A Swarm Intelligence Approach: Combination of Different EEG-Channel Optimization Techniques to Enhance Emotion Recognition
HUMAN-COMPUTER INTERACTION: TECHNOLOGICAL INNOVATION, PT II(2022)
摘要
This paper describes well-known classification techniques which are evaluated for emotion classification. The aim of this work is the comparison of different channel selection techniques to achieve fast computation for electroencephalogram (EEG) data. Swarm intelligence algorithms belong to the class of nature-inspired algorithms which are very useful in achieving high accuracies while reducing the computing cost. In this paper different channel optimization techniques are compared to each other. They are applied to the DEAP dataset to find the most suitable channels in the context of emotion recognition. For channel selection, principal component analysis (PCA), maximum relevance-minimum redundancy (mRMR), particle swarm optimization (PSO), cuckoo search (CS) and grey wolf optimization (GWO) were investigated. By applying these optimization algorithms, the number of EEG channels could be reduced from 32 to 20 while the accuracy remained nearly the same. The proposed optimizations techniques saved between two- and seven-hours of computing time in training the Bidirectional Long Short-Term Memory model to classify emotions, while the computing time without channel selection took 18 h. Among these algorithms, mRMR and CS obtained the most promising results. By using mRMR a total computing time of 11 hwith an accuracy of 92.74% for arousal and 92.36% for valencewas achieved. For CS a total computing time of 15 h was achieved, with an accuracy of 93.33% for arousal and 93.67% for valence.
更多查看译文
关键词
Emotion recognition, Channel selection, Swarm intelligence, EEG, mRMR, Cuckoo search, Computing time reduction
AI 理解论文
溯源树
样例
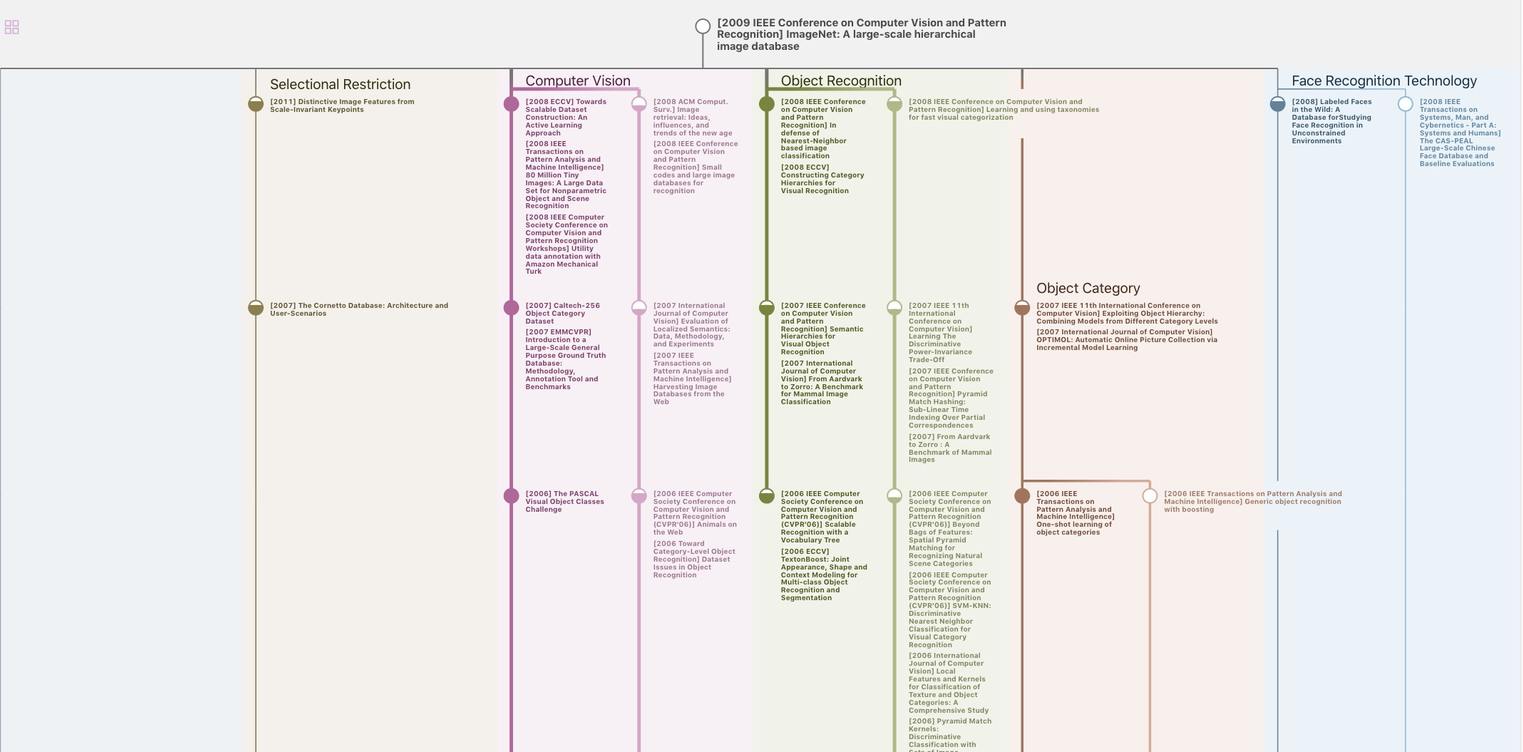
生成溯源树,研究论文发展脉络
Chat Paper
正在生成论文摘要