Adaptive Function Placement with Distributed Deep Reinforcement Learning in RAN Slicing
2022 IEEE 95TH VEHICULAR TECHNOLOGY CONFERENCE (VTC2022-SPRING)(2022)
摘要
The 5th generation mobile communication system (5G system) is expected to support various communication services which require different aspects of quality of service (QoS). To support diverse services, network slicing in a radio access network (RAN slicing) has been proposed to provide a customized network for various services as a slice. In RAN slicing, QoS satisfaction can be achieved by controlling RAN function placement (RFP) under the constraint of the limited network resources to transmit user data. To maximize QoS satisfaction, we consider distributed deep reinforcement learning (distributed DRL) for controlling RFP. In distributed DRL, it is important to design a reward and a state. To achieve high QoS satisfaction, we evaluated several reward and state candidates by conducting numerical simulations with several sets of services, and present the best design for reward and state.
更多查看译文
关键词
5G mobile communication, RAN slicing, Function placement, Reinforcement learning
AI 理解论文
溯源树
样例
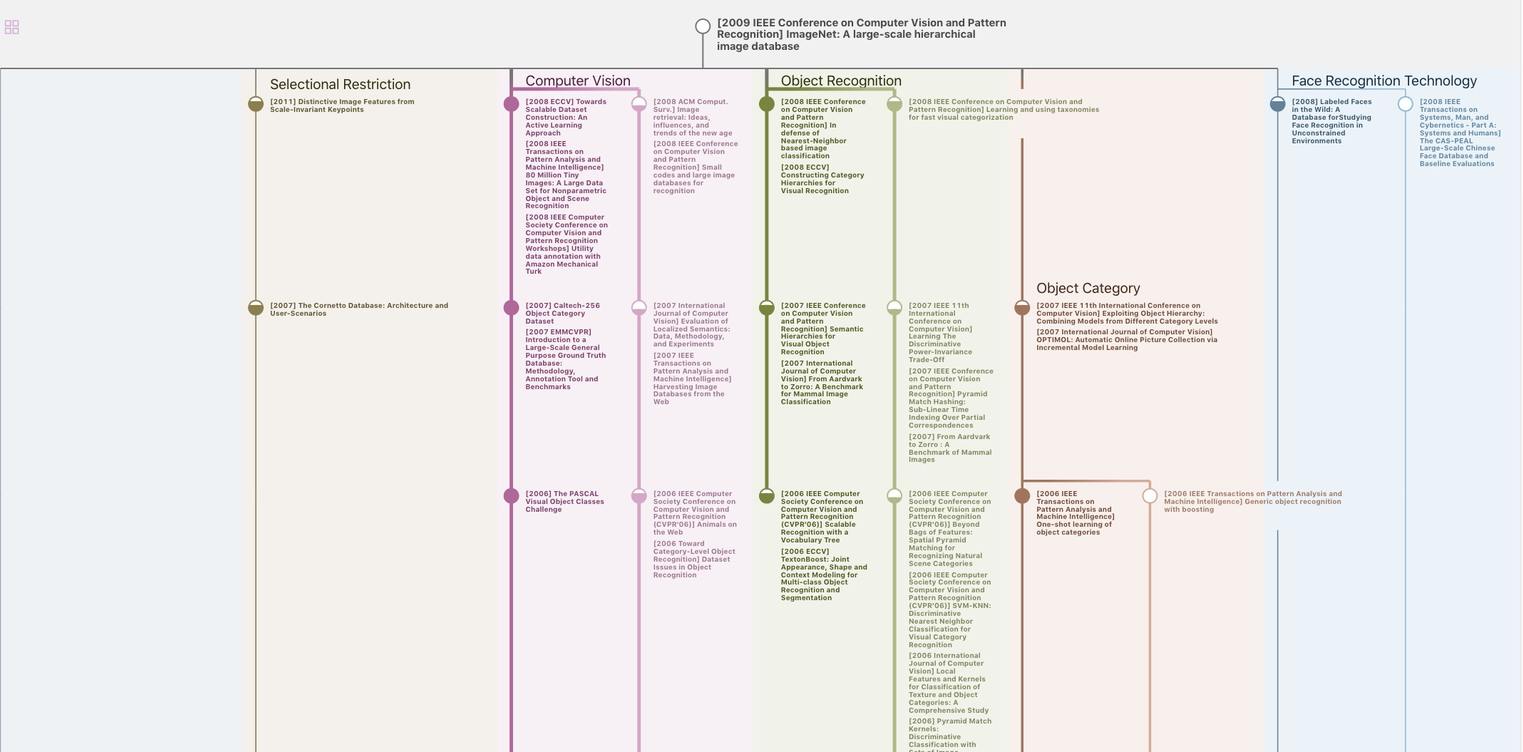
生成溯源树,研究论文发展脉络
Chat Paper
正在生成论文摘要