addition, we introduce multi-input attention modules into Siamese U-nets in order to aggregate the corruption information from the set of images. To the best of our knowledge, this is the first attempt to introduce a low-rank loss for deep learning-based non-rigid alignment. Experiments using both synthetic data and real medical image data demonstrate the effectiveness of the proposed method. The code will be publicly available in https://github.com/asanomitakanori/Unsupervised-Deep-Non-Rigid-Alignment-by-Low-Rank-Loss-and-Multi-Input-Attention.
更多查看译文
AI 理解论文
溯源树
样例
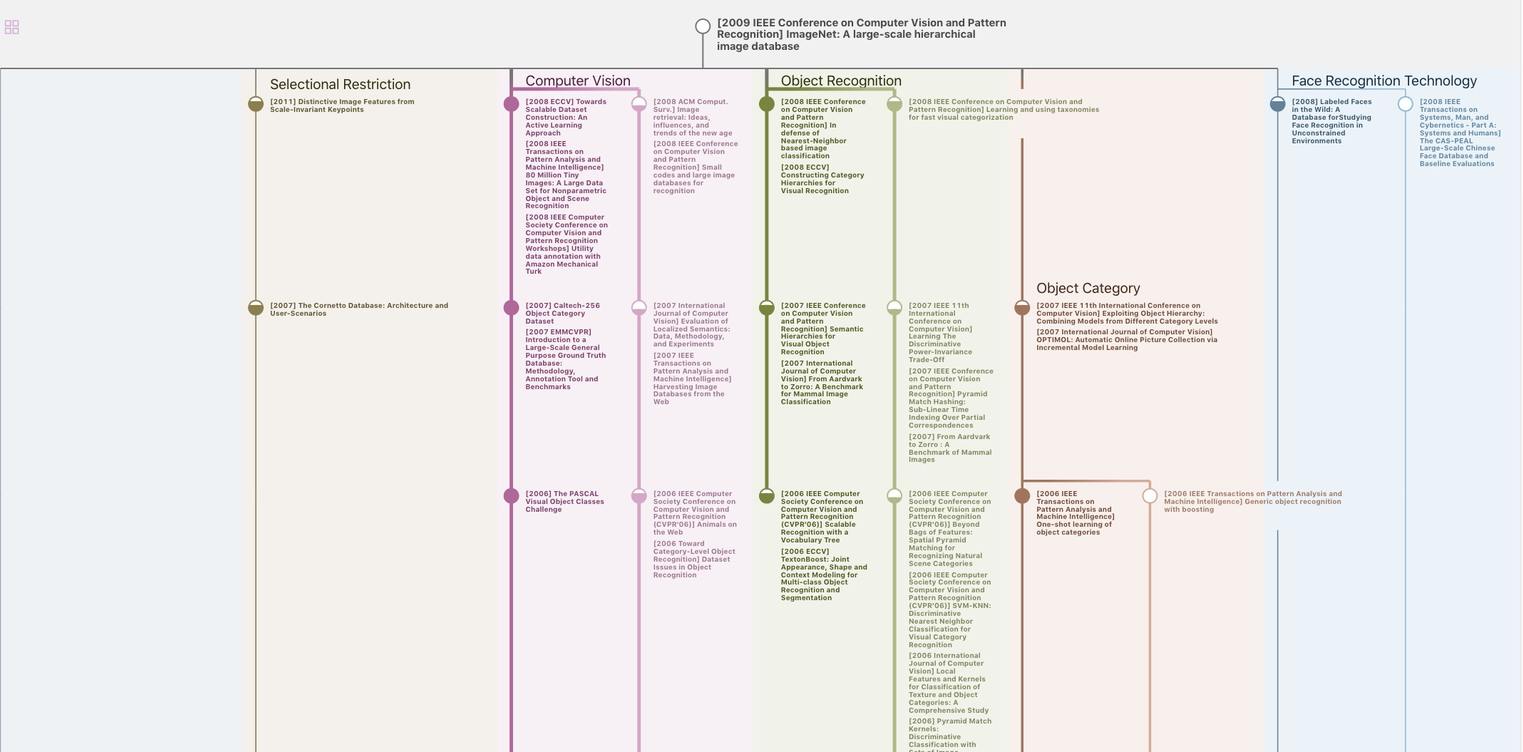
生成溯源树,研究论文发展脉络
Chat Paper
正在生成论文摘要