Improving Model Inference via W-Set Reduction
TESTING SOFTWARE AND SYSTEMS, ICTSS 2021(2022)
摘要
Model inference is a form of systematic testing of black-box systems while learning at the same time a model of their behaviour. In this paper, we study the impact of W-set reduction in hW-inference, an inference algorithm for learning models from scratch. hW-inference relies on progressively extending a sequence h into a homing sequence for the system, and a set W of separating sequences into a fully characterizing set. Like most other inference algorithms, it elaborates intermediate conjectures which can be refined through counterexamples provided by an oracle. We observed that the size of the W-set could vary by an order of magnitude when using random counterexamples. Consequently, the length of the test suite is hugely impacted by the size variation of the W-set. Whereas the original hW-inference algorithm keeps increasing the W-set until it is characterizing, we propose reassessing the set and pruning it based on intermediate conjectures. This can lead to a shorter test suite to thoroughly learn a model. We assess the impact of reduction methods on a self-scanning system as used in supermarkets, where the model we get is a finite state machine with 121 states and over 1800 transitions, leading to an order of magnitude of around a million events for the trace length of the inference.
更多查看译文
关键词
model inference,reduction,w-set
AI 理解论文
溯源树
样例
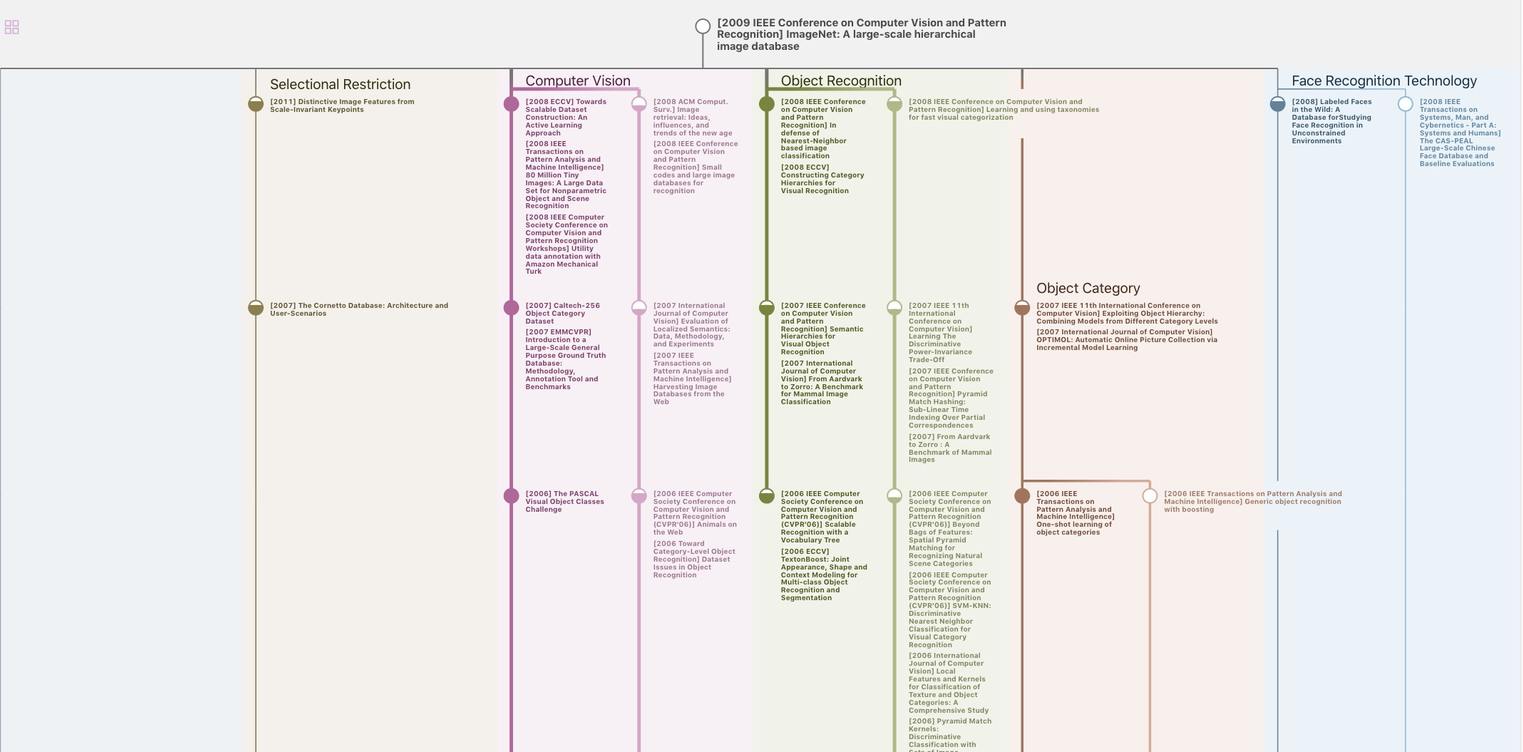
生成溯源树,研究论文发展脉络
Chat Paper
正在生成论文摘要