SVM Ensembles on a Budget
ARTIFICIAL NEURAL NETWORKS AND MACHINE LEARNING - ICANN 2022, PT IV(2022)
摘要
This paper presents a model to train an ensemble of SVMs that achieves better generalization performance at a lower computational training cost than a single SVM. The idea of the proposed model is, instead of training a single SVM on the whole dataset, to train a diverse set of simpler SVMs. Specifically, the proposed algorithm creates B subensembles of T SVMs using a different set of hyper-parameters in each subensemble. Then, in order to gain more diversity, the T SVMs of each of the subsensembles are trained on a different 1/T disjoint fraction of the training set. The paper presents an extensive analysis of the computational training complexity of the algorithm. The experiments show that for any given computational budget, the presented method obtains a better generalization performance than a single SVM.
更多查看译文
关键词
SVM, Ensembles of classifiers, Computational complexity
AI 理解论文
溯源树
样例
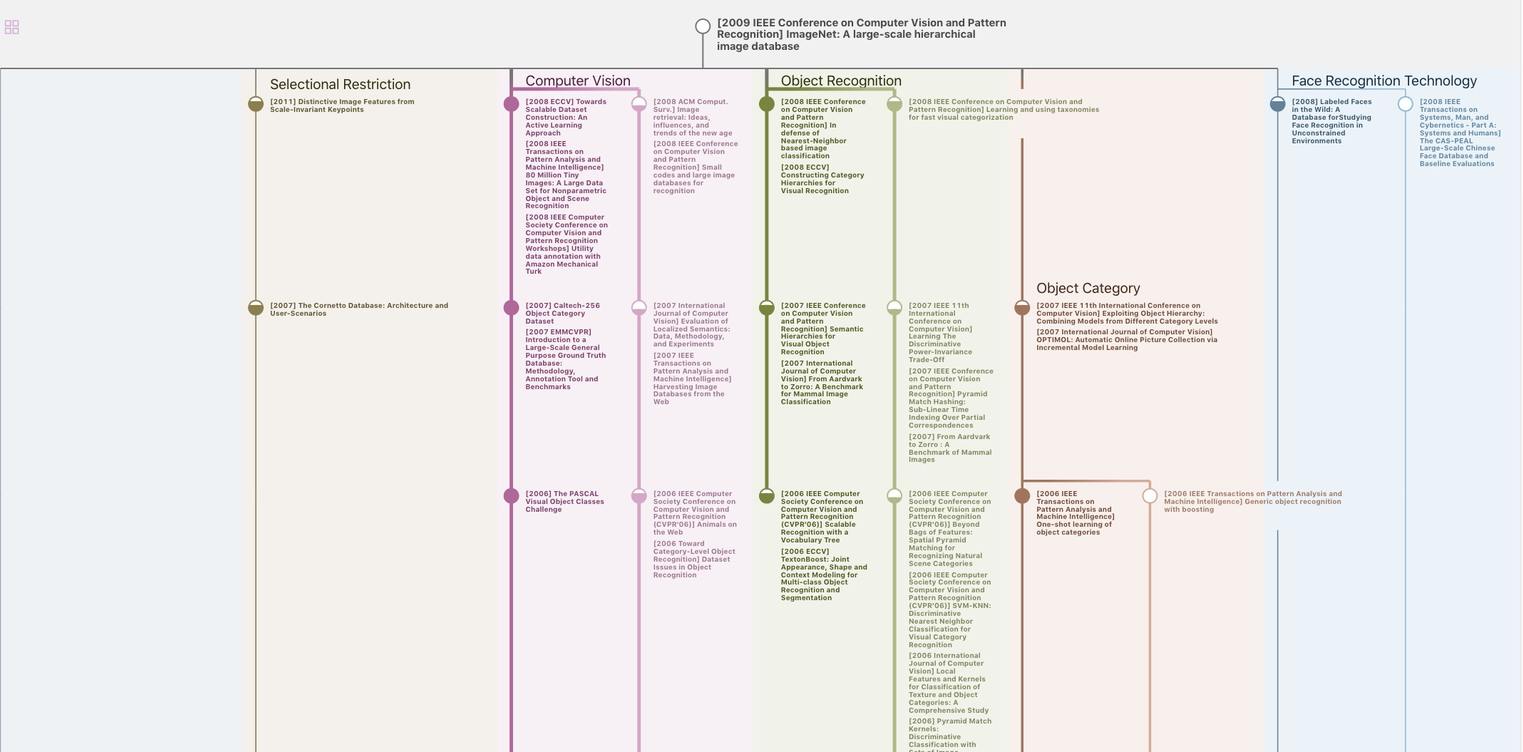
生成溯源树,研究论文发展脉络
Chat Paper
正在生成论文摘要