Spatial-Temporal Autoencoder with Attention Network for Video Compression
IMAGE ANALYSIS AND PROCESSING, ICIAP 2022, PT III(2022)
摘要
Deep learning-based approaches are now state of the art in numerous tasks, including video compression, and are having a revolutionary influence in video processing. Recently, learned video compression methods exhibit a fast development trend with promising results. In this paper, taking advantage of the powerful non-linear representation ability of neural networks, we replace each standard component of video compression with a neural network. We propose a spatial-temporal video compression network (STVC) using the spatial-temporal priors with an attention module (STPA). On the one hand, joint spatial-temporal priors are used for generating latent representations and reconstructing compressed outputs because efficient temporal and spatial information representation plays a crucial role in video coding. On the other hand, we also added an efficient and effective Attention module such that the model pays more effort on restoring the artifact-rich areas. Moreover, we formalize the rate-distortion optimization into a single loss function, in which the network learns to leverage the Spatial-temporal redundancy presented in the frames and decreases the bit rate while maintaining visual quality in the decoded frames. The experiment results show that our approach delivers the state-of-the-art learning video compression performance in terms of MS-S SIM and PSNR.
更多查看译文
关键词
Video compression, Deep learning, Auto-encoder, Rate-distortion optimization, Attention mechanism
AI 理解论文
溯源树
样例
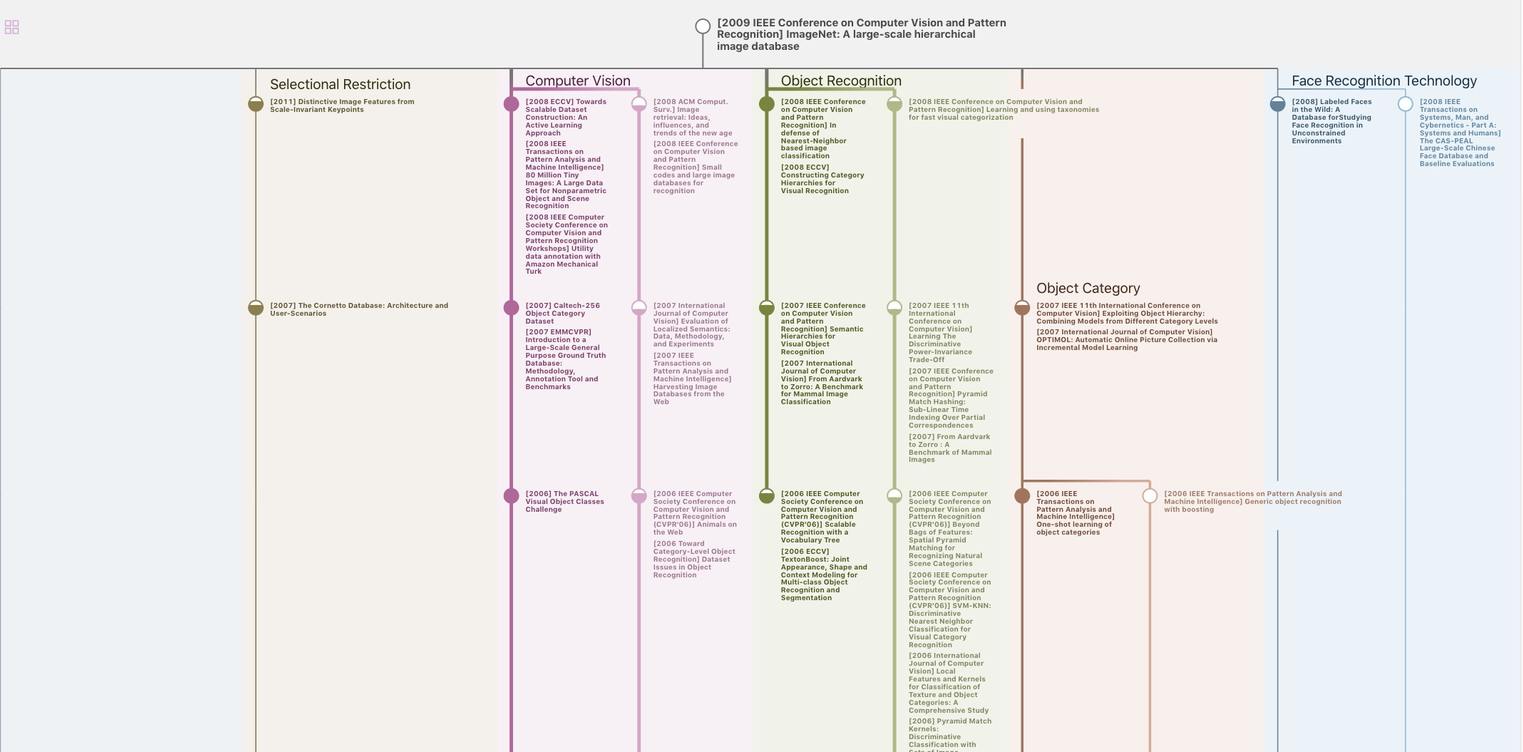
生成溯源树,研究论文发展脉络
Chat Paper
正在生成论文摘要