Towards Better Generalization for Neural Network-Based SAT Solvers
ADVANCES IN KNOWLEDGE DISCOVERY AND DATA MINING, PAKDD 2022, PT II(2022)
摘要
Neural network (NN) has demonstrated its astonishing power in many data mining tasks. Recently, NN is adapted to the boolean satisfiability (SAT) problem as a solver, which is trained on a dataset containing the satisfiable annotation of a series of logical expressions. In SAT problem, each expression is composed of the conjunction of logical variables. The effectiveness of NN as a solver to SAT has been verified empirically when the training and test data contain the same group of logical variables. However, when the test set contains more logical variables, test performance significantly degenerates; that is, the generalization performance on the test set containing more logical variables is far below expected. In this paper, we conjecture that the degeneration may be due to that a non-trivial way is requested to calibrate the continuous output by NN. Based on the conjecture, we design a generalized framework that is expected to improve the NN solver's performance when the test data include much more variables. Specifically, a Temperature-Scaled Neural Network SAT solver (TenSAT) adds a special calibration component to the message-passing NN. Experiments demonstrate the correctness of the conjecture, i.e., TenSAT can stop the test performance from degrading when the test set contains new variables as much as ten folds of the training ones.
更多查看译文
关键词
Neural network, SAT, Generalization, TenSAT
AI 理解论文
溯源树
样例
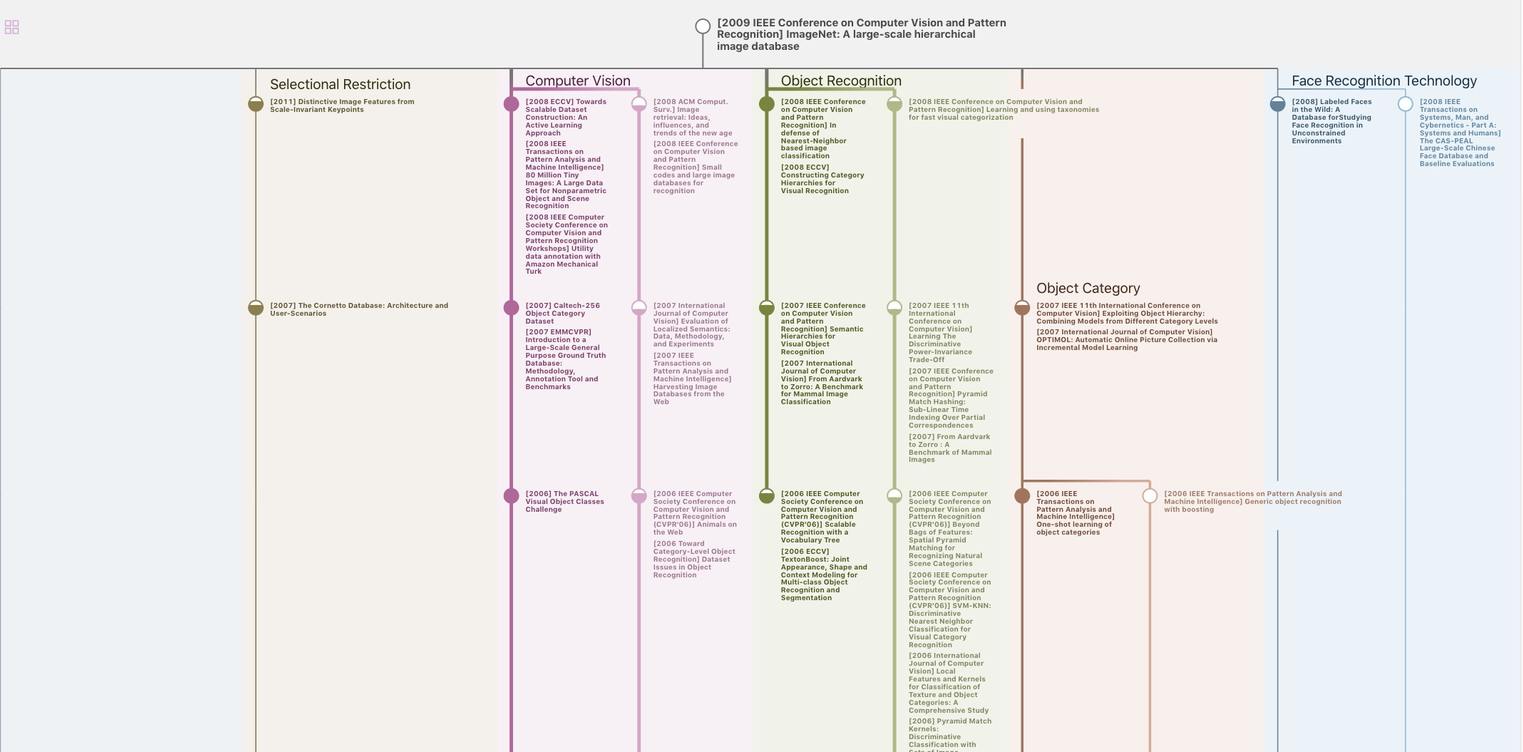
生成溯源树,研究论文发展脉络
Chat Paper
正在生成论文摘要