Predicting Argument Density from Multiple Annotations
NATURAL LANGUAGE PROCESSING AND INFORMATION SYSTEMS (NLDB 2022)(2022)
Abstract
Annotating a corpus with argument structures is a complex task, and it is even more challenging when addressing text genres where argumentative discourse markers do not abound. We explore a corpus of opinion articles annotated by multiple annotators, providing diverse perspectives of the argumentative content therein. New annotation aggregation methods are explored, diverging from the traditional ones that try to minimize presumed errors from annotator disagreement. The impact of our methods is assessed for the task of argument density prediction, seen as an initial step in the argument mining pipeline. We evaluate and compare models trained for this regression task in different generated datasets, considering their prediction error and also from a ranking perspective. Results confirm the expectation that addressing argument density from a ranking perspective is more promising than looking at the problem as a mere regression task. We also show that probabilistic aggregation, which weighs tokens by considering all annotators, is a more interesting approach, achieving encouraging results as it accommodates different annotator perspectives. The code and models are publicly available at https://github.com/DARGMINTS/argument density.
MoreTranslated text
Key words
Argument annotation,Perspectivist NLP,Argument density prediction,Argument mining
AI Read Science
Must-Reading Tree
Example
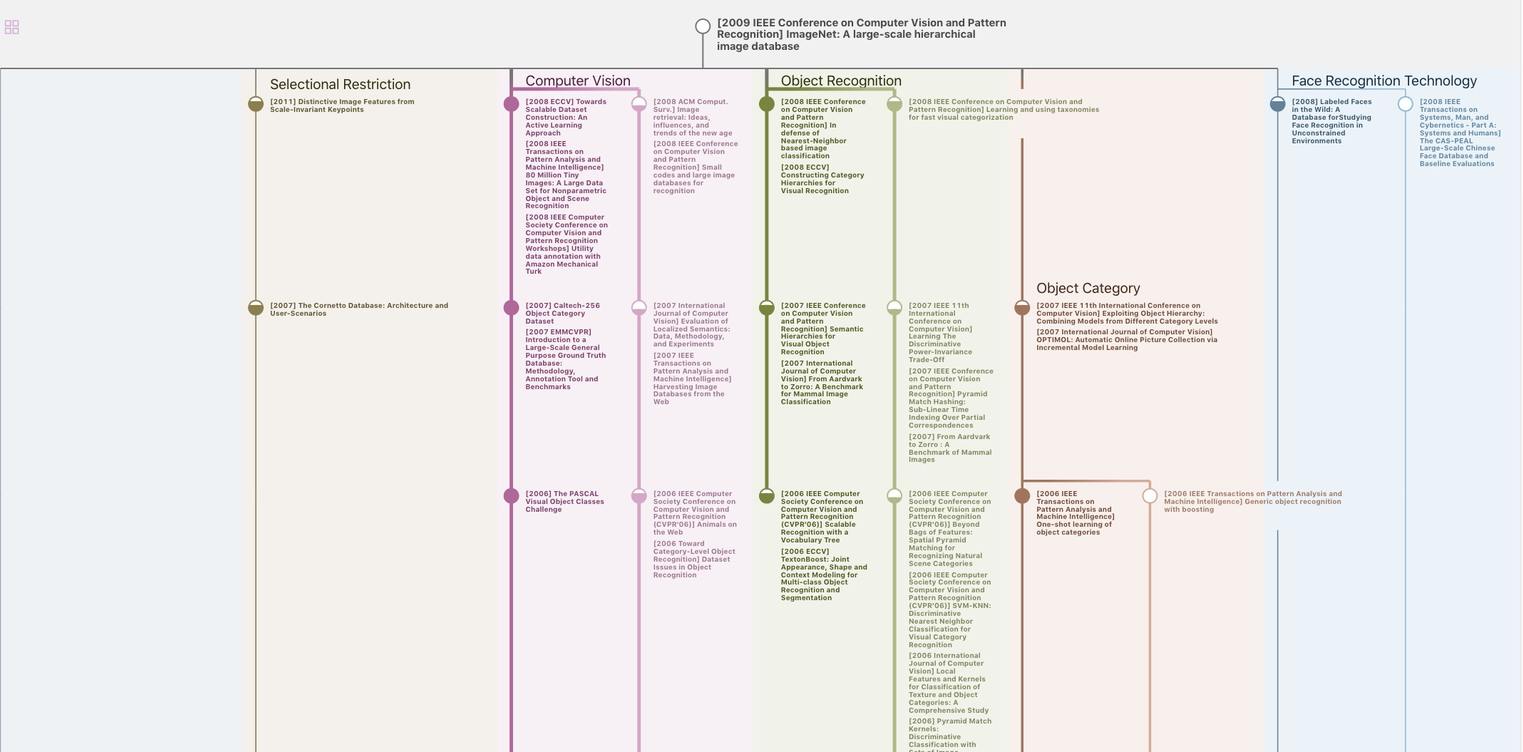
Generate MRT to find the research sequence of this paper
Chat Paper
Summary is being generated by the instructions you defined