UTILISING SIMULATED TREE DATA TO TRAIN SUPERVISED CLASSIFIERS
XXIV ISPRS CONGRESS IMAGING TODAY, FORESEEING TOMORROW, COMMISSION II(2022)
摘要
The aim of our research was to examine whether simulated forest data can be utilized for training supervised classifiers. We included two classifiers namely the random forest classifier and the novel convolutional neural network classifier that utilizes feature images. We simulated tree parameters and created a feature vector for each tree. The original feature vector was utilised with random forest classifier. However, these feature vectors were also converted into feature images suitable for input into a YOLO (You Only Look Once) convolutional neural network classifier. The selected features were red colour, green colour, near-infrared colour, tree height divided by canopy diameter, and NDVI. The random forest classifier and convolutional neural network classifier performed similarly both with simulated data and field-measured reference data. As a result, both methods were able to identify correctly 97.5 % of the field-measured reference trees. Simulated data allows much larger training data than what could be feasible from field measurements.
更多查看译文
关键词
convolutional neural network, YOLO, simulation, feature image, classification
AI 理解论文
溯源树
样例
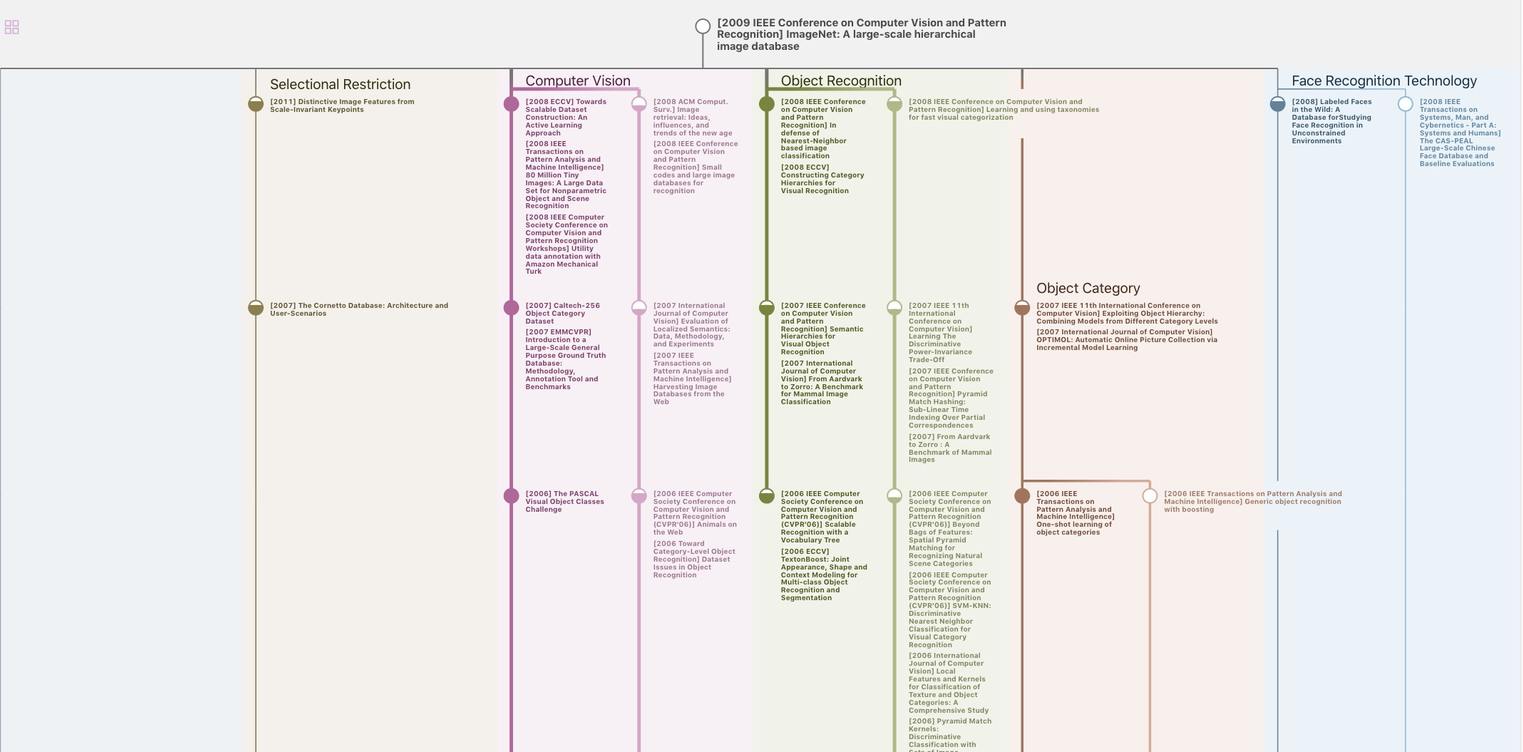
生成溯源树,研究论文发展脉络
Chat Paper
正在生成论文摘要