Differential Learning from Sparse and Noisy Labels for Robust Detection of Clinical Landmarks in Echo Cine Series
SIMPLIFYING MEDICAL ULTRASOUND, ASMUS 2022(2022)
摘要
Quantifying dimensions of the Left Ventricle (LV) chamber of the heart on echocardiography (echo) cine series is an essential step to assess the function of the LV. Training deep neural networks to automate such measurements is challenging because the gold standard clinical labels are noisy due to inherent observer variability. Also, the labels are only available for at most two time instances in the cine series, end-diastole (ED) and end-systole (ES). In this paper, we first present a multi-head U-Net based model to leverage all available annotations of LV Internal Diameter (LVID), Interventricular Septal (IVS), and Left Ventricular Posterior Wall (LVPW) in a multi-task landmark detection setting. The first head detects the inner landmarks of LV, or LVID (supervised on ED and ES frames), and the second head detects the outer landmarks of the LV (supervised only on ED frame). Secondly, we propose differential learning to further improve the model's performance using an auxiliary task that is semantically relevant to the LVID measurement, but is more robust to inherent observer variability in the labels. This is done through comparing estimated Ejection Fraction (EF) based on the representations of a pair of input cines, by integrating the multi-head model as a Siamese network with an EF comparator network. We evaluate our proposed model on two independent datasets: 1) a large cart-based dataset, consisting of 28,577 echo cines obtained from 23,755 patients, where we demonstrate state-of-the-art performance compared to prior work; 2) 51 echo cines obtained from 23 heart-failure patients using a point-of-care ultrasound (POCUS) system. Imaging heart-failure patients is conventionally considered clinically more challenging. Our approach can be extended to tasks in which the labels are not sufficiently reliable for direct regression, yet their comparison as an auxiliary task is possible.
更多查看译文
关键词
Echo landmark detection,Noisy labels,Sparse labels,Differential learning,Weakly supervised learning
AI 理解论文
溯源树
样例
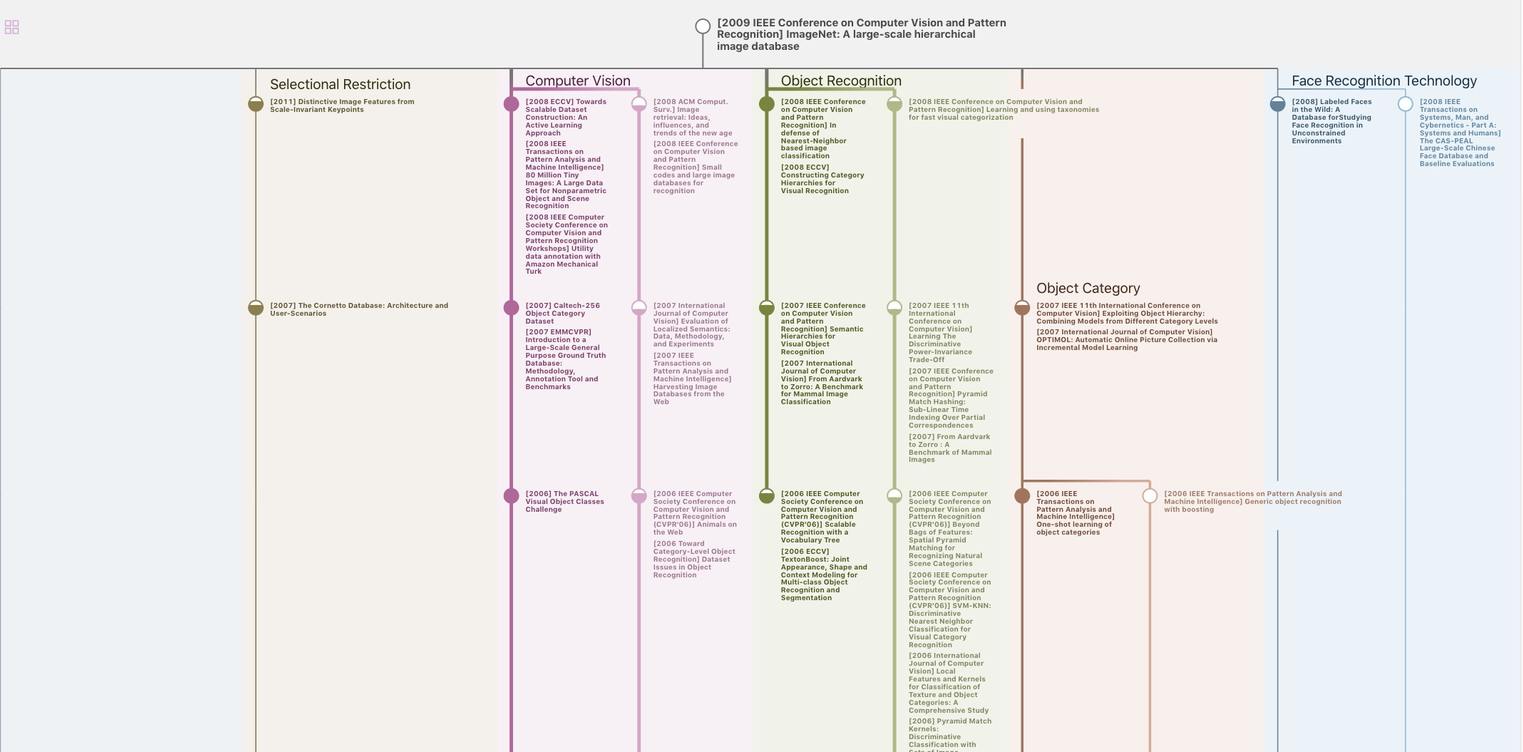
生成溯源树,研究论文发展脉络
Chat Paper
正在生成论文摘要