Investigating the Effect of Using Synthetic and Semi-synthetic Images for Historical Document Font Classification
DOCUMENT ANALYSIS SYSTEMS, DAS 2022(2022)
摘要
This paper studies the effect of using various data augmentation by synthetization approaches on historical image data, particularly for font classification. Historical document image datasets often lack the appropriate size to train and evaluate deep learning models, motivating data augmentation and synthetic document generation techniques for creating additional data. This work explores the effect of various semi-synthetic and synthetic historical document images, some of which appear as recent trends and others not published yet, on a font classification task. We use 10K patch samples as baseline dataset, derived from the dataset of Early Printed Books with Multiple Font Groups, and increase its size using DocCreator software and Generative Adversarial Networks (GAN). Furthermore, we fine-tune different pre-trained Convolutional Neural Network (CNN) classifiers as a baseline using the original dataset and then compare the performance with the additional semi-synthetic and synthetic images. We further evaluate the performance using additional real samples from the original dataset in the training process. DocCreator, and the additional real samples improve the performance giving the best results. Finally, for the best-performing architecture, we explore different sizes of training sets and examine how the gradual addition of data affects the performance.
更多查看译文
关键词
Historical document images, Synthetic image generation, Font classification, Convolutional neural networks, Generative Adversarial Networks, Document Image Analysis, Performance evaluation
AI 理解论文
溯源树
样例
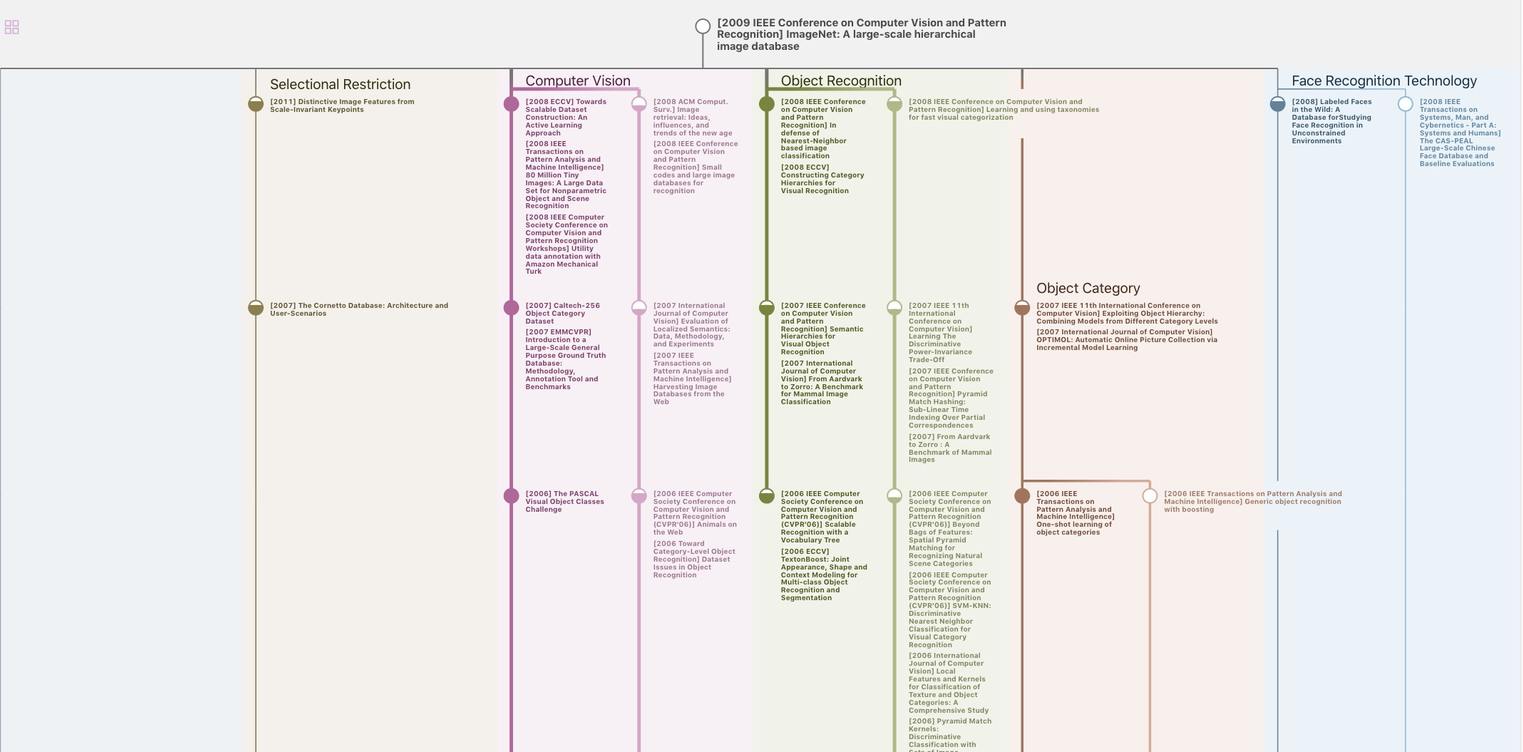
生成溯源树,研究论文发展脉络
Chat Paper
正在生成论文摘要