Wavelet Guided 3D Deep Model to Improve Dental Microfracture Detection
APPLICATIONS OF MEDICAL ARTIFICIAL INTELLIGENCE, AMAI 2022(2022)
摘要
Epidemiological studies indicate that microfractures (cracks) are the third most common cause of tooth loss in industrialized countries. An undetected crack will continue to progress, often with significant pain, until the tooth is lost. Previous attempts to utilize cone beam computed tomography (CBCT) for detecting cracks in teeth had very limited success. We propose a model that detects cracked teeth in high resolution (hr) CBCT scans by combining signal enhancement with a deep CNN-based crack detection model. We perform experiments on a dataset of 45 ex-vivo human teeth with 31 cracked and 14 controls. We demonstrate that a model that combines classical wavelet-based features with a deep 3D CNN model can improve fractured tooth detection accuracy in both micro-Computed Tomography (ground truth) and hr-CBCT scans. The CNN model is trained to predict a probability map showing the most likely fractured regions. Based on this fracture probability map we detect the presence of fracture and are able to differentiate a fractured tooth from a control tooth. We compare these results to a 2D CNN-based approach and we show that our approach provides superior detection results. We also show that the proposed solution is able to outperform oral and maxillofacial radiologists in detecting fractures from the hr-CBCT scans. Early detection of cracks will lead to the design of more appropriate treatments and longer tooth retention.
更多查看译文
关键词
Isotropic wavelets,Cracked teeth,Deep learning
AI 理解论文
溯源树
样例
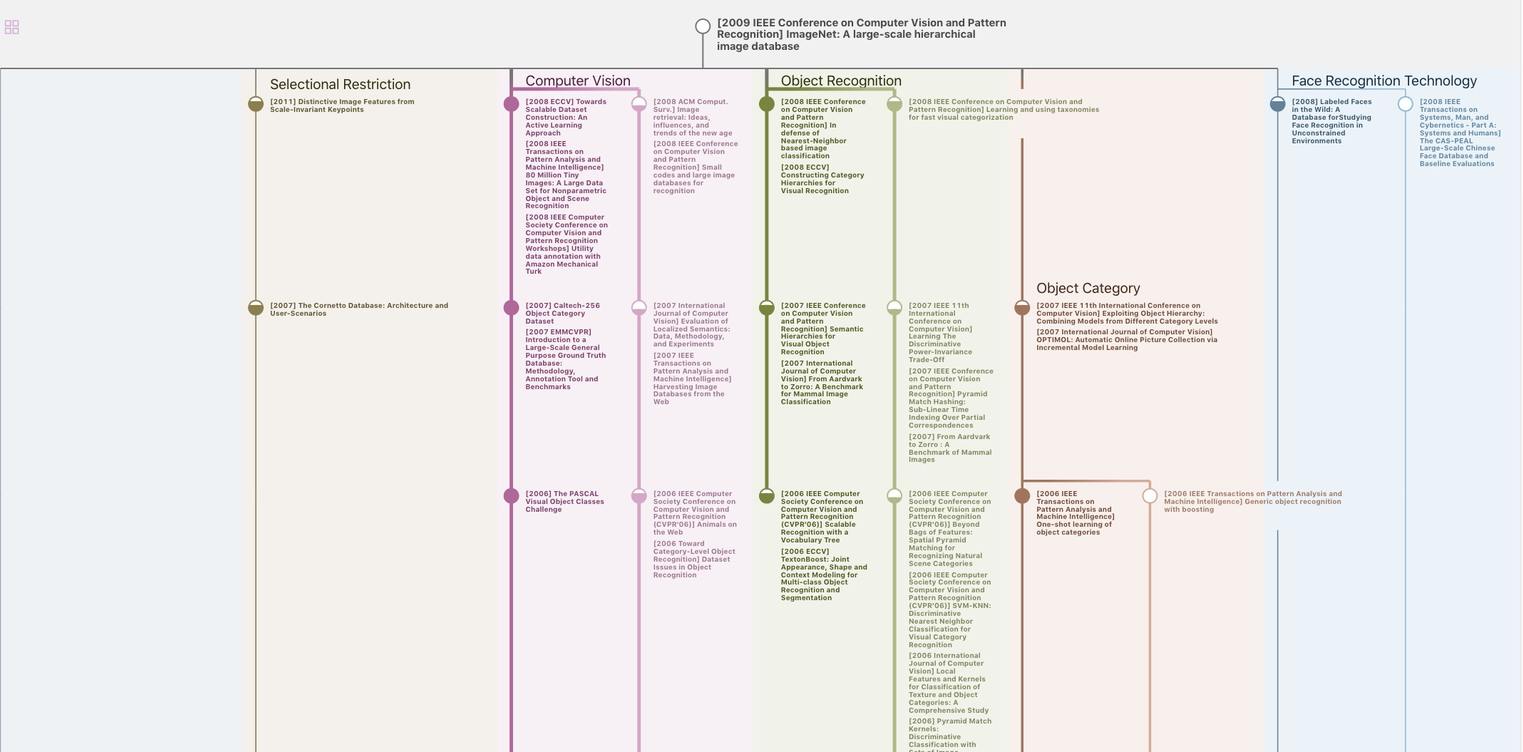
生成溯源树,研究论文发展脉络
Chat Paper
正在生成论文摘要