Optimal Control of Active Distribution Network Using Deep Reinforcement Learning
2022 IEEE International Conference on Environment and Electrical Engineering and 2022 IEEE Industrial and Commercial Power Systems Europe (EEEIC / I&CPS Europe)(2022)
摘要
Distribution network power losses is responsible for a large portion of system energy losses. In an active distribution network (ADN), the greater penetration of distribution generations (DGs) excel to bidirectional power flow that are responsible for large voltage excursions. To address this problem, this research paper proposes a deep reinforcement learning strategy for ADN optimal voltage control that comprises of a solid-state transformer (SST). The proposed scheme computes optimal values of SST's reactive power that helps to solve the problem of ADN bus voltage excursions. Furthermore, in this strategy, the optimal control issue is expressed as a Markov decision process (MDP) that deals with continuous states and action spaces. The deep deterministic policy gradient (DDPG) algorithm is used to study the reactive power control strategies to determine the optimal actions from given states by utilizing the data driven deep neural networks (DNNs). Numerical simulations on modified IEEE 33-bus system using MATLAB show that the proposed strategy effectively sustains entire bus voltage in the allowable limits, and lessens the power loss of the system.
更多查看译文
关键词
active distribution network,deep neural networks,deep deterministic policy gradient,optimal voltage control,reinforcement learning,solid state transformer
AI 理解论文
溯源树
样例
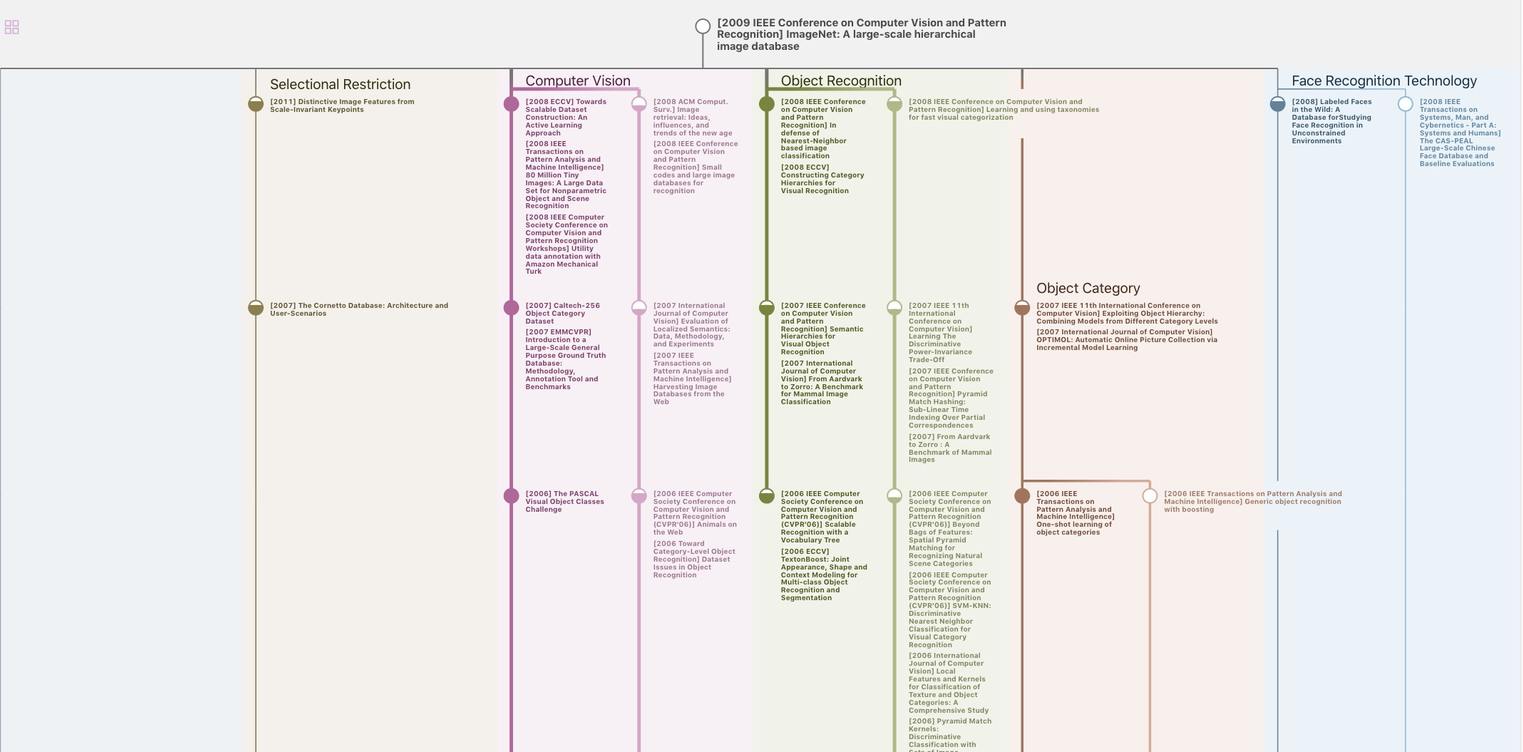
生成溯源树,研究论文发展脉络
Chat Paper
正在生成论文摘要