Learning with Context Encoding for Single-Stage Cranial Bone Labeling and Landmark Localization
MEDICAL IMAGE COMPUTING AND COMPUTER ASSISTED INTERVENTION, MICCAI 2022, PT VIII(2022)
摘要
Automatic anatomical segmentation and landmark localization in medical images are important tasks during craniofacial analysis. While deep neural networks have been recently applied to segment cranial bones and identify cranial landmarks from computed tomography (CT) or magnetic resonance (MR) images, existing methods often provide suboptimal and sometimes unrealistic results because they do not incorporate contextual image information. Additionally, most state-of-the-art deep learning methods for cranial bone segmentation and landmark detection rely on multi-stage data processing pipelines, which are inefficient and prone to errors. In this paper, we propose a novel context encoding-constrained neural network for single-stage cranial bone labeling and landmark localization. Specifically, we design and incorporate a novel context encoding module into a U-Net-like architecture. We explicitly enforce the network to capture context-related features for representation learning so pixel-wise predictions are not isolated from the image context. In addition, we introduce a new auxiliary task to model the relative spatial configuration of different anatomical landmarks, which serves as an additional regularization that further refines network predictions. The proposed method is end-to-end trainable for single-stage cranial bone labeling and landmark localization. The method was evaluated on a highly diverse pediatric 3D CT image dataset with 274 subjects. Our experiments demonstrate superior performance of our method compared to state-of-the-art approaches.
更多查看译文
关键词
Cranial segmentation, Landmark localization, Context encoding
AI 理解论文
溯源树
样例
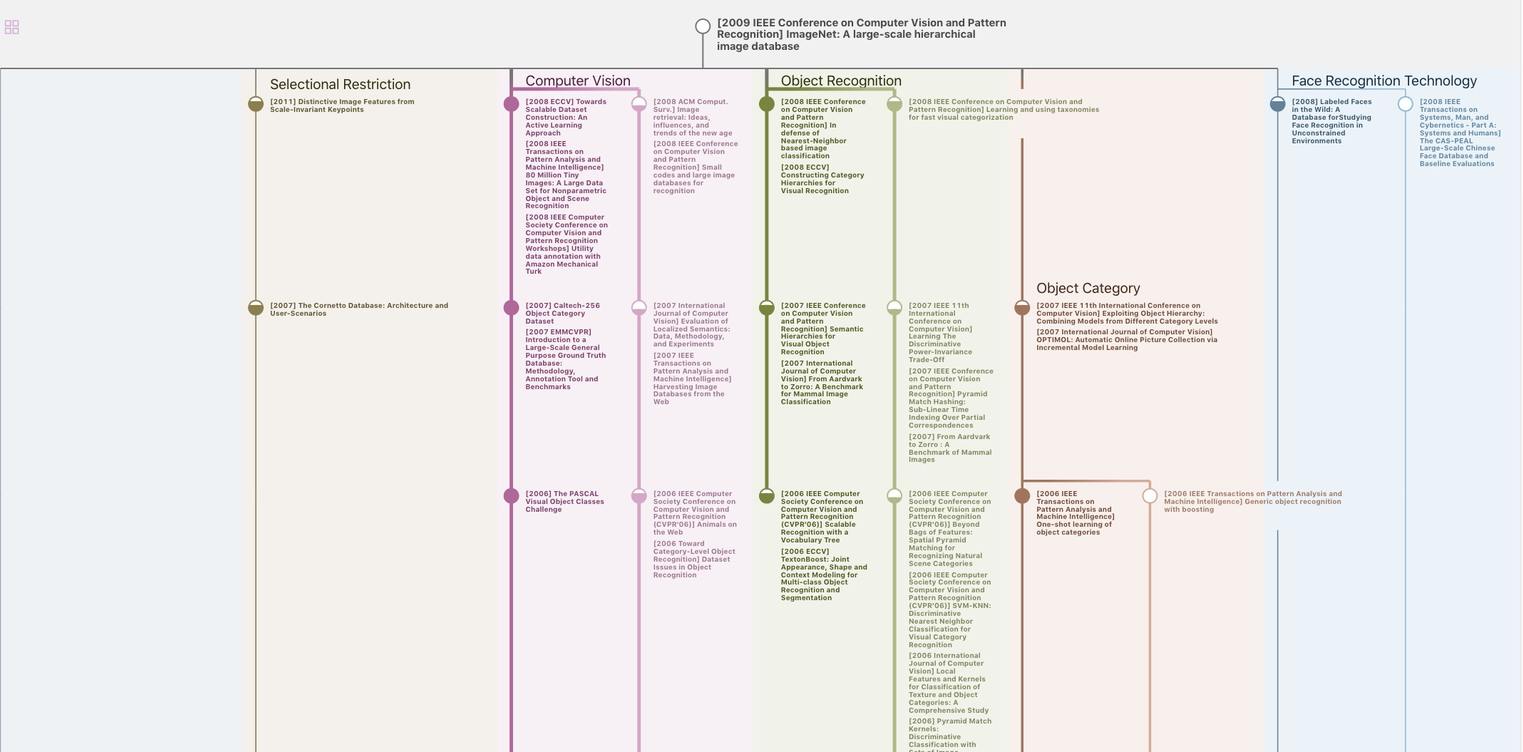
生成溯源树,研究论文发展脉络
Chat Paper
正在生成论文摘要