Multi-instance Embedding Learning Through High-level Instance Selection
ADVANCES IN KNOWLEDGE DISCOVERY AND DATA MINING, PAKDD 2022, PT II(2022)
摘要
Multi-instance learning (MIL) handles complex structured data represented by bags and their instances. MIL embedded algorithms based on representative instance selection transform bags into a single-instance space. However, they may select weak representative instances due to the ignorance of the internal bag structure. In this paper, we propose the multi-instance embedding learning through high-level instance selection (MIHI) algorithm with two techniques. The fast bag-inside instance selection technique obtains instance prototypes of each bag. It fully utilizes the bag information using our new density and affinity metrics. Based on the instance prototypes, the high-level instance selection technique chooses instances using the peak density metric. It obtains high-level instances with higher representative power than the instance prototypes. Experiments were conducted on six learning tasks and nine comparison algorithms. The results confirmed that MIHI achieved better performance in terms of efficiency and classification accuracy. This method, in particular, has a substantial advantage in image retrieval and web data sets.
更多查看译文
关键词
Embedding,High-level instance,Instance selection,Multi-instance learning
AI 理解论文
溯源树
样例
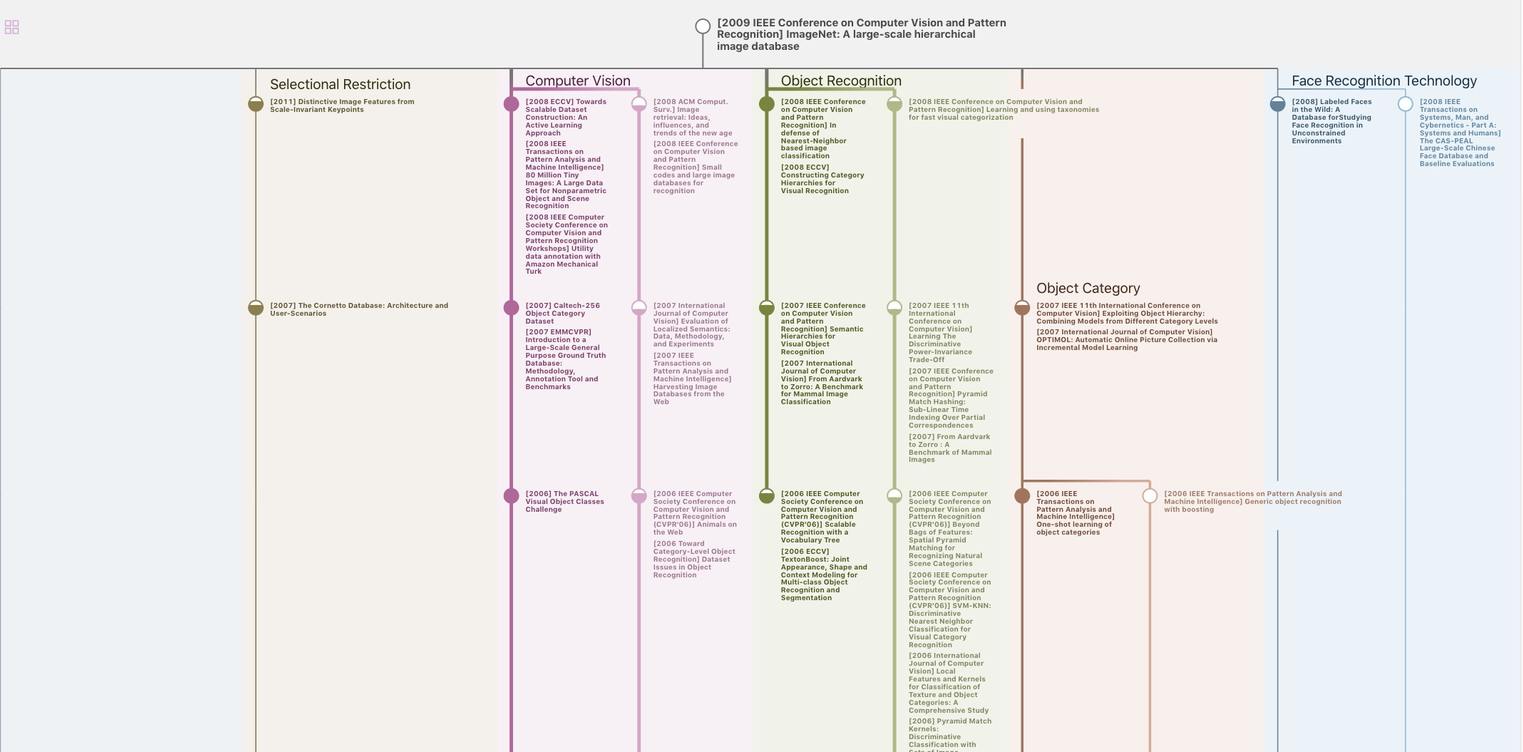
生成溯源树,研究论文发展脉络
Chat Paper
正在生成论文摘要