Leveraging Clinical BERT in Multimodal Mortality Prediction Models for COVID-19
2022 IEEE 35th International Symposium on Computer-Based Medical Systems (CBMS)(2022)
摘要
Clinical prediction models are often based solely on the use of structured data in electronic health records, e.g. vital parameters and laboratory results, effectively ignoring potentially valuable information recorded in other modalities, such as free-text clinical notes. Here, we report on the development of a multimodal model that combines structured and unstructured data. In particular, we study how best to make use of a clinical language model in a multimodal setup for predicting 30-day all-cause mortality upon hospital admission in patients with COVID-19. We evaluate three strategies for incorporating a domain-specific clinical BERT model in multimodal prediction systems: (i) without fine-tuning, (ii) with unimodal fine-tuning, and (iii) with multimodal fine-tuning. The best-performing model leverages multimodal fine-tuning, in which the clinical BERT model is updated based also on the structured data. This multimodal mortality prediction model is shown to outperform unimodal models that are based on using either only structured data or only unstructured data. The experimental results indicate that clinical prediction models can be improved by including data in other modalities and that multimodal fine-tuning of a clinical language model is an effective strategy for incorporating information from clinical notes in multimodal prediction systems.
更多查看译文
关键词
natural language processing,machine learning,language models,clinical BERT,multimodal learning,electronic health records,mortality prediction,COVID-19
AI 理解论文
溯源树
样例
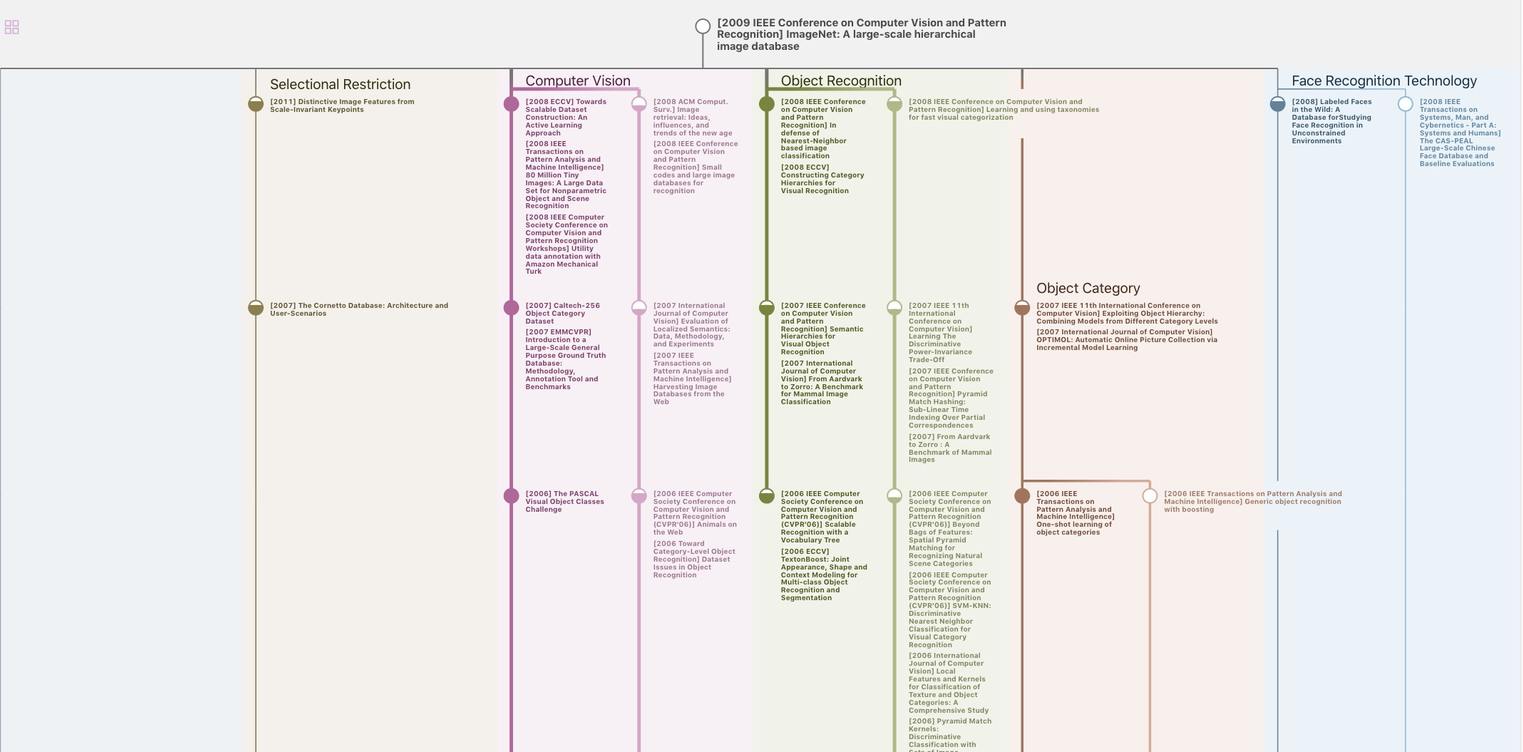
生成溯源树,研究论文发展脉络
Chat Paper
正在生成论文摘要