A Two-Phased Approach to Energy Consumption Prediction for Fused Filament Fabrication of CFR-PEEK
ADVANCES IN PRODUCTION MANAGEMENT SYSTEMS: SMART MANUFACTURING AND LOGISTICS SYSTEMS: TURNING IDEAS INTO ACTION, APMS 2022, PT I(2022)
摘要
With increasing attention on fused filament fabrication (FFF) applications for high-performance polymers, understanding the energy characteristics of FFF is critical to pursue a sustainable additive manufacturing system. In this regard, this study proposes a two-phased energy prediction approach to FFF. A full factorial design of experiments for FFF is planned to collect data for build time, filament volume, and energy consumption by varying major process parameters (i.e., layer thickness, infill density, and printing speed). Carbon fiber reinforced polyetherether-ketone (CFR-PEEK), which is an emerging high-performance polymer for advanced engineering applications, is used for a material of the experiments. In the first phase, two machine learning methods (i.e., decision tree and random forest) are employed to estimate the material addition rate (MAR) of FFF, which indicates the amount of deposited material volume per unit time. Then, a statistical regression model of energy consumption on the estimated MAR is built to predict the energy consumption of FFF for CFR-PEEK. The results show that the decision tree model has better prediction performance to predict the MAR of FFF than the random forest model. In addition, the energy consumption model through the predicted MAR shows that an increase in the MAR reduces energy consumption. The two-phased prediction approach based on the MAR provides better performance than the direct energy prediction from process parameters through a machine learning technique. The findings of this study provide a basis to characterize energy dynamics in FFF for CFR-PEEK.
更多查看译文
关键词
Fused filament fabrication, CFR-PEEK, Material addition rate, Energy consumption, Machine learning
AI 理解论文
溯源树
样例
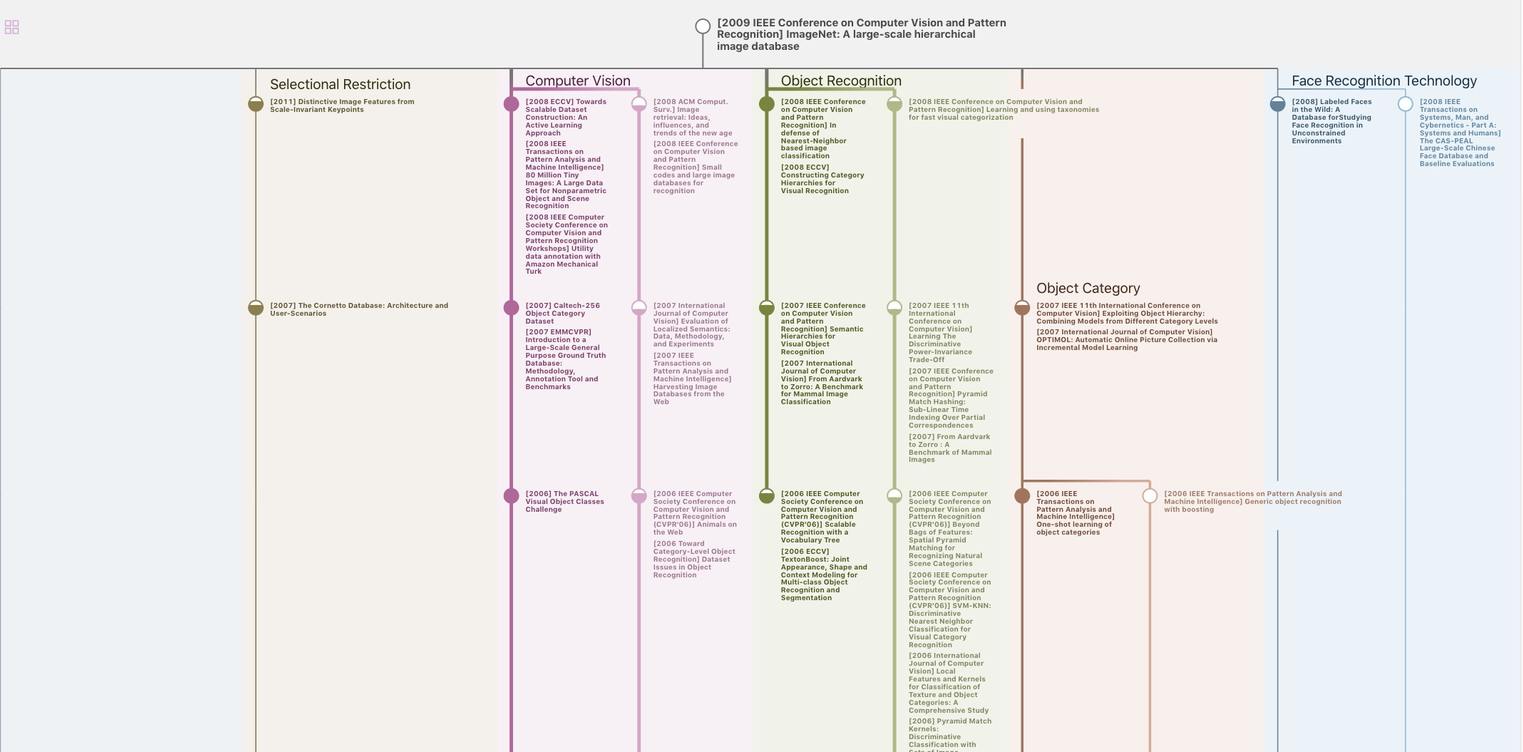
生成溯源树,研究论文发展脉络
Chat Paper
正在生成论文摘要