DeepMIF: Deep Learning Based Cell Profiling for Multispectral Immunofluorescence Images with Graphical User Interface
MEDICAL IMAGE COMPUTING AND COMPUTER ASSISTED INTERVENTION, MICCAI 2022, PT IV(2022)
摘要
Multispectral immunofluorescence (M-IF) analysis is used to investigate the cellular landscape of tissue sections and spatial interaction of cells. However, complex makeup of markers in the images hinders the accurate quantification of cell phenotypes. We developed DeepMIF, a new deep learning (DL) based tool with a graphical user interface (GUI) to detect and quantify cell phenotypes on M-IF images, and visualize whole slide image (WSI) and cell phenotypes. To identify cell phenotypes, we detected cells on the deconvoluted images followed by coexpression analysis to classify cells expressing single or multiple markers. We trained, tested and validated our model on > 50k expert single-cell annotations from multiple immune panels on 15 samples of follicular lymphoma patients. Our algorithm obtained a cell classification accuracy and area under the curve (AUC) >= 0.98 on an independent validation panel. The cell phenotype identification took on average 27.5 min per WSI, and rendering of the WSI took on average 0.07 minutes. DeepMIF is optimized to run on local computers or high-performance clusters independent of the host platform. These suggest that the DeepMIF is an accurate and efficient tool for the analysis and visualization of M-IF images, leading to the identification of novel prognostic cell phenotypes in tumours.
更多查看译文
关键词
Deep learning, Multispectral immunofluorescence, Cell detection, Cell classification, Image viewer
AI 理解论文
溯源树
样例
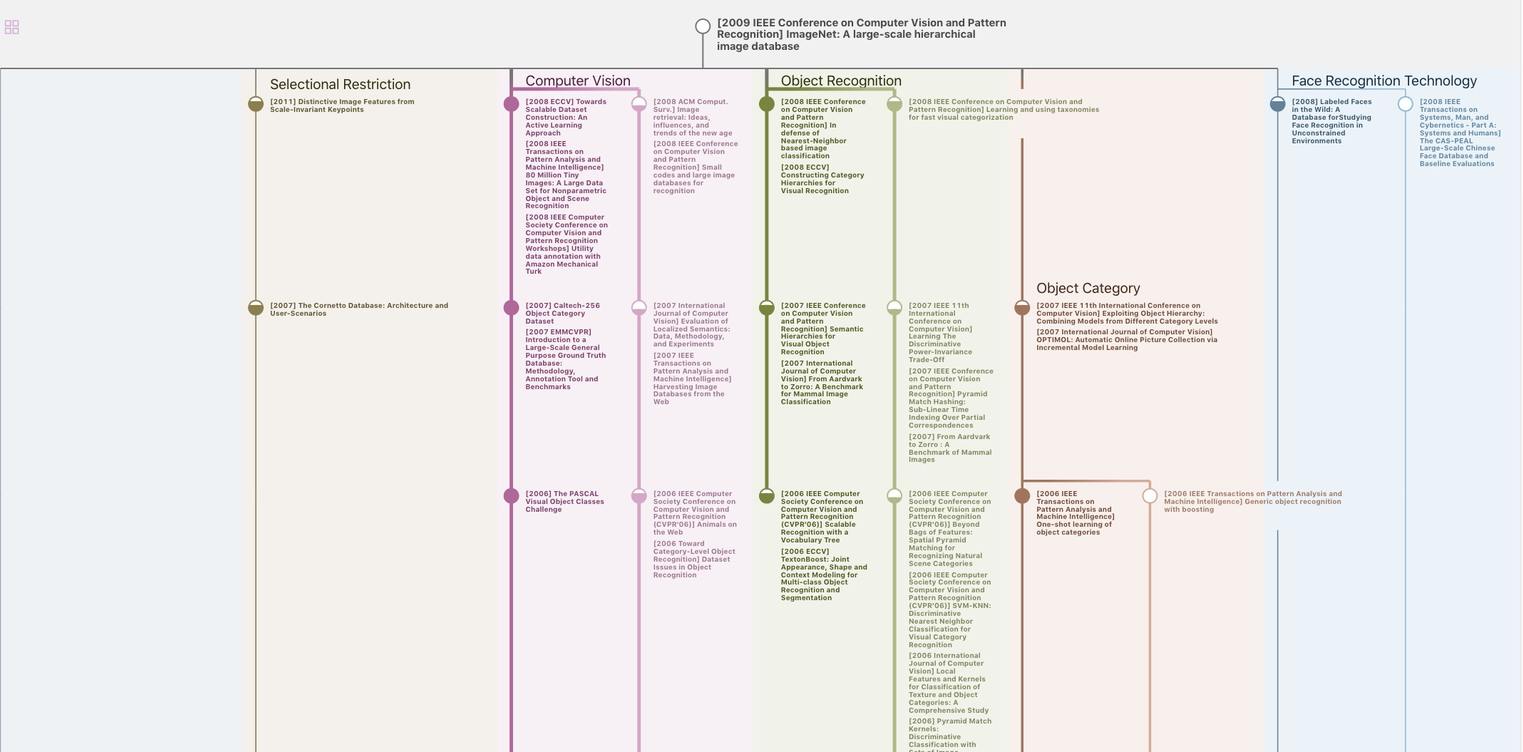
生成溯源树,研究论文发展脉络
Chat Paper
正在生成论文摘要