Learning Visual Landmarks for Localization with Minimal Supervision
IMAGE ANALYSIS AND PROCESSING, ICIAP 2022, PT I(2022)
摘要
Camera localization is one of the fundamental requirements for vision-based mobile robots, self-driving cars, and augmented reality applications. In this context, learning spatial representations relative to unique regions in a scene with Slow Feature Analysis (SFA) has demonstrated large-scale localization. However, it relies on hand-labeled data to train a CNN for recognizing unique regions. We propose a new approach that uses pre-trained CNN-detectable objects as anchors to label and learn new landmark objects or regions in a scene using minimal supervision. The method bootstraps the landmark learning process and removes the need to manually label large amounts of data. The anchor objects are only required to learn the new landmarks and become obsolete for the unsupervised mapping and localization phases. We present localization results with the learned landmarks in simulated and real-world outdoor environments and compare the results to SFA on complete images and PoseNet. The landmark-based localization shows similar or better accuracy than the baseline methods in challenging scenarios. Our results further suggest that the approach scales well and achieves even higher localization accuracy by increasing the number of learned landmarks without increasing the number of anchors.
更多查看译文
关键词
Localization, Mapping, Landmarks, Service robots
AI 理解论文
溯源树
样例
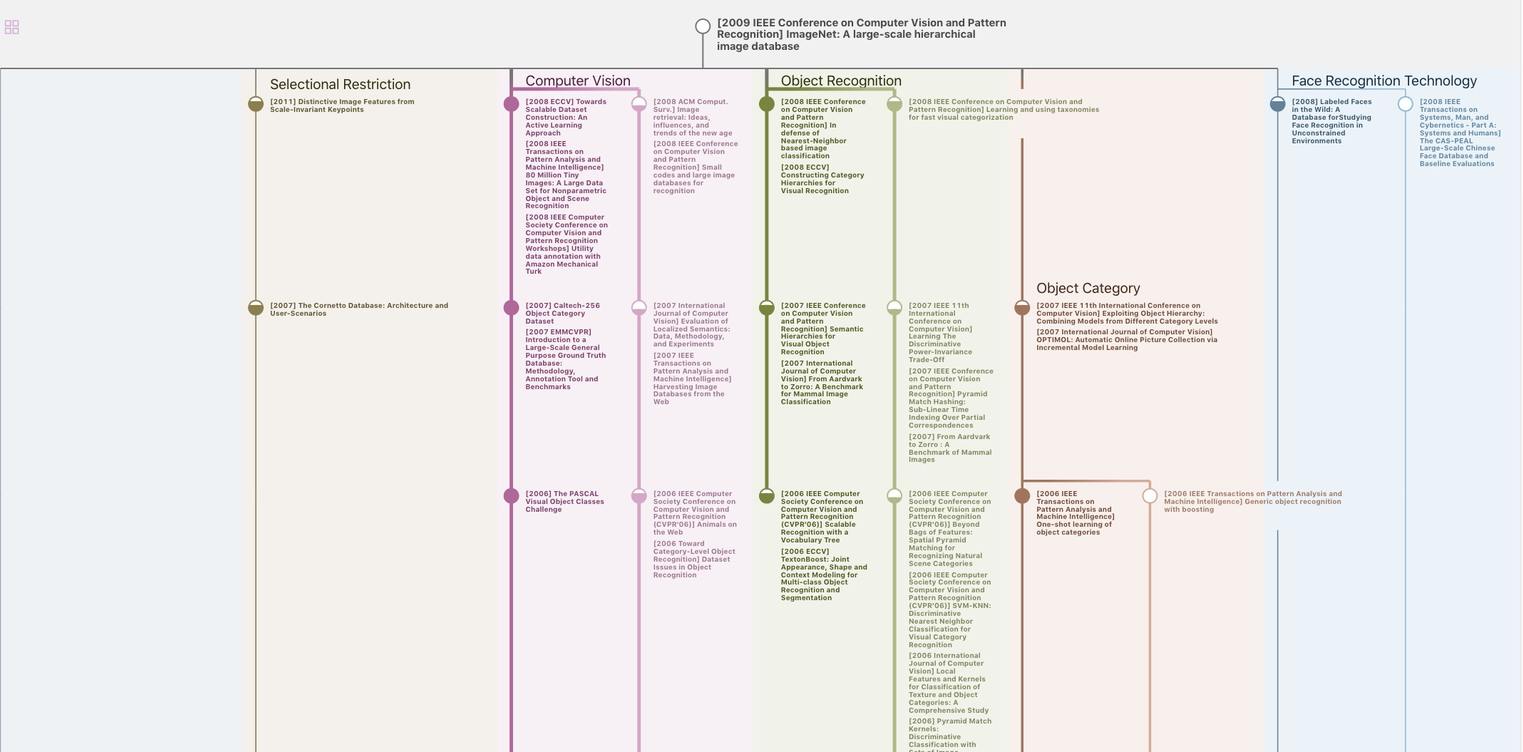
生成溯源树,研究论文发展脉络
Chat Paper
正在生成论文摘要