Arbitrary Voice Conversion via Adversarial Learning and Cycle Consistency Loss
INTELLIGENT COMPUTING THEORIES AND APPLICATION, ICIC 2022, PT II(2022)
摘要
Recently, Non-parallel voice conversion (VC) has attracted the attention of many researchers in the field of speech. However, such model suffers from the limitation that how to improve the generalization of the model to extract the speaker information from unseen speaker. In this paper, we proposed a novel zero-shot VC approach which performs VC only rely on the speaker belong to training set. To achieve this VC method, we disentangle the speaker and content representations with instance normalization, and then use the adversarial learning to encourage model to produce more similar converted result. The experiment results demonstrate that our approach can achieve arbitrary voice conversion without any supervision.
更多查看译文
关键词
Voice conversion, Zero-shot learning, Adversarial learning, Disentangle representations
AI 理解论文
溯源树
样例
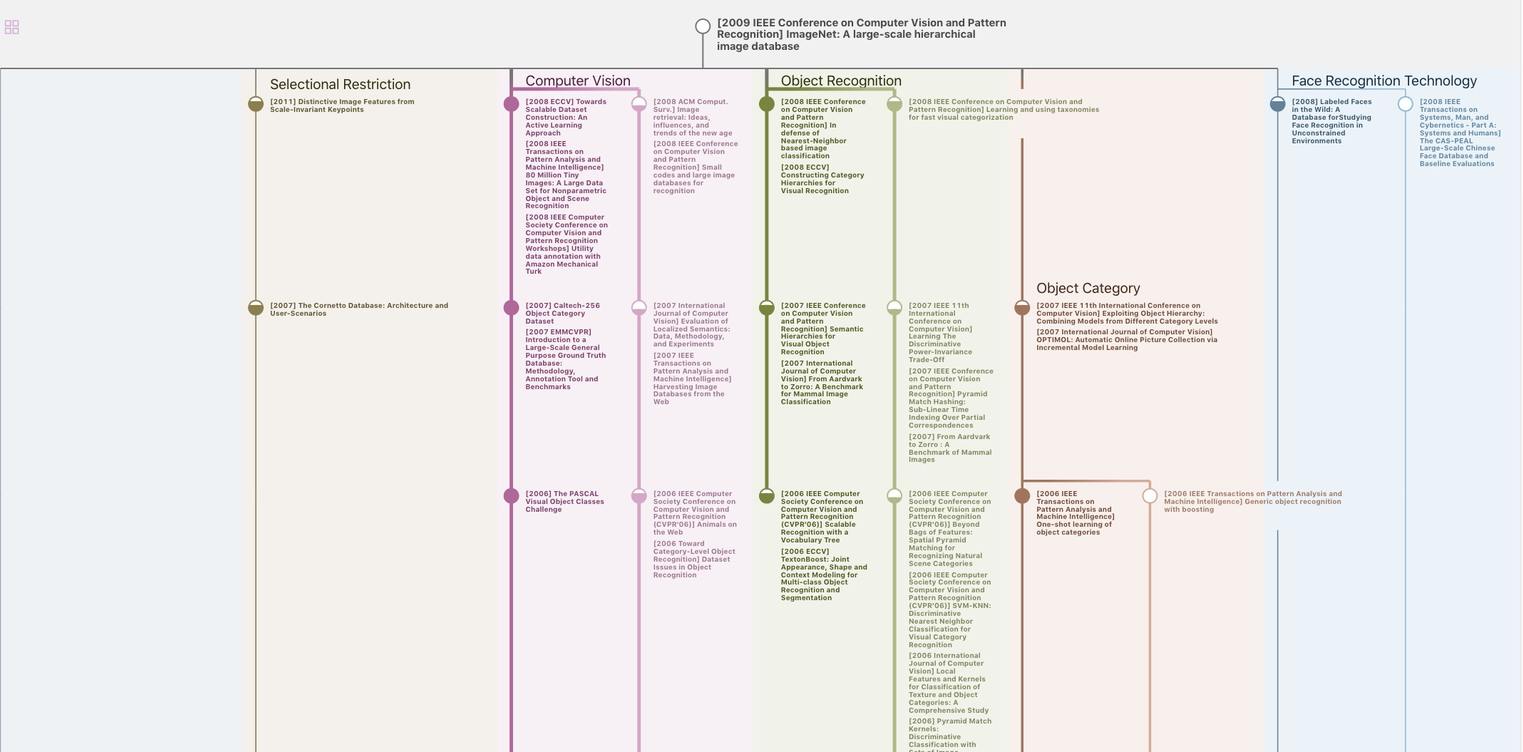
生成溯源树,研究论文发展脉络
Chat Paper
正在生成论文摘要