An Optimization-Based Decomposition Heuristic for the Microaggregation Problem
PRIVACY IN STATISTICAL DATABASES, PSD 2022(2022)
摘要
Given a set of points, the microaggregation problem aims to find a clustering with a minimum sum of squared errors (SSE), where the cardinality of each cluster is greater than or equal to k. Points in the cluster are replaced by the cluster centroid, thus satisfying k-anonymity. Microaggregation is considered one of the most effective techniques for numerical microdata protection. Traditionally, non-optimal solutions to the microaggregation problem are obtained by heuristic approaches. Recently, the authors of this paper presented a mixed integer linear optimization (MILO) approach based on column generation for computing tight solutions and lower bounds to the microaggregation problem. However, MILO can be computationally expensive for large datasets. In this work we present a new heuristic that combines three blocks: (1) a decomposition of the dataset into subsets, (2) the MILO column generation algorithm applied to each dataset in order to obtain a valid microaggregation, and (3) a local search improvement algorithm to get the final clustering. Preliminary computational results show that this approach was able to provide (and even improve upon) some of the best solutions (i.e., of smallest SSE) reported in the literature for the Tarragona and Census datasets, and k is an element of{3, 5, 10}.
更多查看译文
关键词
Statistical disclosure control, Microdata, Microaggregation problem, Mixed integer linear optimization, Column generation, Local search, Heuristics
AI 理解论文
溯源树
样例
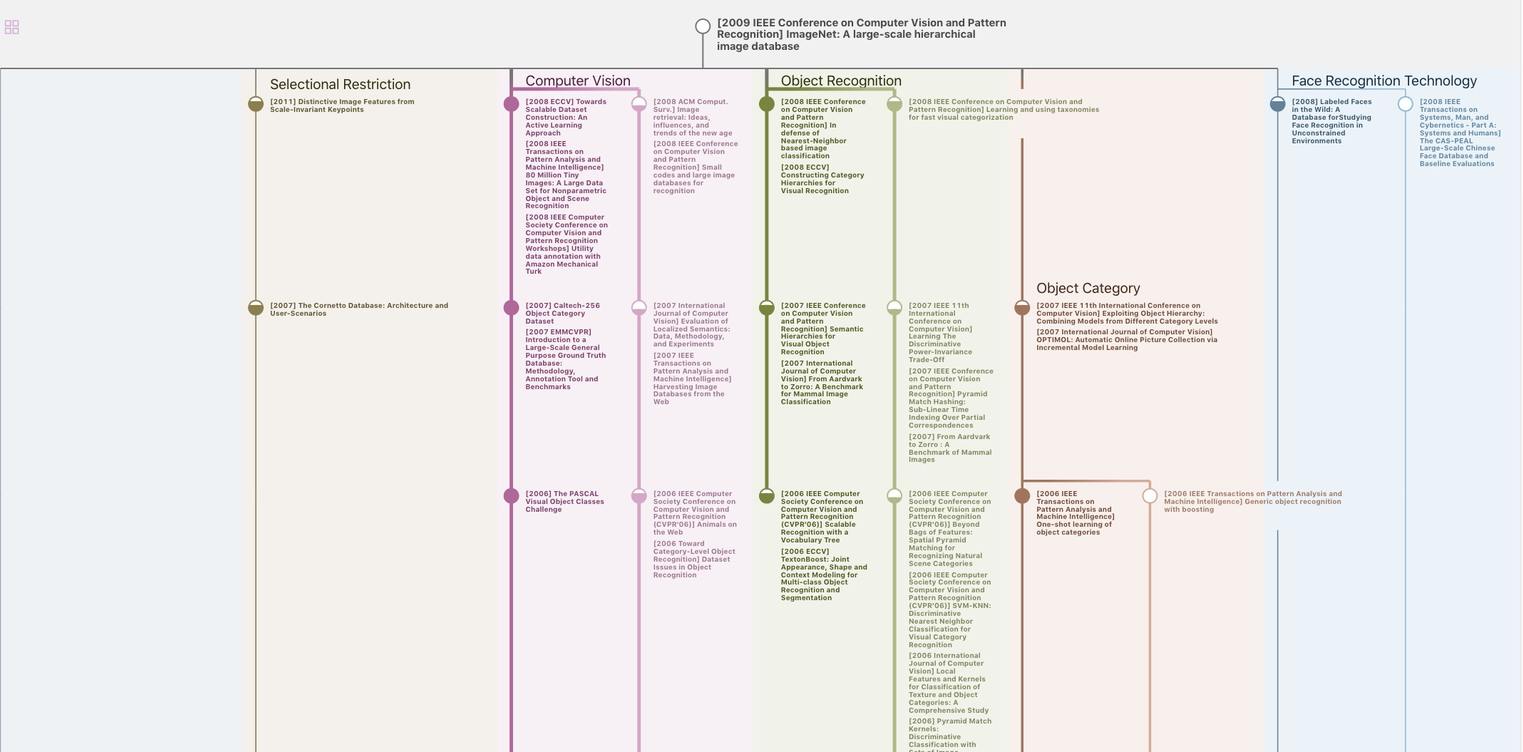
生成溯源树,研究论文发展脉络
Chat Paper
正在生成论文摘要