GAN-Based Data Augmentation for Prediction Improvement Using Gene Expression Data in Cancer
COMPUTATIONAL SCIENCE - ICCS 2022, PT III(2022)
摘要
Within the area of bioinformatics, Deep Learning (DL) models have shown exceptional results in applications in which histological images, scans and tomographies are used. However, when gene expression data is under analysis, the performance is often limited, further hampered by the complexity of these models that require several instances, in the order of thousands, to provide good results. Due to the difficulty and the costs involved in the collection of medical data, the application of Data Augmentation (DA) techniques to alleviate the lack of samples is a topic of great relevance. State-of-the-art models based on Conditional Generative Adversarial Networks (CGAN) and some introduced modifications are used in this work to investigate the effect of DA for prediction of the vital status of patients from RNA-Seq gene expression data. Experimental results on several real-world data sets demonstrate the effectiveness and efficiency of the proposed models. The application of DA methods significantly increase prediction accuracy, leading by 12% with respect to benchmark data sets and 3.15% with respect to data processed with feature selection. Results based on CGAN models outperform in most cases, alternative methods like the SMOTE or noise injection techniques.
更多查看译文
关键词
Data Augmentation, Gene expression, Bioinformatics, Deep Learning, CGAN
AI 理解论文
溯源树
样例
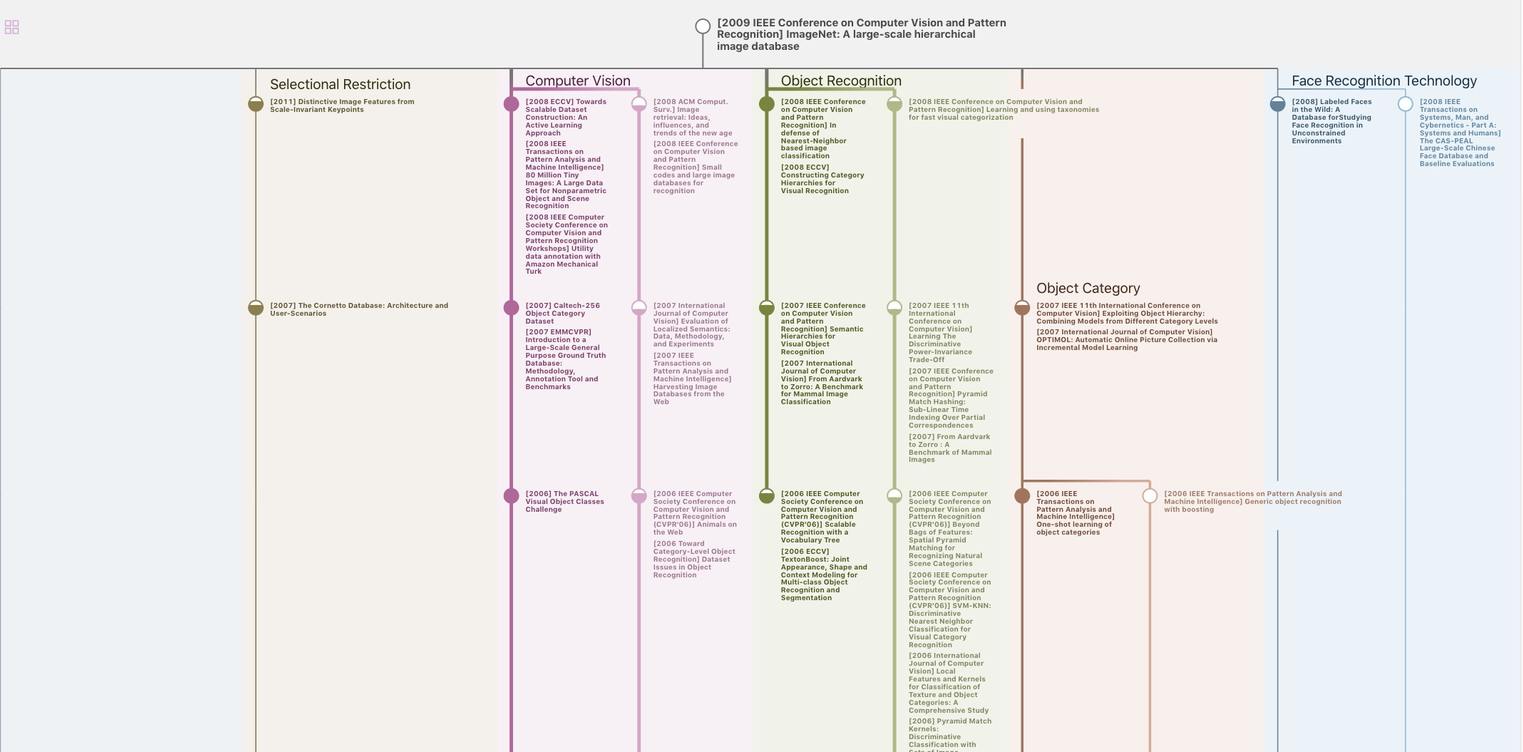
生成溯源树,研究论文发展脉络
Chat Paper
正在生成论文摘要