SELF-SUPERVISED ADVERSARIAL SHAPE COMPLETION
XXIV ISPRS CONGRESS IMAGING TODAY, FORESEEING TOMORROW, COMMISSION II(2022)
摘要
The goal of this paper is 3D shape completion: given an incomplete instance of a known category, hallucinate a complete version of it that is geometrically plausible. We develop an adversarial framework that makes it possible to learn shape completion in a self-supervised fashion, only from incomplete examples. This is enabled by a discriminator network that rejects incomplete shapes, via a loss function that separately assesses local sub-regions of the generated example and accepts only regions with sufficiently high point count. This inductive bias against empty regions forces the generator to output complete shapes. We demonstrate the effectiveness of this approach on synthetic data from ShapeNet and ModelNet, and on a real mobile mapping dataset with nearly 9'000 incomplete cars. Moreover, we apply it to the KITTI autonomous driving dataset without retraining, to highlight its ability to generalise to different data characteristics.
更多查看译文
关键词
3D Point Clouds, Adversarial Learning, Unsupervised Learning, Shape Completion
AI 理解论文
溯源树
样例
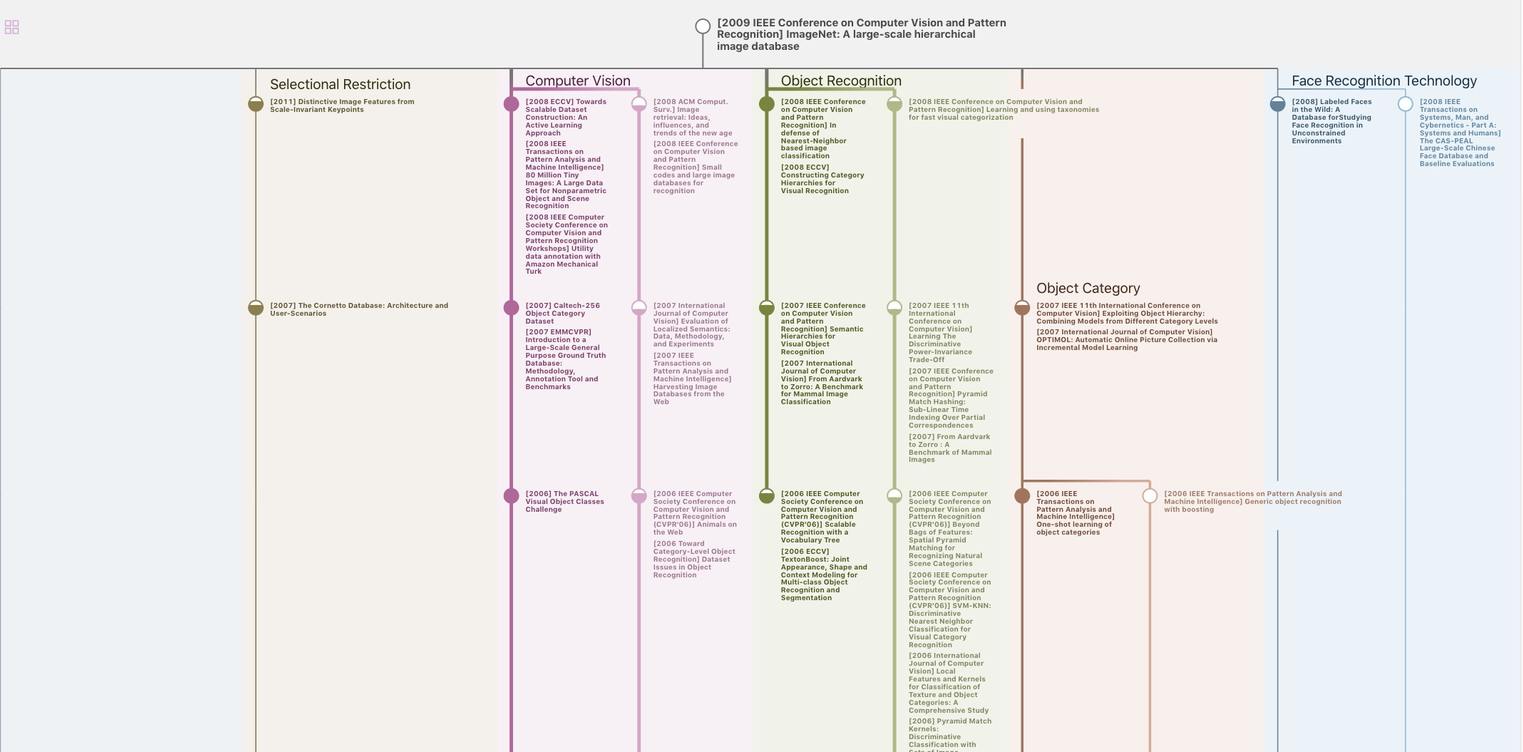
生成溯源树,研究论文发展脉络
Chat Paper
正在生成论文摘要