An enhanced driver’s risk perception modeling based on gate recurrent unit network
2022 IEEE INTELLIGENT VEHICLES SYMPOSIUM (IV)(2022)
摘要
Risk perception is one of the most important driving skills for drivers to detect potential traffic accident and make correct risk avoidance behaviors. Accurate model and evaluation of risk perception can effectively identify driver’s perception deficiencies and serve as an important human factor for the design of advance driver assistance systems. Most traditional perception ability assessing methods are based on macroscopic statistical results and lack effective mathematical models, thus making it difficult to evaluate risk perception quantitatively. To this end, this paper first obtains semantic understanding of traffic scenes through a semantic segmentation method based on deep learning. Then, the semantic understanding information is fused with the driver’s risk perception results to form the time series data reflecting the risk perception ability. Finally, we use gate recurrent unit (GRU) network-based learning framework to learn the time series data features and thus obtain the behavioral model which responds to the driver’s risk perception ability. By verifying the classification performance on multiple drivers, the risk perception model based on traffic scene understanding can accurately predict the actual cognitive behavior of individual driver. Besides, the proposed GRU-based method outperforms the traditional machine learning algorithm in modeling the driver’s risk perception behavior.
更多查看译文
关键词
risk perception,enhanced drivers,gate
AI 理解论文
溯源树
样例
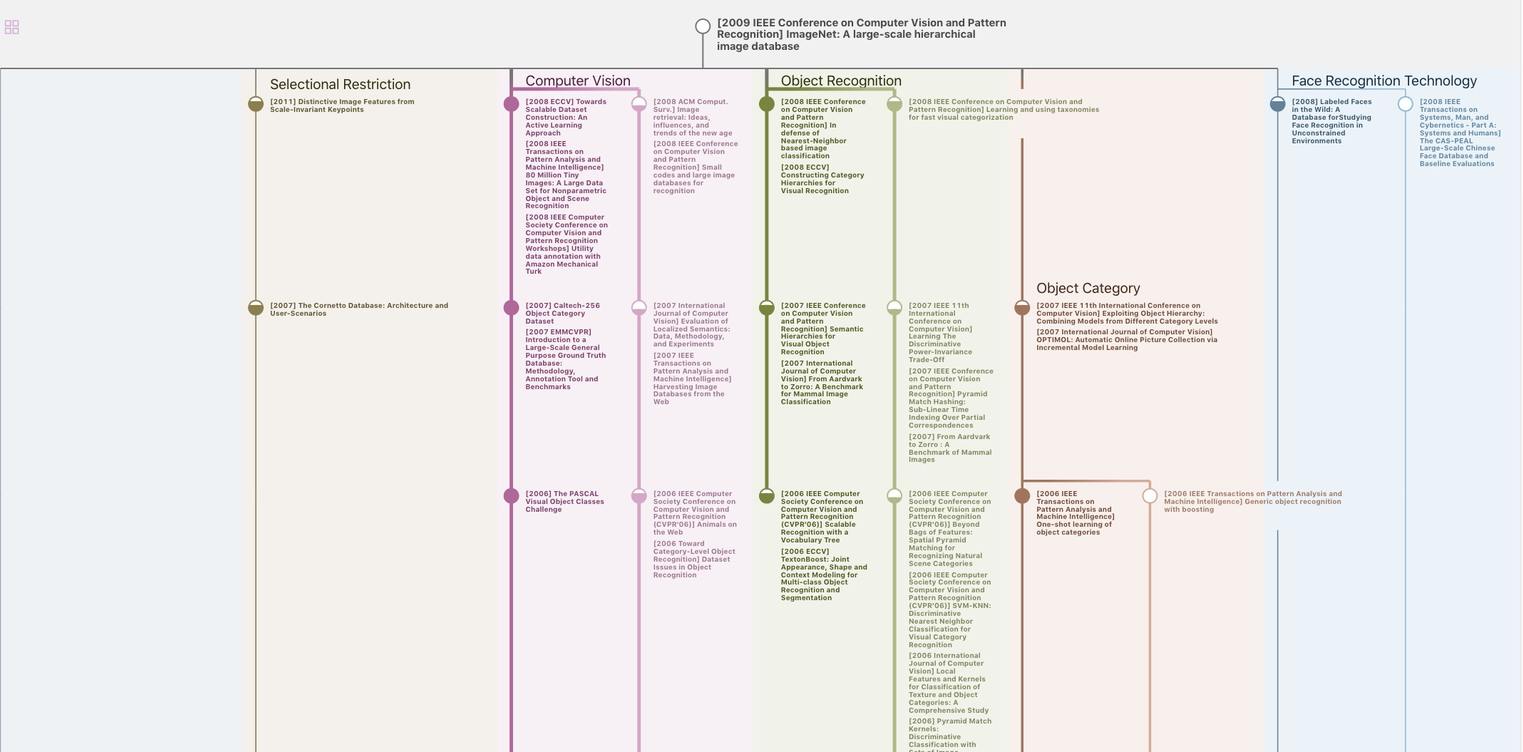
生成溯源树,研究论文发展脉络
Chat Paper
正在生成论文摘要