Mask Rearranging Data Augmentation for 3D Mitochondria Segmentation
MEDICAL IMAGE COMPUTING AND COMPUTER ASSISTED INTERVENTION, MICCAI 2022, PT IV(2022)
摘要
3D mitochondria segmentation in electron microscopy (EM) images has achieved significant progress. However, existing learning-based methods with high performance typically rely on extensive training data with high-quality manual annotations, which is time-consuming and labor-intensive. To address this challenge, we propose a novel data augmentation method tailored for 3D mitochondria segmentation. First, we train a Mask2EM network for learning the mapping from the ground-truth instance masks to real 3D EM images in an adversarial manner. Based on the Mask2EM network, we can obtain synthetic 3D EM images from arbitrary instance masks to form a sufficient amount of paired training data for segmentation. Second, we design a 3D mask layout generator to generate diverse instance layouts by rearranging volumetric instance masks according to mitochondrial distance distribution. Experiments demonstrate that, as a plug-and-play module, the proposed method boosts existing 3D mitochondria segmentation networks to achieve state-of-the-art performance. Especially, the proposed method brings significant improvements when training data is extremely limited. Code will be available at: https://github.com/qic999/MRDA_MitoSeg.
更多查看译文
关键词
Mitochondria segmentation,Data augmentation,3D convolution,Generative adversarial networks,Electron microscopy
AI 理解论文
溯源树
样例
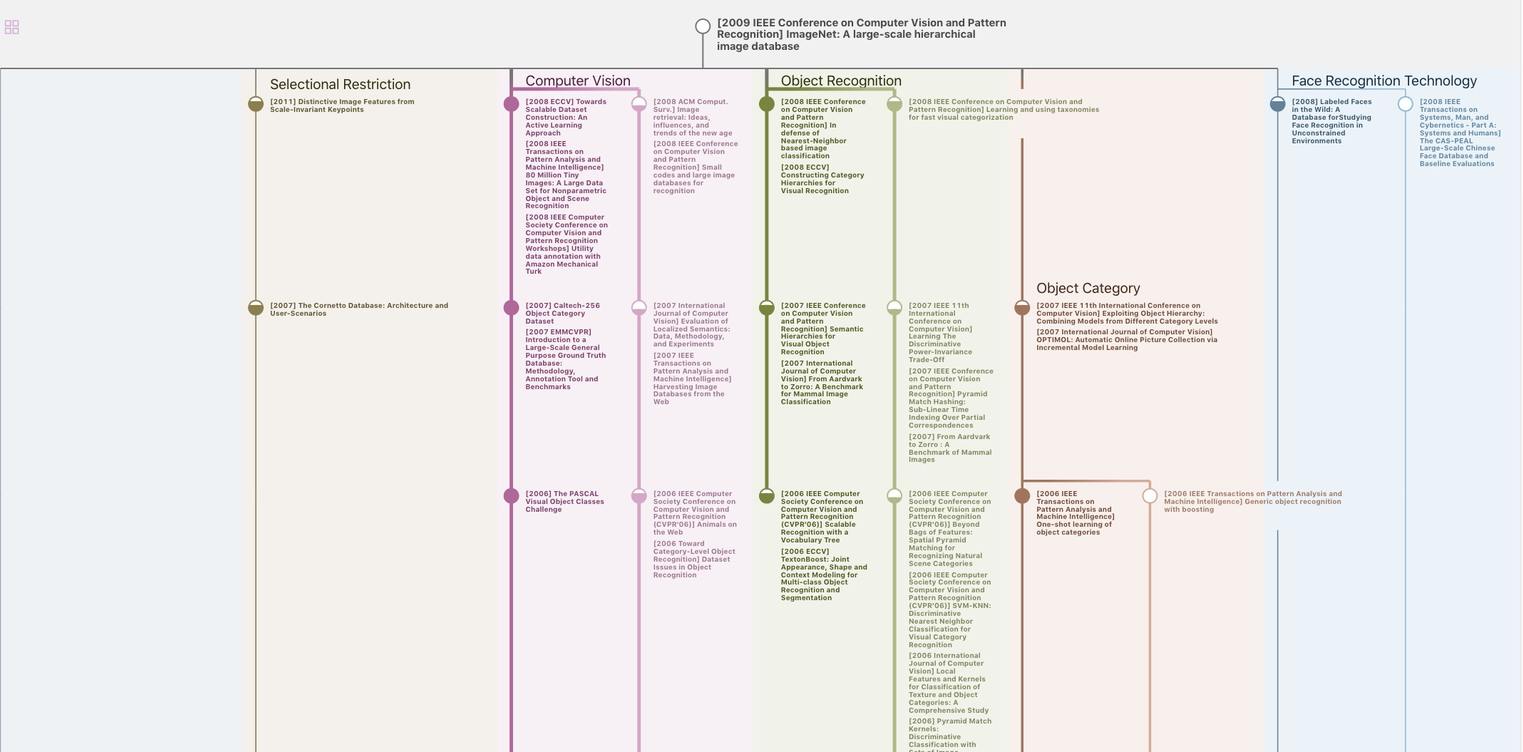
生成溯源树,研究论文发展脉络
Chat Paper
正在生成论文摘要