FLiB: Fair Link Prediction in Bipartite Network
ADVANCES IN KNOWLEDGE DISCOVERY AND DATA MINING, PAKDD 2022, PT II(2022)
摘要
Graph neural networks have become a popular modeling choice in many real-world applications like social networks, recommender systems, molecular science. GNNs have been shown to exhibit greater bias compared to other ML models trained on i.i.d data, and as they are applied to many socially-consequential use-cases, it becomes imperative for the model results and learned representations to be fair. Real-world applications of GNNs involve learning over heterogeneous networks with several nodes and edge types. We show that various kinds of nodes in a heterogeneous network can pick bias from a particular node type and remain non-trivial to debias using standard fairness algorithms. We propose a novel framework- Fair Link Prediction in Bipartite Networks (FLiB) that ensures fair link prediction while learning fair representations for all types of nodes with respect to the sensitive attribute of one of the node type. We further propose S-FLiB, which effectively mitigates bias at the subgroup level by regularising model predictions for subgroups defined over problem-specific grouping criteria.
更多查看译文
关键词
Fairness, GNN, Link prediction, Bipartite graph
AI 理解论文
溯源树
样例
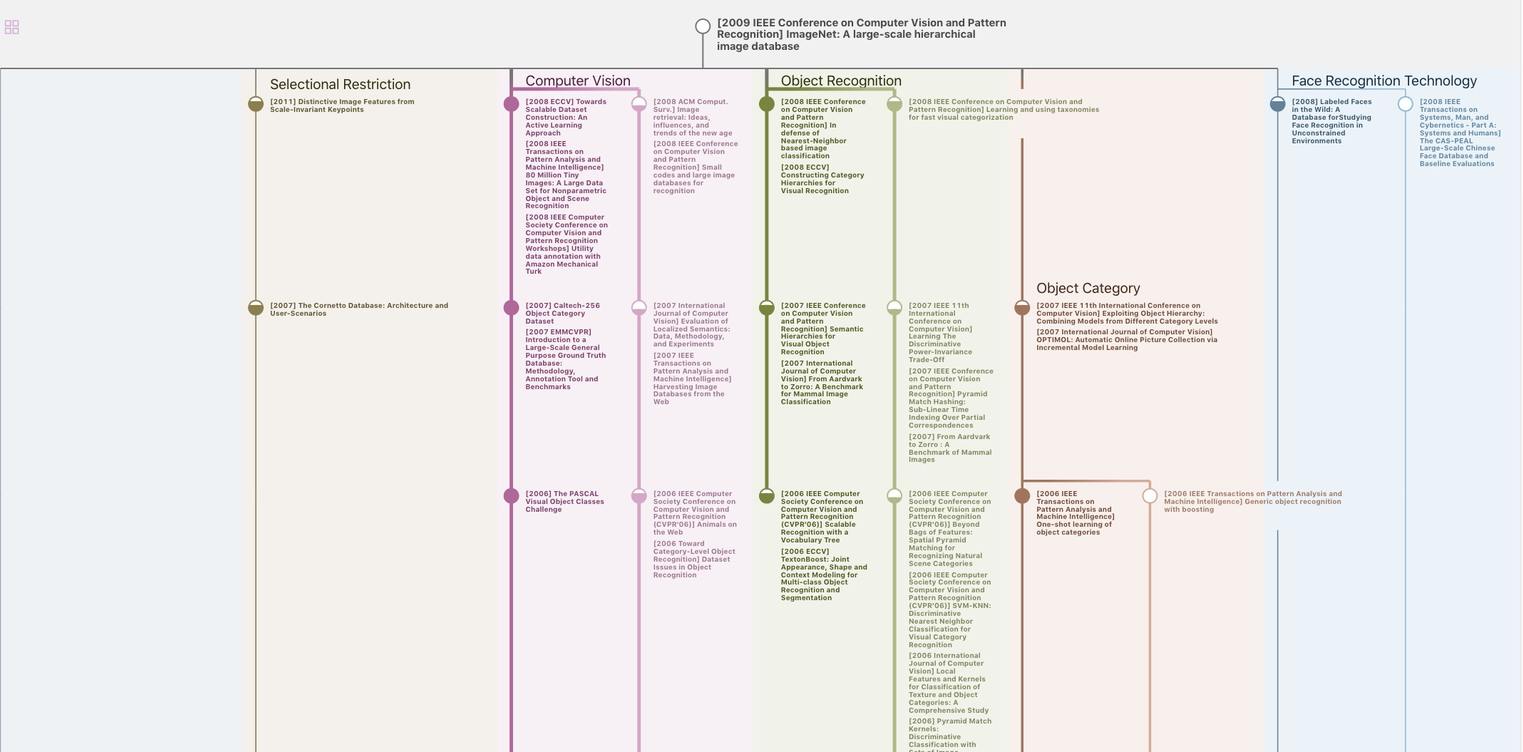
生成溯源树,研究论文发展脉络
Chat Paper
正在生成论文摘要