Flat-Aware Cross-Stage Distilled Framework for Imbalanced Medical Image Classification
MEDICAL IMAGE COMPUTING AND COMPUTER ASSISTED INTERVENTION, MICCAI 2022, PT III(2022)
摘要
Medical data often follow imbalanced distributions, which poses a long-standing challenge for computer-aided diagnosis systems built upon medical image classification. Most existing efforts are conducted by applying re-balancing methods for the collected training samples, which improves the predictive performance for the minority class but at the cost of decreasing the performance for the majority. To address this paradox, we adopt a flat-aware cross-stage distilled framework (FCD), where we first search for flat local minima of the base training objective function on the original imbalanced dataset, and then continuously finetune this classifier within the flat region on the re-balanced one. To further prevent the performance decreasing for the majority, we propose a cross-stage distillation regularizing term to promote the optimized features to remain in the common optimal subspace. Extensive experiments on two imbalanced medical image datasets demonstrate the effectiveness of our proposed framework and its generality in improving the performance of existing imbalanced methods. The code of this work will be released publicly.
更多查看译文
关键词
Imbalanced image classification, Flat-aware optimization, Cross-stage distillation
AI 理解论文
溯源树
样例
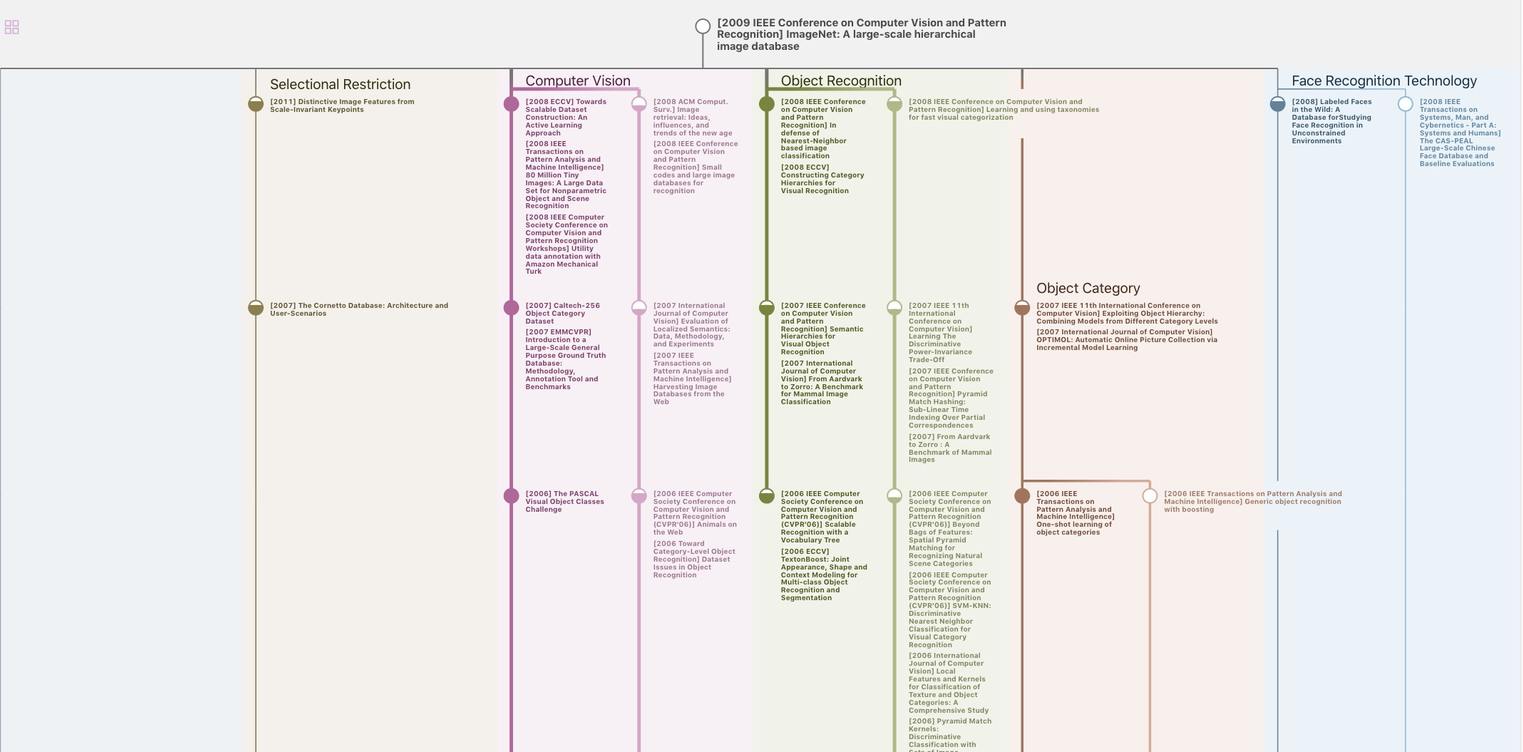
生成溯源树,研究论文发展脉络
Chat Paper
正在生成论文摘要