Internal Fault Diagnosis of Riveted Aluminum Alloy Plates Based on Gaussian CDBN with Multi-layer Feature Fusion
PROCEEDINGS OF 2022 IEEE INTERNATIONAL CONFERENCE ON MECHATRONICS AND AUTOMATION (IEEE ICMA 2022)(2022)
摘要
The application of lightweight material jointing is popular for aircraft and automotive industry due to fuel economy. In order to ensure the quality of the body riveting, it is essential to develop a nondestructive testing (NDT) method to identify the internal riveting defects. The paper presents a diagnosis algorithm for internal riveting faults by improved Gaussian convolutional deep belief network (CDBN). Firstly, the feature extraction method is proposed to process the detection data collected from the eddy current sensor to improve the efficiency of data analysis. Secondly, Gaussian visible units are used to replace the binary visible units of the network model to enhance the feature representation ability. Finally, the multilayer feature fusion method is employed to improve the feature learning ability of each layer of the model. The algorithm is applied to the detection of riveting internal faults. The experimental results reflect that the algorithm can effectively improve the recognition rate of riveting faults compared with the methods based on DBN, standard CDBN and convolutional neural network.
更多查看译文
关键词
Aluminum alloy riveting, Defect diagnosis, Feature extraction, CDBN, Multi-layer feature fusion
AI 理解论文
溯源树
样例
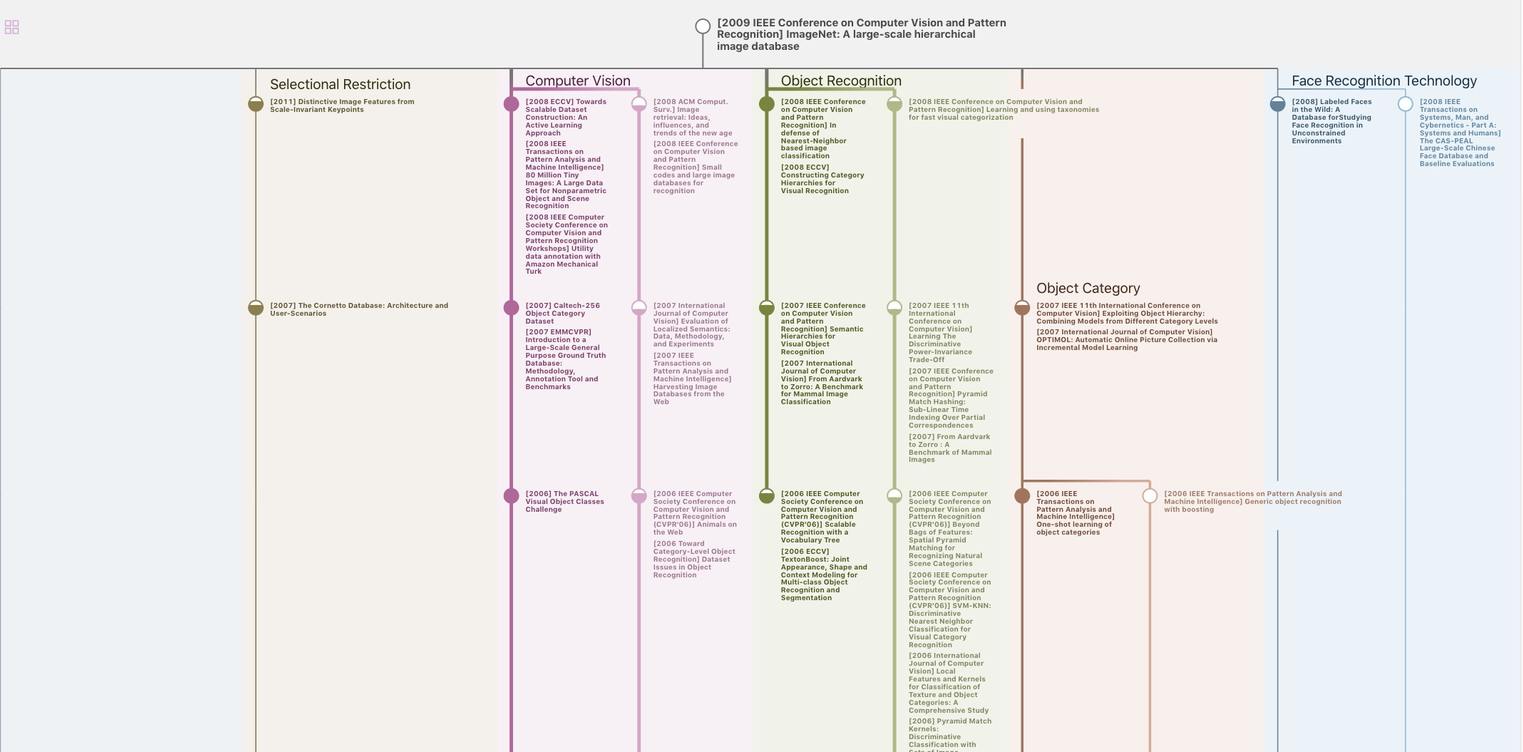
生成溯源树,研究论文发展脉络
Chat Paper
正在生成论文摘要