Comparison of Different Supervised and Self-supervised Learning Techniques in Skin Disease Classification
IMAGE ANALYSIS AND PROCESSING, ICIAP 2022, PT I(2022)
摘要
For years now, The International Skin Imaging Collaboration has been providing datasets of dermoscopic images. Several studies show that dermoscopy provides improved diagnostic accuracy, in comparison to standard photography. Excellent results have been obtained that even exceed human performance. In this paper we broke the state of the art for the dataset provided for the ISIC 2019 challenge. In this work were compared the performance of various convolutional networks, various data augmentations, and various cost functions and optimizers. Results obtained using transfer learning from IMAGENET were compared with the performance obtained using BYOL (bootstrap your own latent), a self-supervised technique. Moreover, it has been demonstrated that self-supervised learning techniques can be used in this field improving the performance of the network compared to training from scratch. We were obtained a balanced multiclass accuracy (BCA) of 87% in the test and validation dataset and with a top-2 accuracy of 97%.
更多查看译文
关键词
Skin disease classification, CNN, Self-supervised learning
AI 理解论文
溯源树
样例
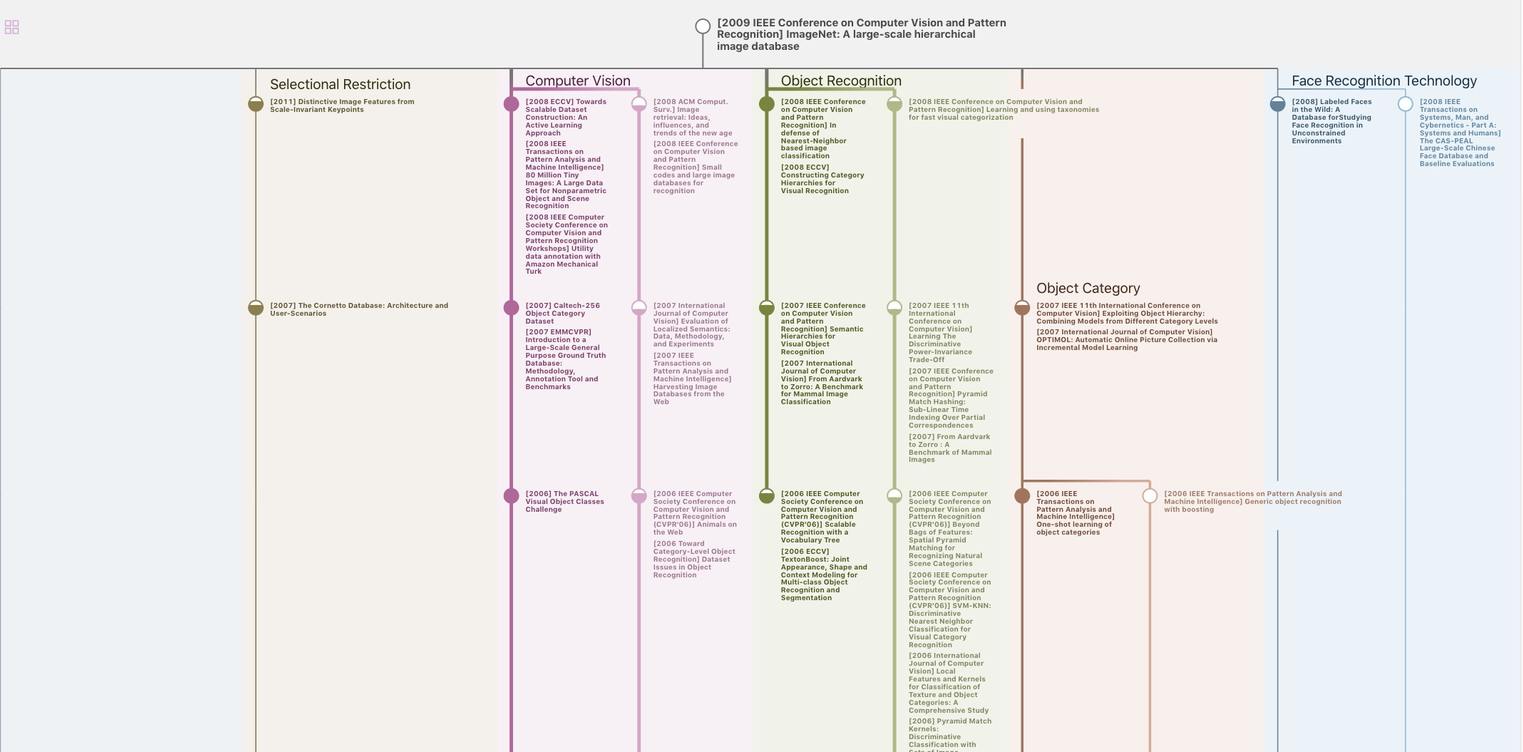
生成溯源树,研究论文发展脉络
Chat Paper
正在生成论文摘要