Cellular Actin Cytoskeleton Morphology Identification for Mechanical Characterization Using Deep Learning
IEEE ACCESS(2022)
摘要
Actin cytoskeleton morphology is able to affect and reflect the cellular mechanical properties. However, due to the lack of efficient approaches to quantifying actin cytoskeleton and mechanical properties, the study of mechanotransduction dynamics is still laborious. In this paper, a model to characterize the cellular actin cytoskeleton morphology was built using the graph to vector embedding technique together with neural network in machine learning (ML). The proposed ML model consists of a skip-gram model followed by a fully connected classifier. The images of NIH/3T3 cells treated with Latrunculin B at different concentrations were taken as the inputs, and the outputs were the actin cytoskeleton morphology labels defined by treatment concentrations (i.e., the actin depolymerization level). The proposed model was also compared to a general convolutional neural network (CNN) and three commonly used transfer learning models (GoogleNet, Xception, and VGG16), the results demonstrated the capabilities of the proposed model in extracting actin cytoskeleton features, avoiding overfitting, and keeping the model generalization.
更多查看译文
关键词
Morphology, Feature extraction, Brightness, Deep learning, Mathematical models, Convolutional neural networks, Data models, Actin cytoskeleton, classification, graph to vector embedding, machine learning, skip-gram model
AI 理解论文
溯源树
样例
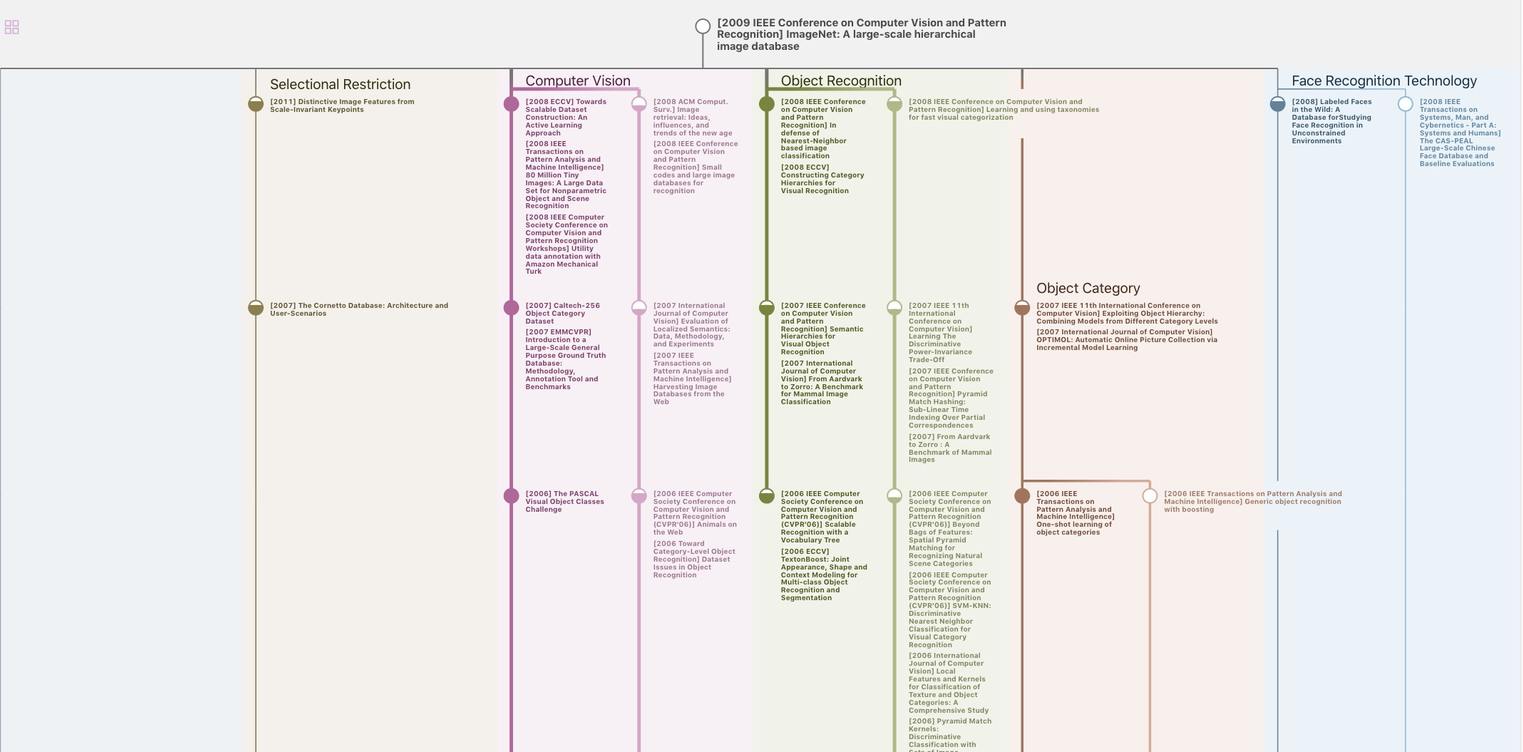
生成溯源树,研究论文发展脉络
Chat Paper
正在生成论文摘要