Multi-task Alignment Scheme for Span-level Aspect Sentiment Triplet Extraction
ARTIFICIAL NEURAL NETWORKS AND MACHINE LEARNING - ICANN 2022, PT II(2022)
摘要
Aspect Sentiment Triplet Extraction (ASTE) aims to extract aspect terms, opinion terms, and the corresponding sentiments from the target sentence, which is a universal subtask in the field of aspect-based sentiment analysis. Recent models achieve considerable performance in a joint learning manner but still suffer from significant limitations, such as the inability to capture span representations accurately and redundant triples are extracted due to ignoring syntactic dependencies. To tackle these issues, we propose a novel multi-task alignment scheme (MAS) for the ASTE task to tackle these issues. Particularly, we explore the aspect/opinion semantic composition module to obtain the aspect candidate set and the opinion candidate set at span-level. Moreover, the pointer-specific tagging strategy is designed to characterize internal associations between aspects and opinions by incorporating syntactic dependencies. Furthermore, a triplet alignment scheme is designed to generate triplets by aligning aspects and corresponding opinions with the position of specific pointers. Experimental results on four benchmark datasets demonstrate that our proposed model outperforms other representative ones in terms of Precision, Recall and Macro-Averaged F1.
更多查看译文
关键词
Span-level triplet extraction, Multi-task learning, Position alignment, Syntactic dependency, Pointer-specific tagging
AI 理解论文
溯源树
样例
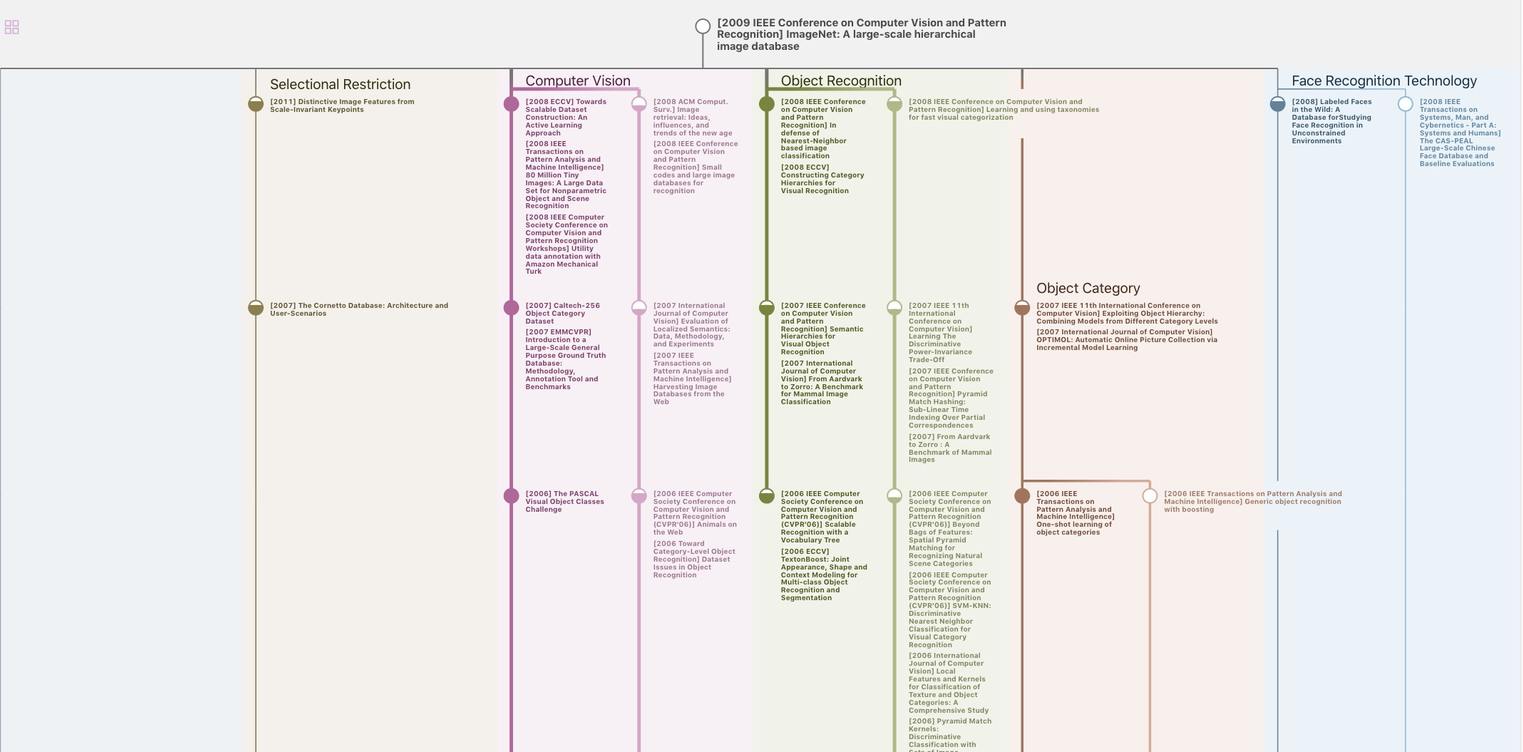
生成溯源树,研究论文发展脉络
Chat Paper
正在生成论文摘要