Reinforcement Learning Driven Intra-modal and Inter-modal Representation Learning for 3D Medical Image Classification
MEDICAL IMAGE COMPUTING AND COMPUTER ASSISTED INTERVENTION, MICCAI 2022, PT III(2022)
摘要
Multi-modality 3D medical images play an important role in the clinical practice. Due to the effectiveness of exploring the complementary information among different modalities, multi-modality learning has attracted increased attention recently, which can be realized by Deep Learning (DL) models. However, it remains a challenging task for two reasons. First, the prediction confidence of multi-modality learning network cannot be guaranteed when the model is trained with weakly-supervised volume-level labels. Second, it is difficult to effectively exploit the complementary information across modalities and also preserve the modality-specific properties when fusion. In this paper, we present a novel Reinforcement Learning (RL) driven approach to comprehensively address these challenges, where two Recurrent Neural Networks (RNN) based agents are utilized to choose reliable and informative features within modality (intra-learning) and explore complementary representations across modalities (inter-learning) with the guidance of dynamic weights. These agents are trained via Proximal Policy Optimization (PPO) with the confidence increment of the prediction as the reward. We take the 3D image classification as an example and conduct experiments on a multi-modality brain tumor MRI data. Our approach outperforms other methods when employing the proposed RL-based multi-modality representation learning.
更多查看译文
关键词
Multi-modality learning, 3D medical images, Reinforcement learning, Classification
AI 理解论文
溯源树
样例
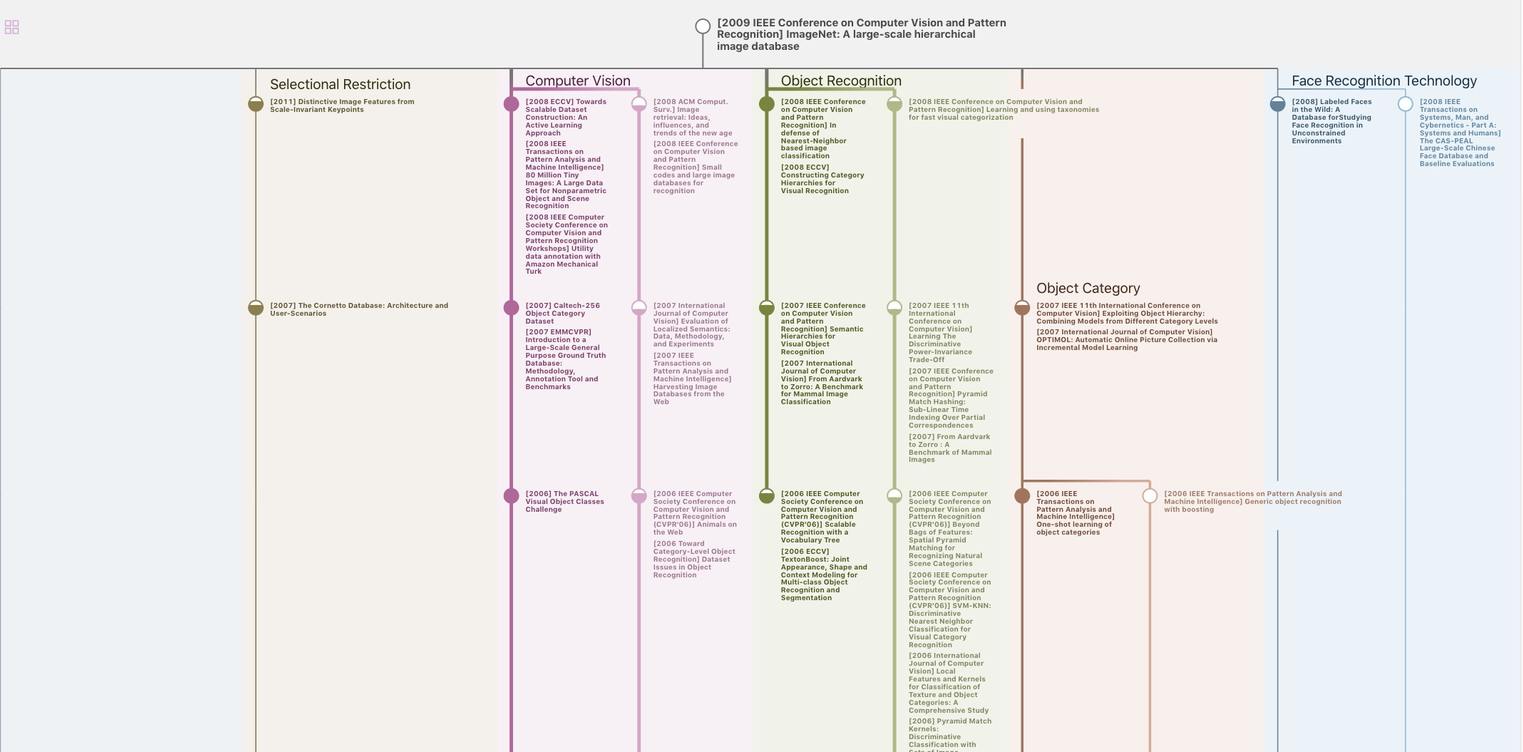
生成溯源树,研究论文发展脉络
Chat Paper
正在生成论文摘要