NerveFormer: A Cross-Sample Aggregation Network for Corneal Nerve Segmentation
MEDICAL IMAGE COMPUTING AND COMPUTER ASSISTED INTERVENTION, MICCAI 2022, PT IV(2022)
摘要
The segmentation of corneal nerves in corneal confocal microscopy (CCM) is of great to the quantification of clinical parameters in the diagnosis of eye-related diseases and systematic diseases. Existing works mainly use convolutional neural networks to improve the segmentation accuracy, while further improvement is needed to mitigate the nerve discontinuity and noise interference. In this paper, we propose a novel corneal nerve segmentation network, named NerveFormer, to resolve the above-mentioned limitations. The proposed NerveFormer includes a Deformable and External Attention Module (DEAM), which exploits the Transformer-based Deformable Attention (TDA) and External Attention (TEA) mechanisms. TDA is introduced to explore the local internal nerve features in a single CCM, while TEA is proposed to model global external nerve features across different CCM images. Specifically, to efficiently fuse the internal and external nerve features, TDA obtains the query set required by TEA, thereby strengthening the characterization ability of TEA. Therefore, the proposed model aggregates the learned features from both single-sample and cross-sample, allowing for better extraction of corneal nerve features across the whole dataset. Experimental results on two public CCM datasets show that our proposed method achieves state-of-the-art performance, especially in terms of segmentation continuity and noise discrimination.
更多查看译文
关键词
Corneal nerve segmentation, Transformer, Cross-sample
AI 理解论文
溯源树
样例
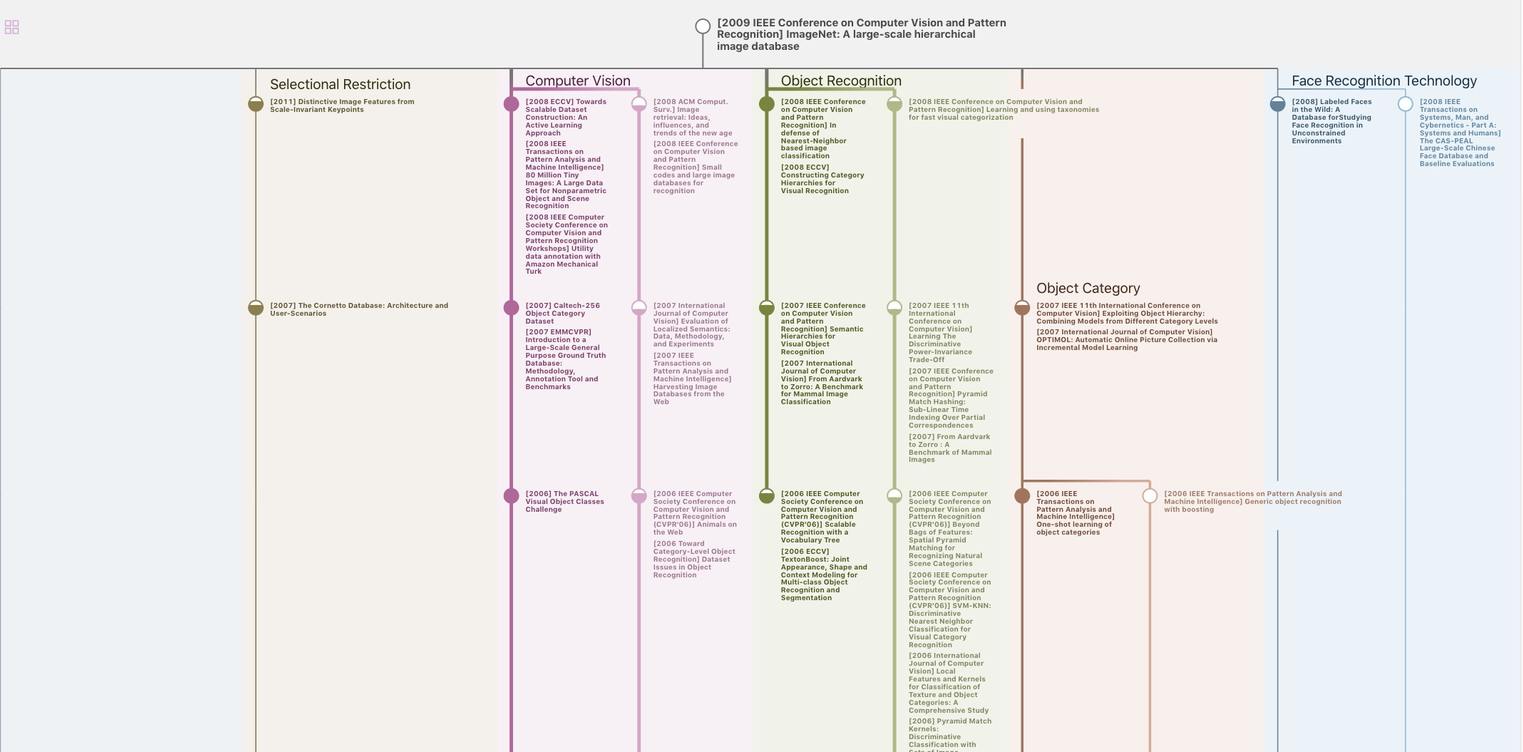
生成溯源树,研究论文发展脉络
Chat Paper
正在生成论文摘要