Vulnerability Detection for Smart Contract via Backward Bayesian Active Learning
APPLIED CRYPTOGRAPHY AND NETWORK SECURITY WORKSHOPS, ACNS 2022(2022)
摘要
Smart contract is a piece of program code running on the blockchain, which aims to realize trusted transactions without third parties. In recent years, smart contract vulnerabilities emerge one after another, resulting in huge economic losses. Machine learning technology is widely used in smart contract vulnerability detection. It is common that model training in machine learning often requires a large amount of labeled data while the unlabeled data in the current field is very rich and acquiring labels is extremely difficult. As a result, it takes a lot of manpower and time to label a vulnerability, and it is challenging to perform effective smart contract vulnerability detection. To tackle this problem, we propose BwdBAL, a novel framework for smart contract vulnerability detection that combines Bayesian Active Learning (BAL) and a backward noise removal method. We use BAL to remove the impact of model uncertainty on uncertainty sampling in active learning. During the backward process, we clean up the noise in the labeled dataset to reduce the negative influence on the classification model. We evaluate BwdBAL on 8 vulnerabilities about 4929 smart contracts with four performance indicators. The experimental results show that BwdBAL outperforms two baseline methods: conventional machine learning-enabled classification method and one-way active learning method.
更多查看译文
关键词
Smart contract, Vulnerability detection, Active learning, Uncertainty measure, Backward learning
AI 理解论文
溯源树
样例
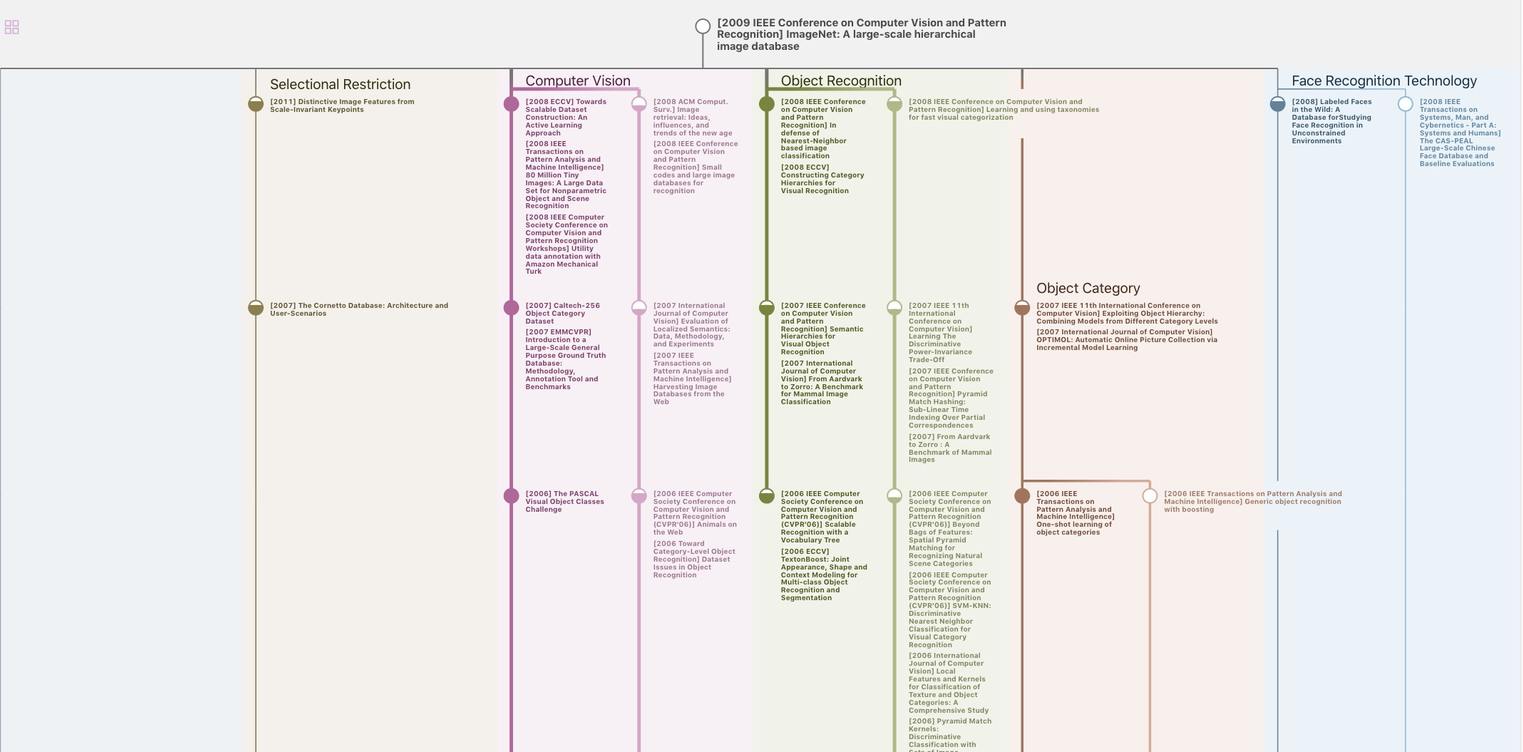
生成溯源树,研究论文发展脉络
Chat Paper
正在生成论文摘要