Effective ML-Block and Weighted IoU Loss for Object Detection
ARTIFICIAL NEURAL NETWORKS AND MACHINE LEARNING - ICANN 2022, PT IV(2022)
摘要
In computer vision tasks, better performance of the transformer model is due to self-attention mechanism and learning of global information. However, it also largely increases parameters and calculations. In this paper, we propose the following problems. (1) How to build a lighter module that integrates CNN and Transformer? We propose the ML-block module in this paper. Especially, for one thing, reducing the number of channels after the convolution module; for another, spatial attention is introduced after the ML-block input layer to reduce the loss caused by information fusion. (2) Small object detection problem in one-stage object detector. We propose weighted IoU loss in this paper. According to the object size, it adaptively weighs the IoU loss to improve the performance of the detector. We adopt YOLOX-s as the baseline through sufficient experiments on PASCAL VOC data sets and COCOmini data sets to demonstrate the effectiveness of our methods, and AP increases by 1.1% and 3.3%.
更多查看译文
关键词
Object detection, ML-block, Transformer, Weighted IoU loss
AI 理解论文
溯源树
样例
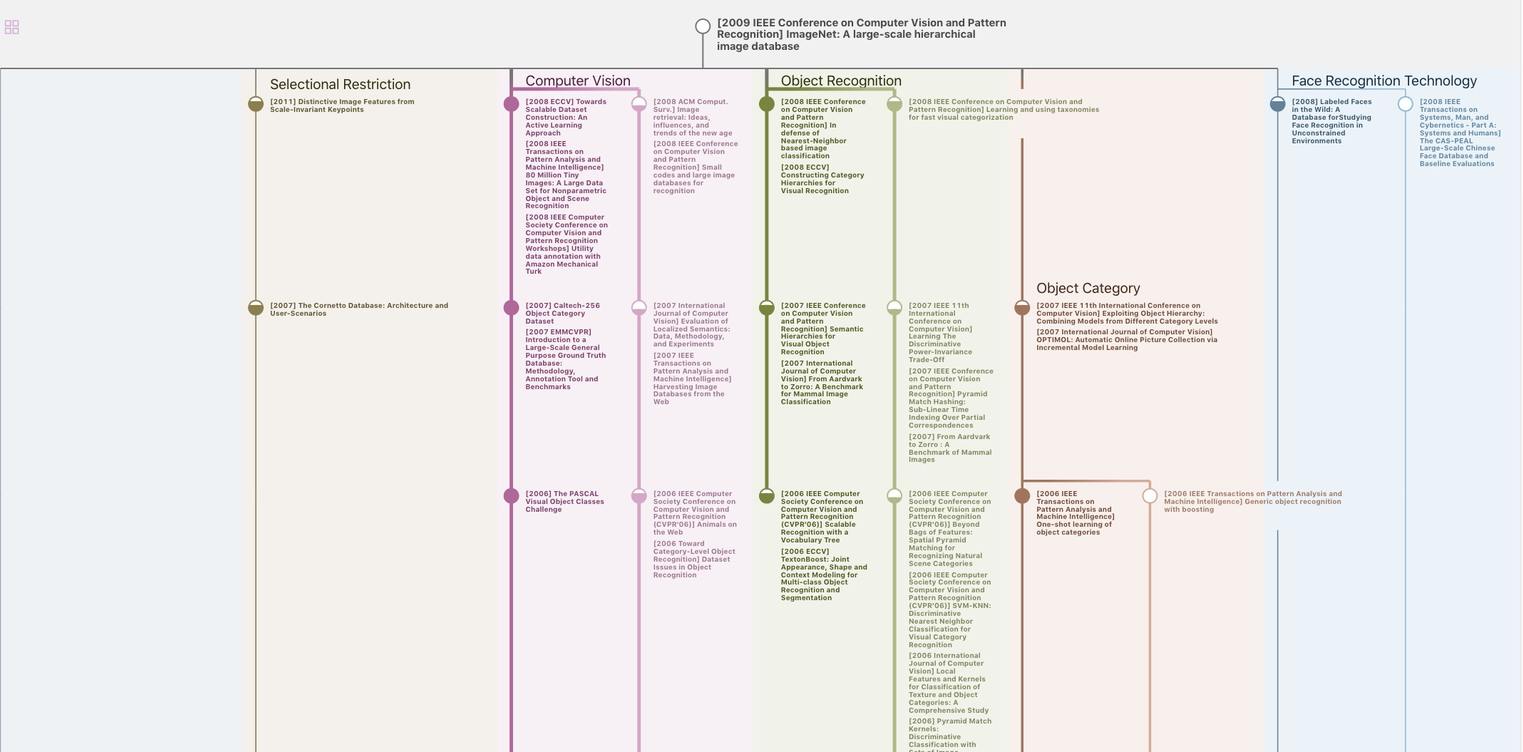
生成溯源树,研究论文发展脉络
Chat Paper
正在生成论文摘要