CDAIL-BIAS MEASURER: A Model Ensemble Approach for Dialogue Social Bias Measurement
NATURAL LANGUAGE PROCESSING AND CHINESE COMPUTING, NLPCC 2022, PT II(2022)
摘要
Dialogue systems based on neural networks trained on large-scale corpora have a variety of practical applications today. However, using uncensored training corpora may have risks, such as potential social bias issues. Meanwhile, manually reviewing these training corpora for social bias content is costly. So, it is necessary to design a recognition model that automatically detects social bias in dialogue systems. NLPCC 2022 Shared Task 7 - Fine-Grain Dialogue Social Bias Measurement, aims to measure social bias in dialogue systems and provides a well-annotated Chinese social bias dialogue dataset - CDAIL-BIAS DATASET. Based on CDAIL-BIAS DATASET, this paper proposes a powerful classifier, CDAIL-BIAS MEASURER. Specifically, we adopt a model ensemble approach, which combines five different pre-trained language models, and uses adversarial training and regularization strategy to enhance the robustness of the model. Finally, labels are obtained by using a novel method - a label-based weighted voting method. The result shows that the classifier has a macro F1 score of 0.580 for social bias measurement in dialogue systems. And our result ranks the third, demonstrating the effectiveness and superiority of our model.
更多查看译文
关键词
Model ensemble, Weighted voting, Pre-trained language model
AI 理解论文
溯源树
样例
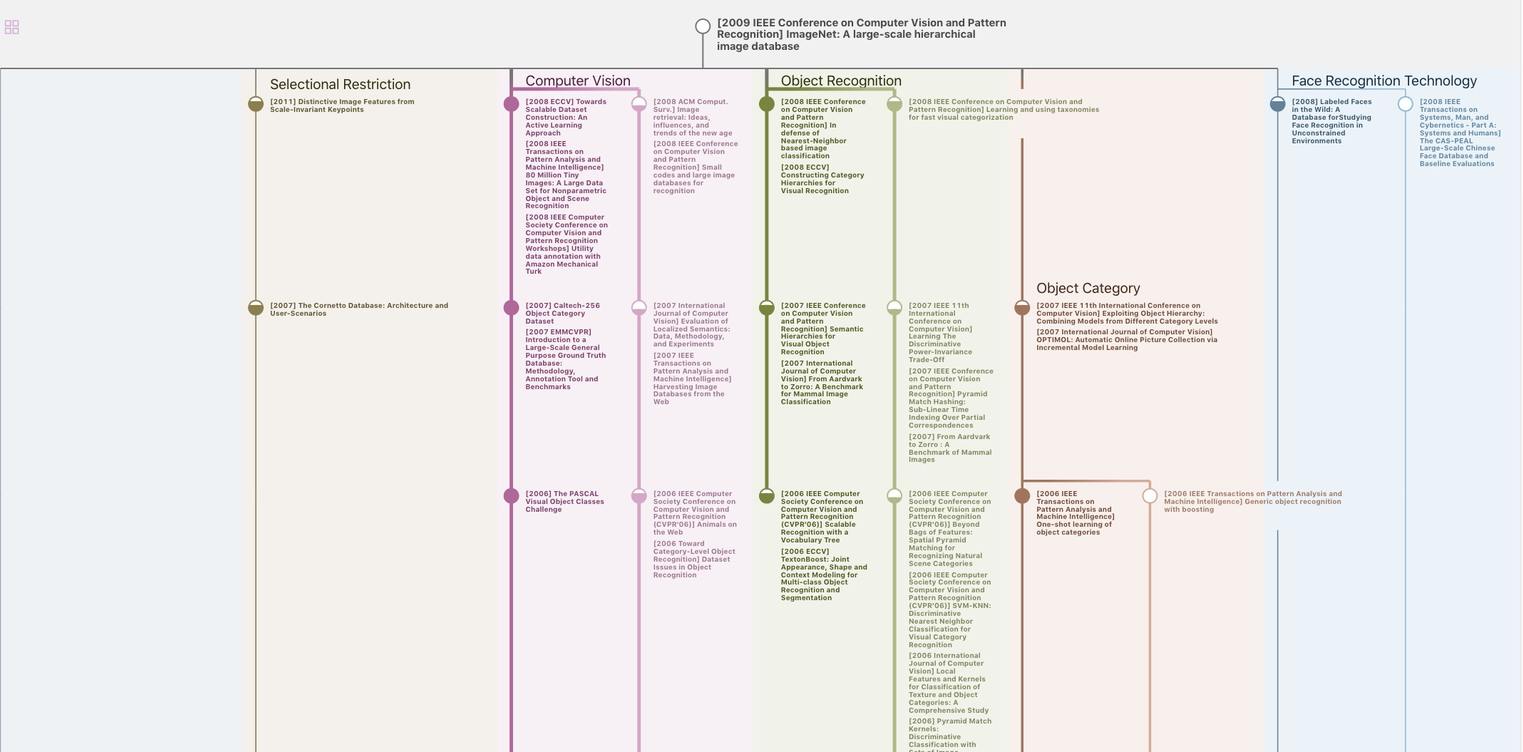
生成溯源树,研究论文发展脉络
Chat Paper
正在生成论文摘要