Outage-Constrained Transceiver Power Loading: A Deep Learning Approach to Robust Massive MIMO Downlink
2022 IEEE International Black Sea Conference on Communications and Networking (BlackSeaCom)(2022)
摘要
In this research, we consider massive multiple-input multiple-output (MIMO) communication systems, where the base station (BS) equipped with a uniform planar array (UPA) serves several multi-antennas users based on imperfect channel state information (CSI). By utilizing the statistical properties of channel estimation error, our purpose is to design beamformers to minimize the total transmitted power, subject to certain quality of service (QoS) outage probability. Inspired by the Chebyshev Inequality, we relax the probabilistic QoS constraints by constructing a function on the channel estimation error, whose mean value is a significant multiple of its standard deviation, where the multiple is determined by the error distribution assumption and required outage probability. Then we develop an iterative algorithm correspondingly, which is able to properly allocate the transmitted power. The standard deviation plays a crucial role in power allocation, yet is time-consuming to be solved by the iterative manner. For this reason, we construct a deep learning (DL)-based framework to obtain the standard deviation directly. Experimental results prove the proposed robust transceiver can improve the outage probability remarkably, while the DL-based framework can distinctly reduce the computational complexity.
更多查看译文
关键词
massive MIMO,robust transceiver,imperfect CSI,deep learning,downlink beamforming,channel uncertainty
AI 理解论文
溯源树
样例
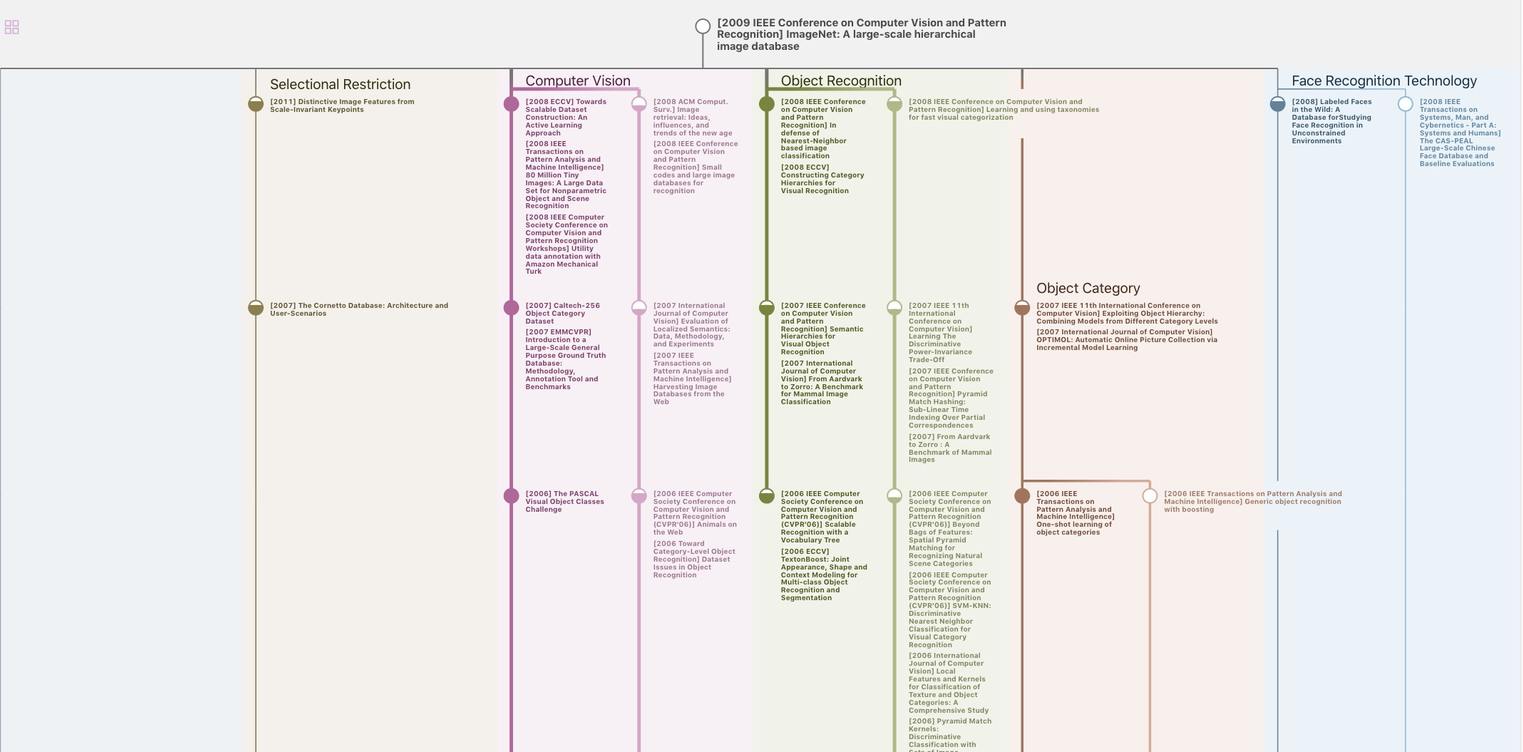
生成溯源树,研究论文发展脉络
Chat Paper
正在生成论文摘要