Studying Cohort Influence on Student Performance Prediction in Multi-cohort University Courses
EDUCATING FOR A NEW FUTURE: MAKING SENSE OF TECHNOLOGY-ENHANCED LEARNING ADOPTION, EC-TEL 2022(2022)
摘要
Advances in educational data mining and learning analytics techniques allow instructors and institutions to analyze log data generated from learning management systems to inform themselves about student learning and success. Over the years, several machine learning techniques have been developed, used, and researched to provide more accurate predictions of students' performance in courses. These techniques commonly need and are focused on large sample sizes and low dimensionality which is not the case for university courses in blended contexts. In some studies, student cohorts across years and programs are merged together to increase sample sizes and achieve better prediction accuracies. While there have been other recent studies experimenting with lower samples and fewer dimensions, they do not focus on cohort influences on prediction. There is a need to study this area of analysis and prediction in multi-cohort university courses both to inform (a) instructors, of key course features, prediction insights, and student study behavior to offer relevant interventions to specific cohorts and (b) curriculum designers, who can gain insights into improving program designs. In this study, we look for empirical evidence if performance metrics and key features in prediction are influenced by cohorts in multi-cohort university courses.
更多查看译文
关键词
Predictive learning analytics, Higher education, Multi-cohort university courses
AI 理解论文
溯源树
样例
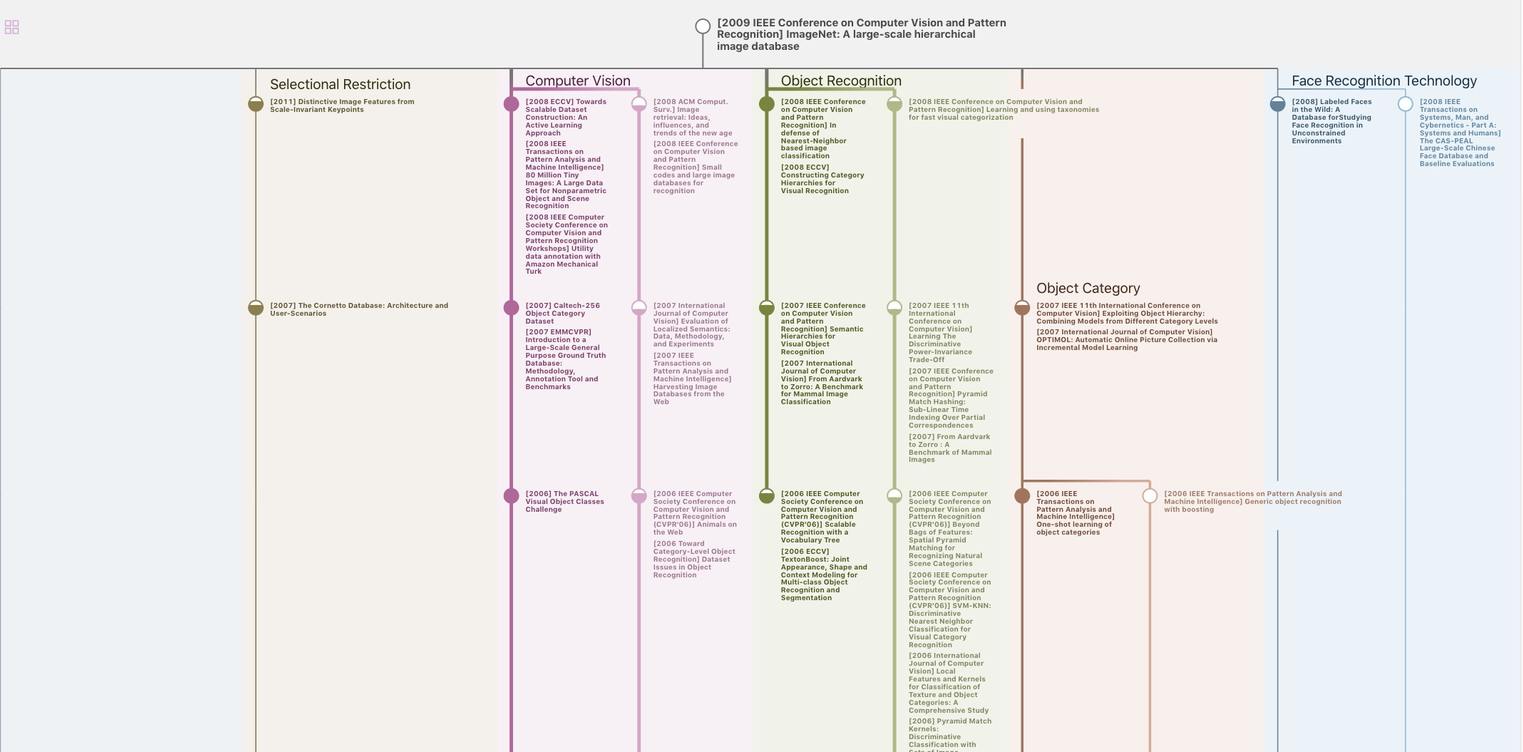
生成溯源树,研究论文发展脉络
Chat Paper
正在生成论文摘要