Criterion constrained Bayesian hierarchical models
TEST(2022)
摘要
The goal of this article is to improve the predictive performance of a Bayesian hierarchical statistical model by incorporating a criterion typically used for model selection. In this article, we view the problem of prediction of a latent real-valued mean as a model selection problem, where the candidate models are from an uncountable infinite set (i.e., the parameter space of the mean represents the candidate set of models). Specifically, we select a subset of our Bayesian hierarchical statistical model’s parameter space with high predictive performance (as measured by a criterion). Explicitly, we truncate the joint support of the data and the parameter space of a given Bayesian hierarchical model to only include small values of the covariance penalized error (CPE) criterion. The CPE is a general expression that contains several information criteria as special cases. Simulation results show that as long as the truncated set does not have near-zero probability, we tend to obtain a lower squared error than Bayesian model averaging. Additional theoretical results are provided asthe foundation for these observations. We apply our approach to a dataset consisting of American Community Survey period estimates to illustrate that this perspective can lead to improvements in a single model.
更多查看译文
关键词
Bayesian hierarchical model, Markov chain Monte Carlo, Posterior predictive p value, Information theory, Gaussian Processes
AI 理解论文
溯源树
样例
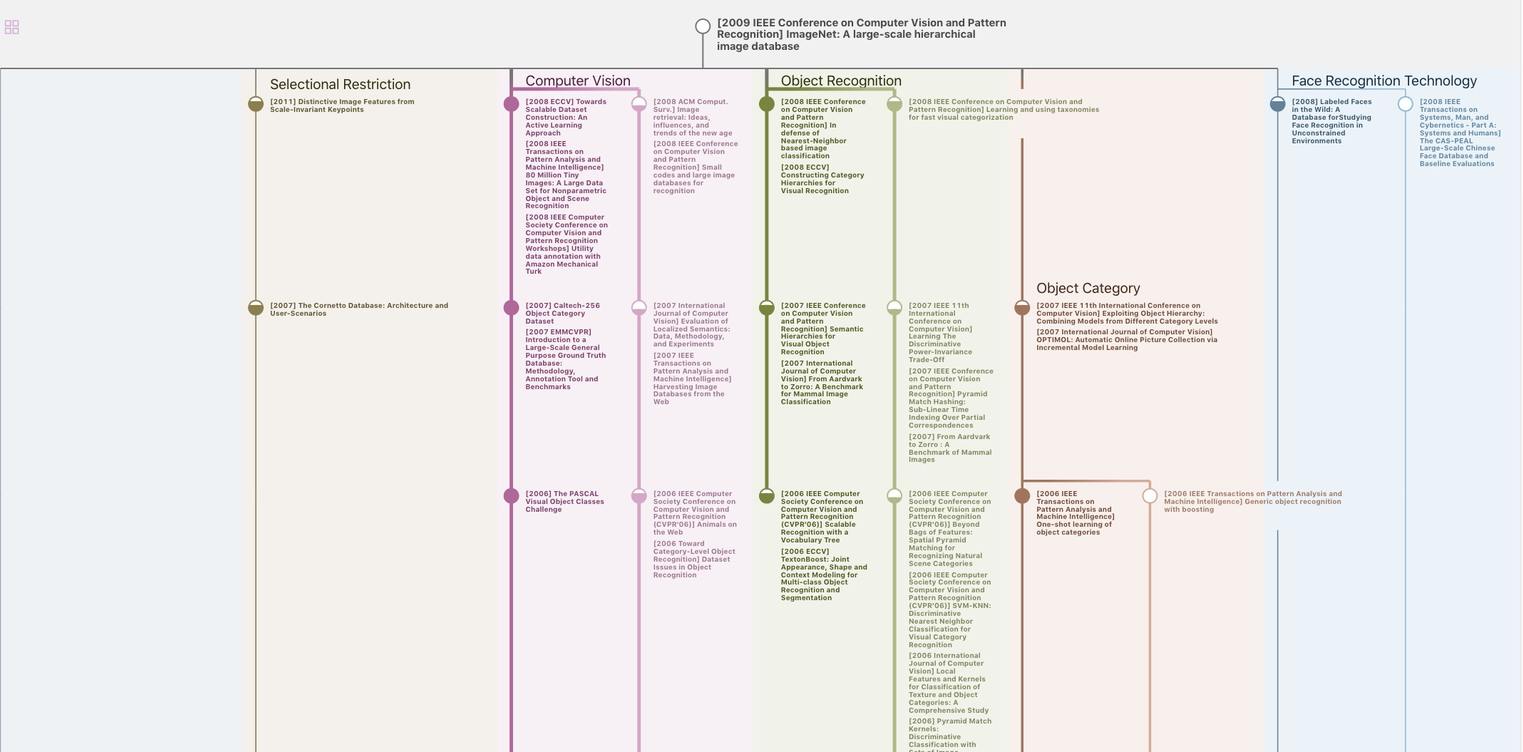
生成溯源树,研究论文发展脉络
Chat Paper
正在生成论文摘要