Motor imagery classification using sparse nonnegative matrix factorization and convolutional neural networks
NEURAL COMPUTING & APPLICATIONS(2024)
摘要
The motor movement performed by different body parts affects the synaptic potential at different brain cortices, which can be observed by the electroencephalogram (EEG) signal. The recorded EEG signals can be used to decode the imagined motor task. The EEG signals are non-stationary and transient and contain time, frequency, and space information. Extracting this information and processing them with the latest machine learning and deep learning algorithms can be useful for brain-computer interfacing and other human-machine interaction techniques. EEG signal contains negative values. Hence, nonnegative matrix factorization can be used to provide a meaningful explanation of information within EEG signals. Sparseness in feature vectors is another essential factor to consider while identifying the structures in an input signal. In this work, we propose a novel motor imagery classification model that extracts the weights for predefined motor imagery features from EEG signals and classifies them using a convolution neural network (CNN). Sparse nonnegative matrix factorization is used to extract the fundamental feature vectors for different motor imagery events, which are further used to extract the combined weight matrix of unknown motor imagery events. The designed CNN classifies the extracted weight matrix in the corresponding classes. The acquired EEG signals from all the channels are processed simultaneously using the CNN, which helps extract spatial information from the signals. BCI Competition IV dataset IIa and BCI Competition III dataset IVa are used to validate the proposed method. The proposed method has been compared with existing methods and validates their superiority in terms of average accuracy. The classification accuracy for two types and four types of motor imagery signals is 99.53% and 94.58%, respectively. Empirical results show that EEG signals' sparseness characteristics can be considered an effective feature for motor imagery classification.
更多查看译文
关键词
Motor imagery,Classification,Convolutional neural networks,SNMF
AI 理解论文
溯源树
样例
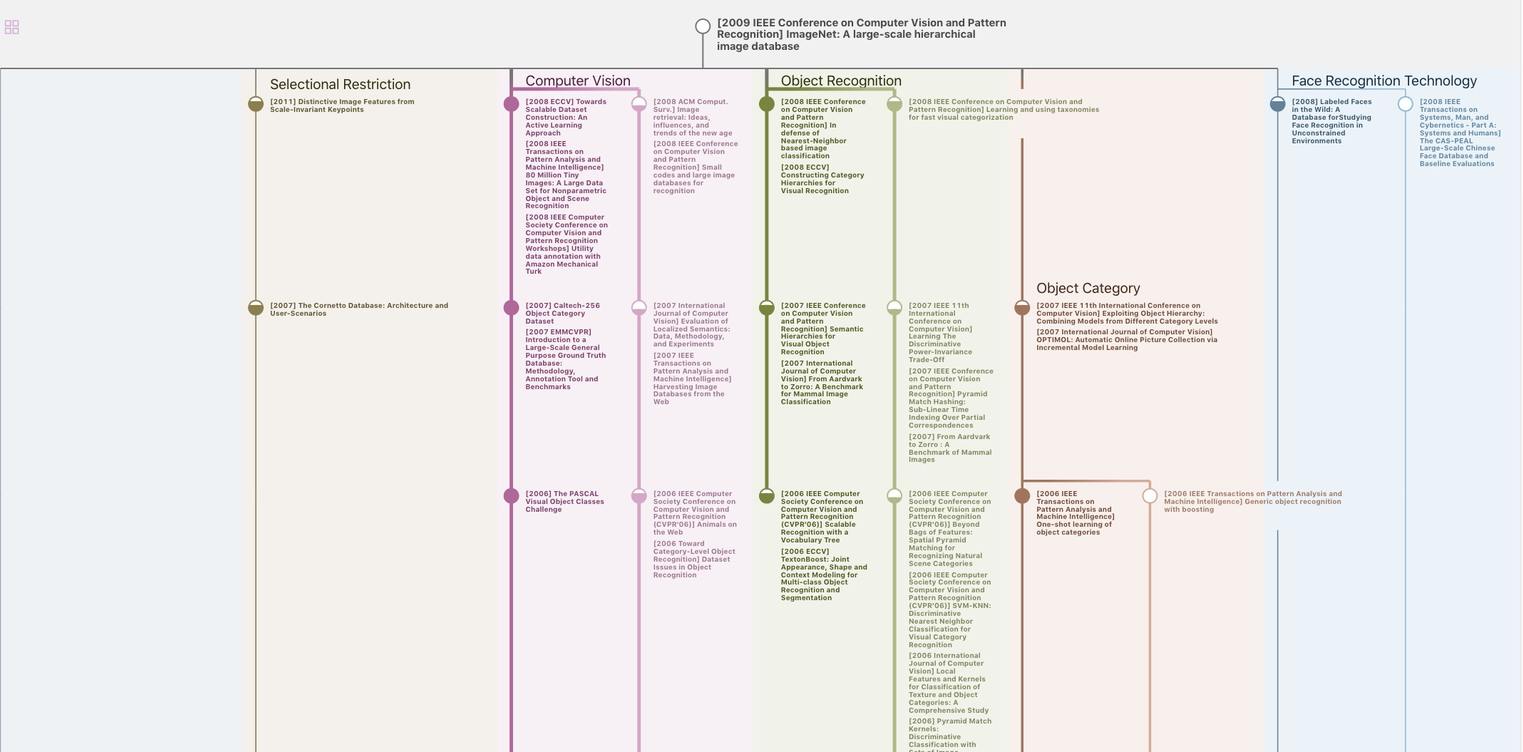
生成溯源树,研究论文发展脉络
Chat Paper
正在生成论文摘要