Multi-agent Pathfinding with Communication Reinforcement Learning and Deadlock Detection
INTELLIGENT ROBOTICS AND APPLICATIONS (ICIRA 2022), PT I(2022)
摘要
The learning-based approach has been proved to be an effective way to solve multi-agent path finding (MAPF) problems. For large warehouse systems, the distributed strategy based on learning method can effectively improve efficiency and scalability. But compared with the traditional centralized planner, the learning-based approach is more prone to deadlocks. Communication learning has also made great progress in the field of multi-agent in recent years and has been be introduced into MAPF. However, the current communication methods provide redundant information for reinforcement learning and interfere with the decision-making of agents. In this paper, we combine the reinforcement learning with communication learning. The agents select its communication objectives based on priority and mask off redundant communication links. Then we use a feature interactive network based on graph neural network to achieve the information aggregation. We also introduce an additional deadlock detection mechanism to increase the likelihood of an agent escaping a deadlock. Experiments demonstrate our method is able to plan collision-free paths in different warehouse environments.
更多查看译文
关键词
Multi-agent path finding, Reinforcement learning, Communication learning, Deadlock detection
AI 理解论文
溯源树
样例
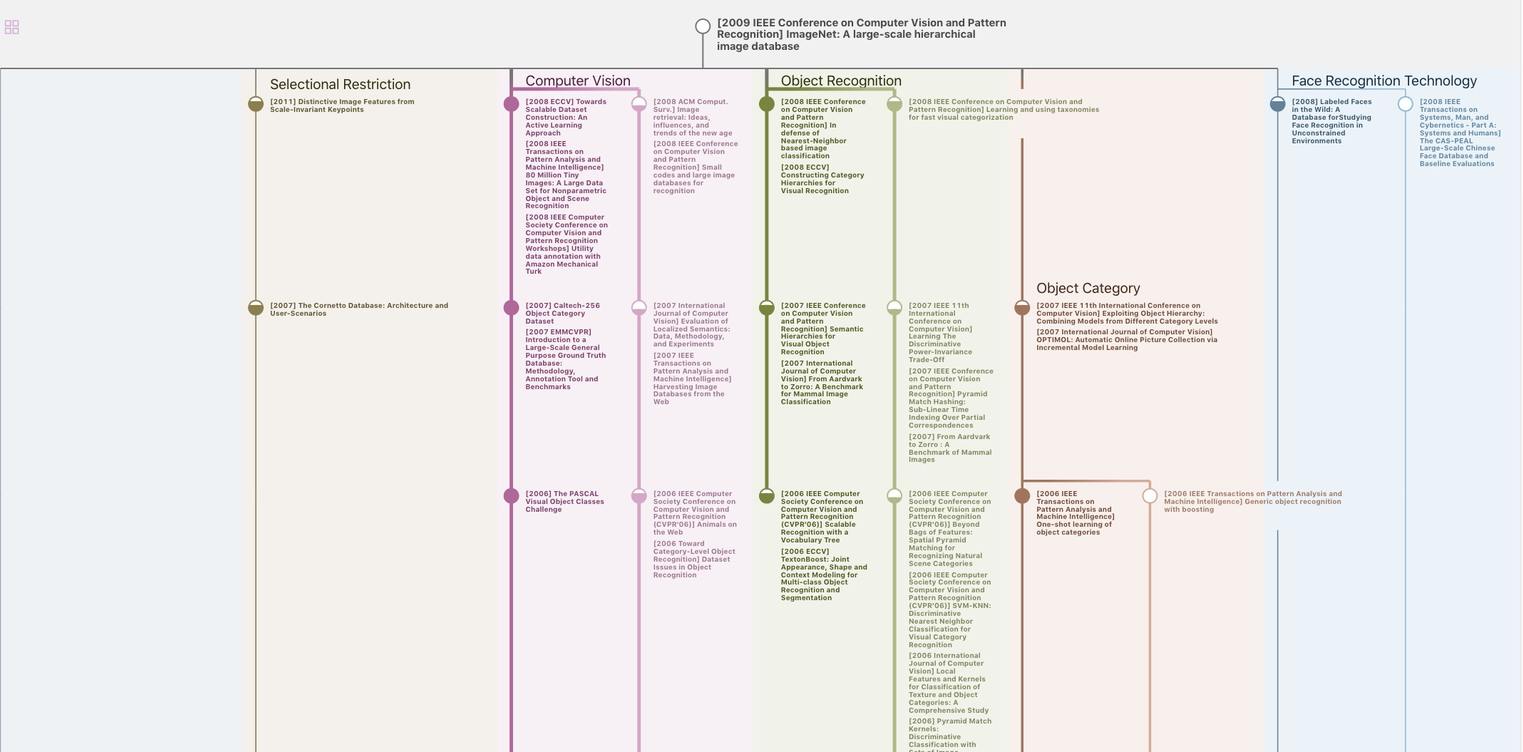
生成溯源树,研究论文发展脉络
Chat Paper
正在生成论文摘要