Cluster Centers Provide Good First Labels for Object Detection
IMAGE ANALYSIS AND PROCESSING, ICIAP 2022, PT I(2022)
摘要
Learning object detection models with a few labels, is possible due to ingenious few-shot techniques, and due to clever selection of images to be labeled. Few-shot techniques work with as few as 1 to 10 randomized labels per object class. We are curious if performance of randomized label selection can be improved by selecting 1 to 10 labels per object class in a non-random manner. Several active learning techniques have been proposed to select object labels, but all started with a minimum of several tens of labels. We explore an effective and simple label selection strategy, for the case of 1 to 10 labels per object class. First, the full unlabeled dataset is clustered into N clusters, where N is the desired number of labels. Clustering is based on k-means on embedding vectors from a state-of-the-art pretrained image classification model (SimCLR v2). The image closest to the center is selected to be labeled. It is effective: on Pascal VOC we validate that it improves over randomized selection over 25%, with large improvements especially when having 1 label per object class. We have several benefits to report on this simple strategy: it is easy to implement, it is effective, and it is relevant in practice where one often starts with a dataset without any labels.
更多查看译文
关键词
Label selection, Few labels, Object detection, Clustering
AI 理解论文
溯源树
样例
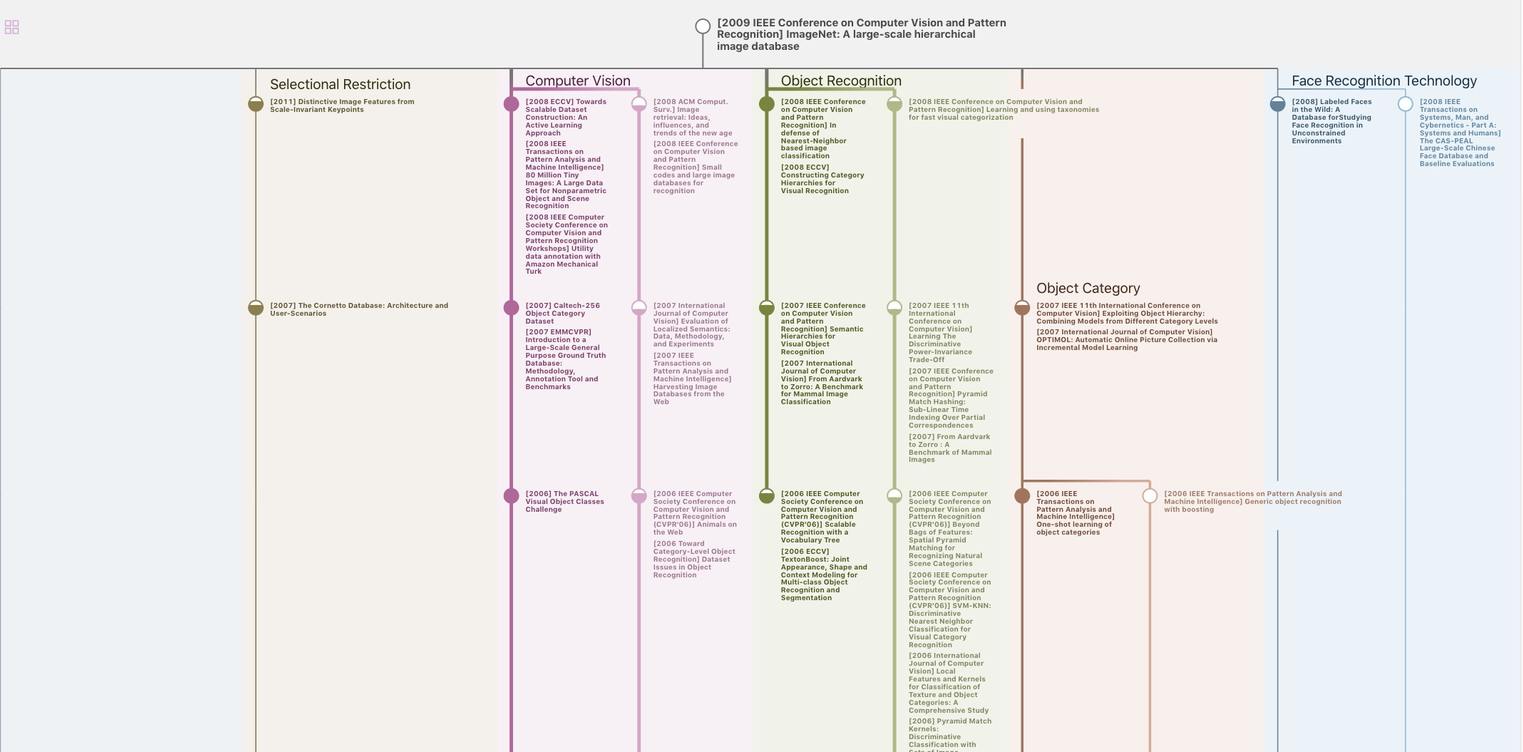
生成溯源树,研究论文发展脉络
Chat Paper
正在生成论文摘要