Sentence Graph Attention for Content-Aware Summarization
APPLIED SCIENCES-BASEL(2022)
摘要
Neural network-based encoder-decoder (ED) models are widely used for abstractive text summarization. While the encoder first reads the source document and embeds salient information, the decoder starts from such encoding to generate the summary word-by-word. However, the drawback of the ED model is that it treats words and sentences equally, without discerning the most relevant ones from the others. Many researchers have investigated this problem and provided different solutions. In this paper, we define a sentence-level attention mechanism based on the well-known PageRank algorithm to find the relevant sentences, then propagate the resulting scores into a second word-level attention layer. We tested the proposed model on the well-known CNN/Dailymail dataset, and found that it was able to generate summaries with a much higher abstractive power than state-of-the-art models, in spite of an unavoidable (but slight) decrease in terms of the Rouge scores.
更多查看译文
关键词
summarization, knowledge graph, neural networks, pagerank, natural language processing
AI 理解论文
溯源树
样例
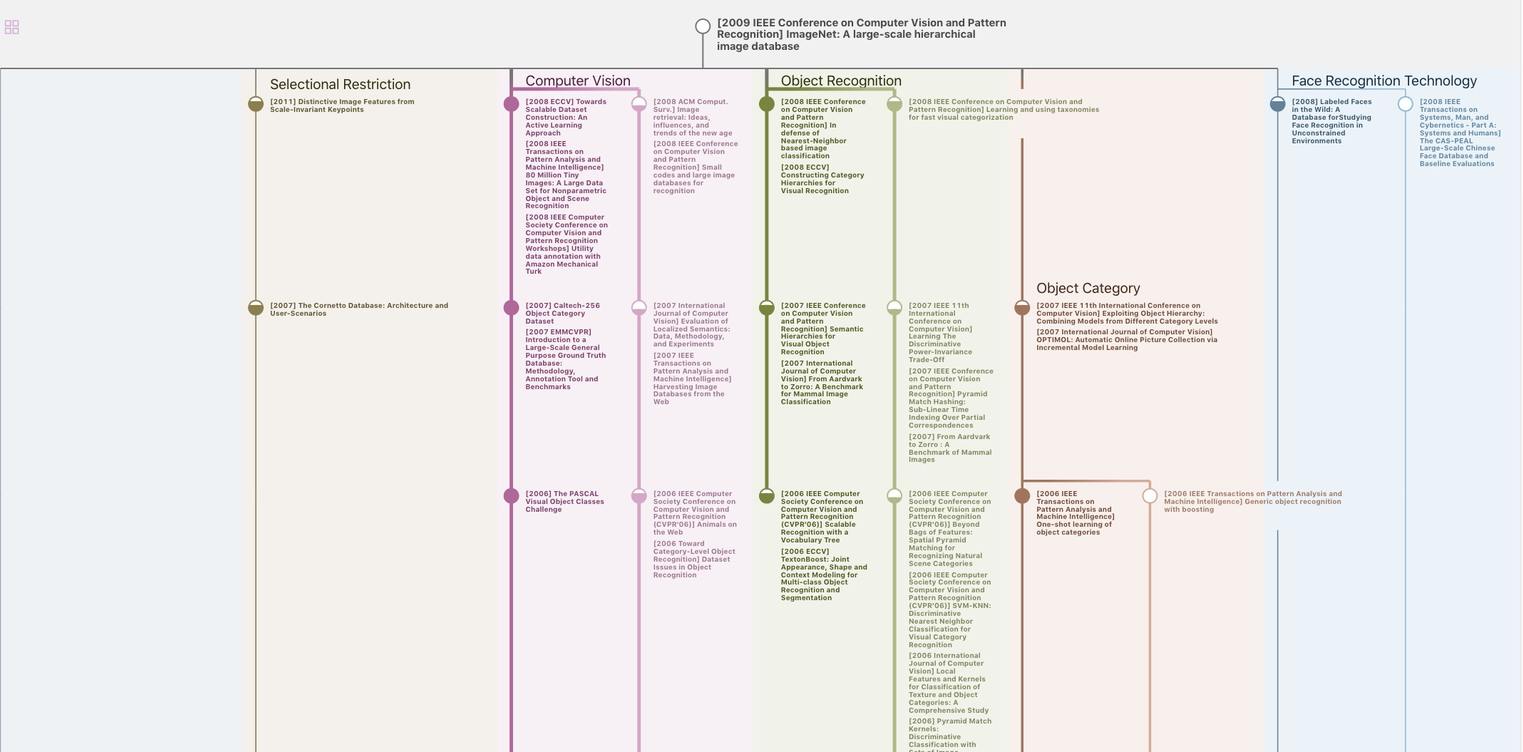
生成溯源树,研究论文发展脉络
Chat Paper
正在生成论文摘要